Comparing and Interpreting Differently-Designed Random Forests for Next-Day Severe Weather Hazard Prediction
Weather and Forecasting(2022)
摘要
Abstract Recent research has shown that random forests (RFs) can create skillful probabilistic severe weather hazard forecasts from numerical weather prediction (NWP) ensemble data. However, it remains unclear how RFs use NWP data and how predictors should be generated from NWP ensembles. This paper compares two methods for creating RFs for next-day severe weather prediction using simulated forecast data from the convection-allowing High-Resolution Ensemble Forecast System, Version 2.1 (HREFv2.1). The first method uses predictors from individual ensemble members (IM) at the point of prediction, while the second uses ensemble mean (EM) predictors at multiple spatial points. IM and EM RFs are trained with all predictors as well as predictor subsets, and the Python module tree interpreter (TI) is used to assess RF variable importance and the relationships learned by the RFs. Results show that EM RFs have better objective skill compared to similarly-configured IM RFs for all hazards, presumably because EM predictors contain less noise. In both IM and EM RFs, storm variables are found to be most important, followed by index and environment variables. Interestingly, RFs created from storm and index variables tend to produce forecasts with greater or equal skill than those from the all-predictor RFs. TI analysis shows that the RFs emphasize different predictors for different hazards in a way that makes physical sense. Further, TI shows that RFs create calibrated hazard probabilities based on complex, multi-variate relationships that go well beyond thresholding 2-5km updraft helicity.
更多查看译文
关键词
Ensembles, Forecasting, Artificial intelligence, Decision trees, Machine learning, Model interpretation and visualization
AI 理解论文
溯源树
样例
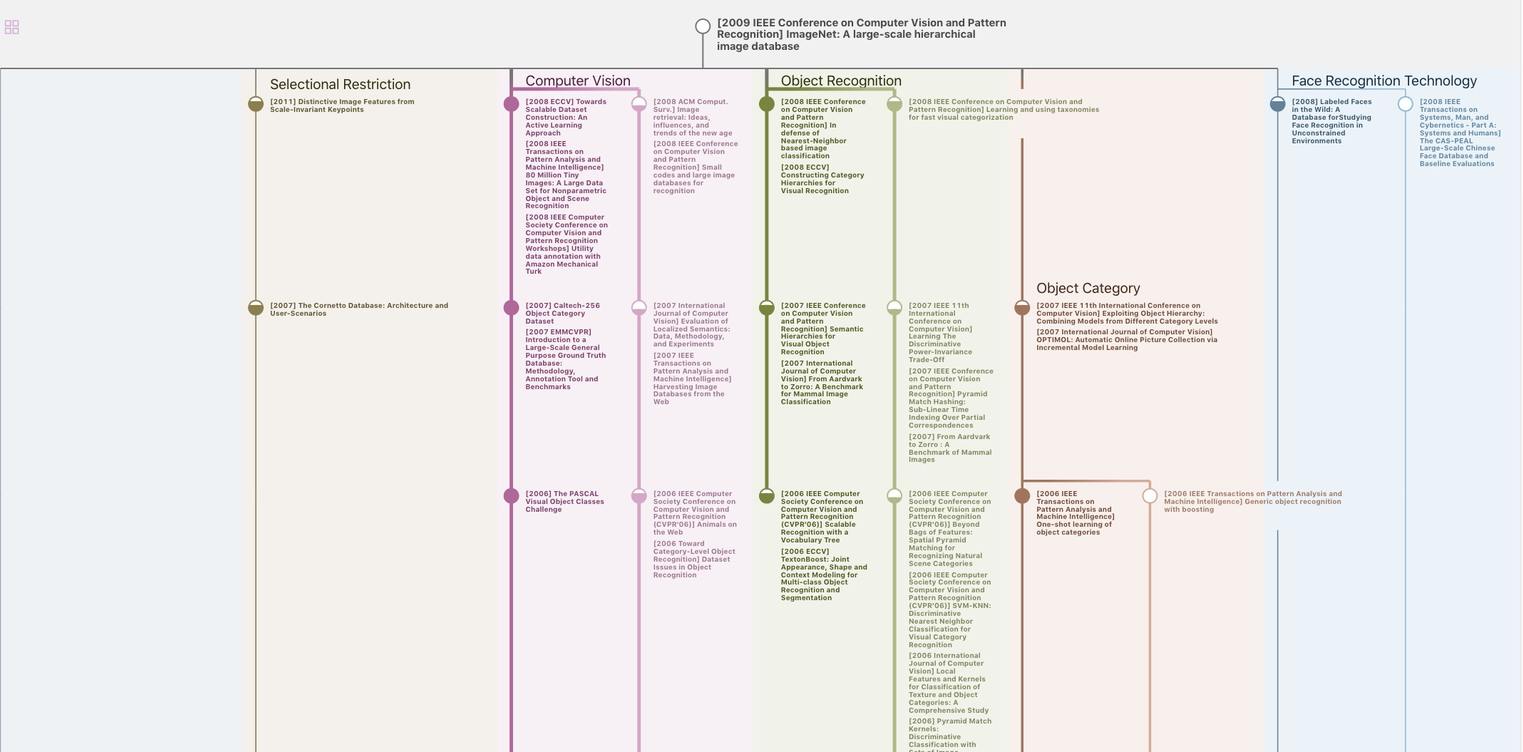
生成溯源树,研究论文发展脉络
Chat Paper
正在生成论文摘要