An Invariant and Highly–Accurate Strategy for Data-Driven Turbulence Modelling
SSRN Electronic Journal(2022)
Abstract
We develop a data–driven Machine Learning (ML) turbulence modeling based on a recently proposed method that significantly enhances the accuracy of the solution of Reynolds–averaged Navier–Stokes (RANS) equations, which were shown to be ill-conditioned. The approach is able to extract from the mean velocity field high fidelity data, the essential information to construct the Reynolds force vector (RFV). This quantity was shown to produce less error propagation, which motivates its use as a target for ML techniques. Invariance is enforced by means of a novel procedure where vectorial and tensorial quantities are represented by an intrinsic local coordinate system associated with the eigenvectors of the rate-of-strain tensor of the injection environment. The proposed hybrid method employs an implicit procedure for the linear part of the RFV. This structure is applied to two geometries analyzed in the literature namely the Square Duct and the Periodic Hill flows, using Direct Numerical Simulation (DNS) data as a learning environment andRANS simulations as an injection environment. A Random–Forest (RF) approach is used for the Machine Learning process. A comparison between the predictions obtained with the present method and other ones employed in the literature illustrates the capability of the strategy. The new hybrid method that presented lower error propagation and better conditioning was also the method with lower error propagation in the predictions by the ML approach for both geometries.
MoreTranslated text
Key words
turbulence,data-driven
AI Read Science
Must-Reading Tree
Example
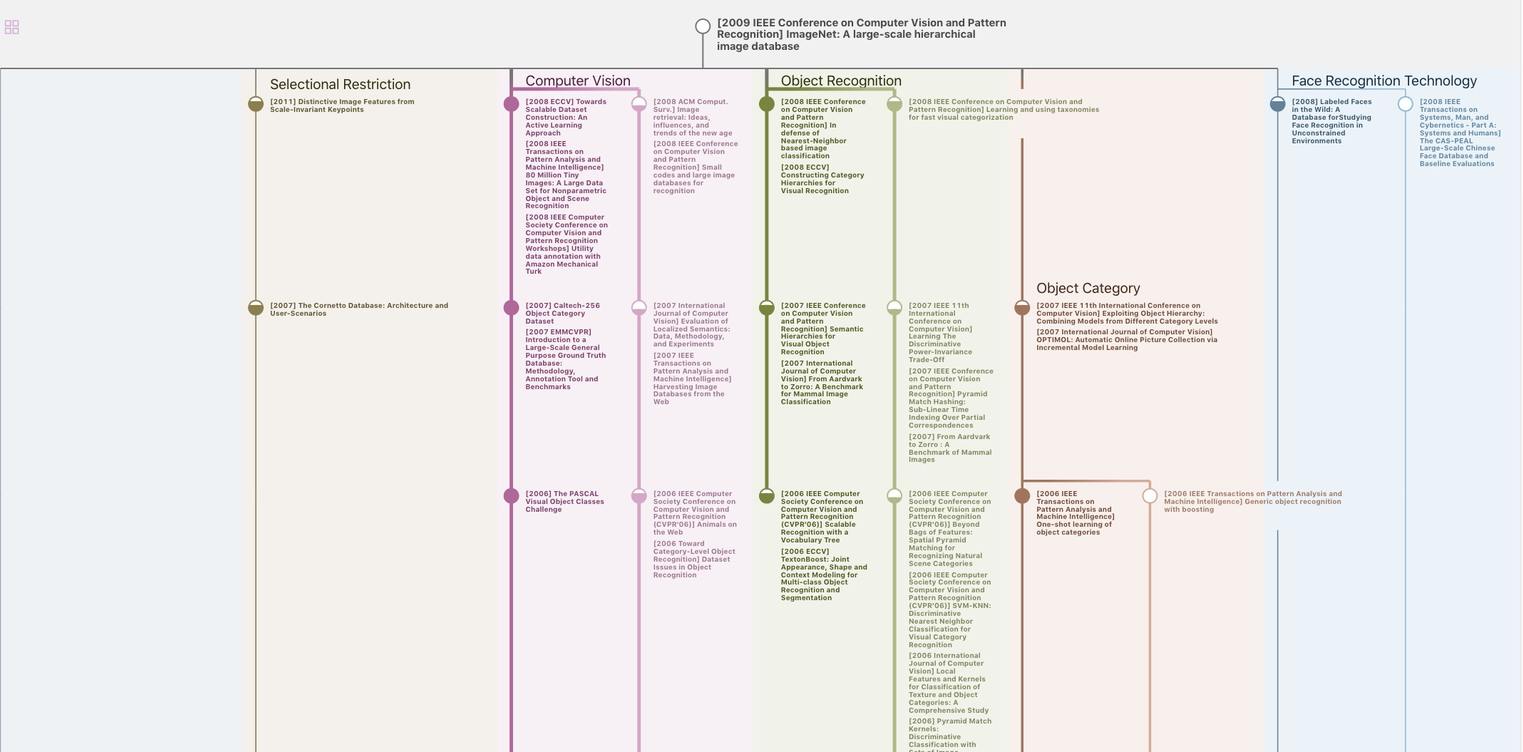
Generate MRT to find the research sequence of this paper
Chat Paper
Summary is being generated by the instructions you defined