Device specific SD-OCT Retinal Layer Segmentation using Cycle-Generative Adversarial Networks in patients with AMD
MEDICAL IMAGING 2022: COMPUTER-AIDED DIAGNOSIS(2022)
Abstract
Spectral Domain Optical Coherence Tomography (SD-OCT) is a much-utilized imaging modality in retina clinics as it provides a 3D rendering of retina structure revealing disease-specific changes. Due to the stark difference in intensities and signal to noise ratios between the images captured by two different instruments, a model trained on images from one instrument performs poorly on the images of the other vendor. Our goal was to investigate methods to utilize a segmentation algorithm developed using Heidelberg Spectralis images on images obtained using Zeiss Cirrus images. Methods: Utilizing a dataset containing Heidelberg images and Cirrus images, we address the problem of accurately segmenting images on one domain with an algorithm developed on another domain. In our approach we use unpaired CycleGAN based domain adaptation network to transform the Cirrus volumes to the Spectralis volumes, before using our trained segmentation network. Results: We show that the intensity distribution shifts towards the Spectralis domain when we domain adapt Cirrus images to Spectralis images. Our results show that the segmentation model performs significantly better on the domain translated volumes (Total Retinal Volume Error: -0.41 +/- 0.38mm(3), RPEDC Volume Error: -0.087 +/- 0.24mm(3)) compared to the raw volumes (Total Retinal Volume-Error: -9.44 +/--4.91mm(3), RPEDC Volume Error: -4.47 +/- 6.35mm(3)) from the Cirrus domain and that such domain adaptation approaches are feasible solutions. Conclusions: Both our qualitative and quantitative results show that CycleGAN domain adaptation network can be used as an efficient technique to perform unpaired domain adaptation between SD-OCT images generated from different devices. We show that a 3D segmentation model trained on Spectralis volume performs better on domain adapted Cirrus volumes, compared to raw Cirrus volumes.
MoreTranslated text
Key words
3D-UNet,Domain Adaptation,Device independent feature extraction,retinal layer segmentation,Generative Adversarial Networks (GAN's),cycle Generative Adversarial Networks (Cycle-GAN's),retina,volumetric segmentation
AI Read Science
Must-Reading Tree
Example
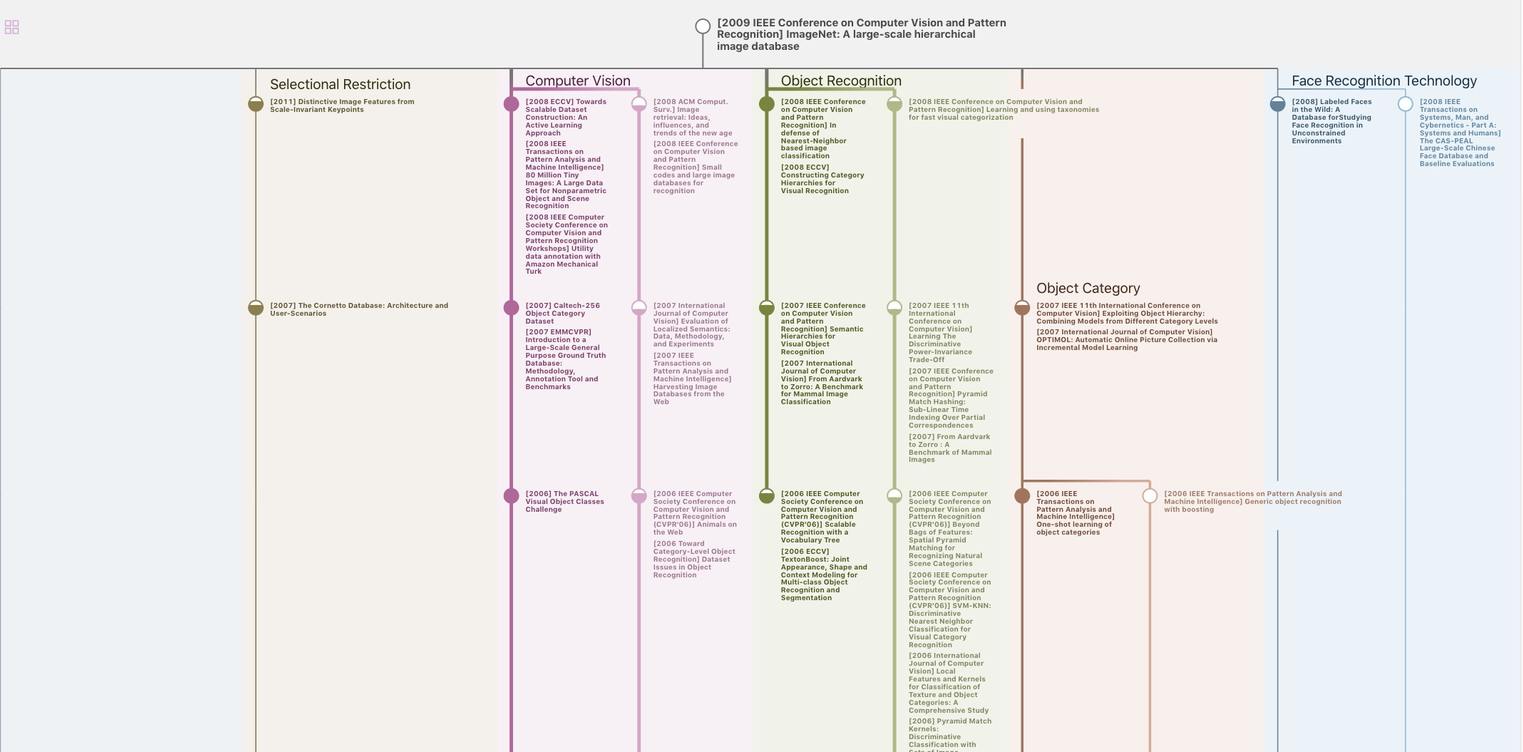
Generate MRT to find the research sequence of this paper
Chat Paper
Summary is being generated by the instructions you defined