Aligning Heterogeneous Optimization Problems with Optimal Correspondence Assisted Affine Transformation for Evolutionary Multi-Tasking
SSRN Electronic Journal(2022)
摘要
Evolutionary multi-tasking optimization (EMTO) aims to boost the overall efficiency of optimizing multiple tasks by triggering knowledge transfer among them. Unfortunately, it may suffer from negative transfer on heterogeneous composite tasks that have low similarity. Some studies try to learn an intertask alignment transformation based on the paired samples from the involved tasks, but risk a failed alignment with improper pairwise methods. To solve this issue, this study proposes an optimal correspondence assisted affine transformation (OCAT) algorithm. OCAT explicitly constructs a mathematical model for the intertask alignment problem and theoretically deduces its optimal solution in an iterative method. As a result, the sample correspondences that enable the learned transformation to achieve the maximum intertask similarity can be located. Besides, a novel approach to deriving the affine transformation formula is also developed for OCAT. The resulting affine alignment transformation will not impair the knowledge contained in the tasks during the alignment process. By integrating OCAT with the estimation of distribution algorithm, this study finally develops a many-tasking optimization algorithm named MaT-EDA, where the solutions from other tasks are explicitly transferred as the samples for estimating the current distribution model. Extensive simulation studies have indicated that OCAT can significantly enhance the performance of EMTO, and MaT-EDA also achieves impressive many-tasking optimization performance.(c) 2023 Elsevier B.V. All rights reserved.
更多查看译文
关键词
Evolutionary multi-tasking,Intertask alignment,Affine transformation,Many-tasking optimization,Estimation of distribution algorithm
AI 理解论文
溯源树
样例
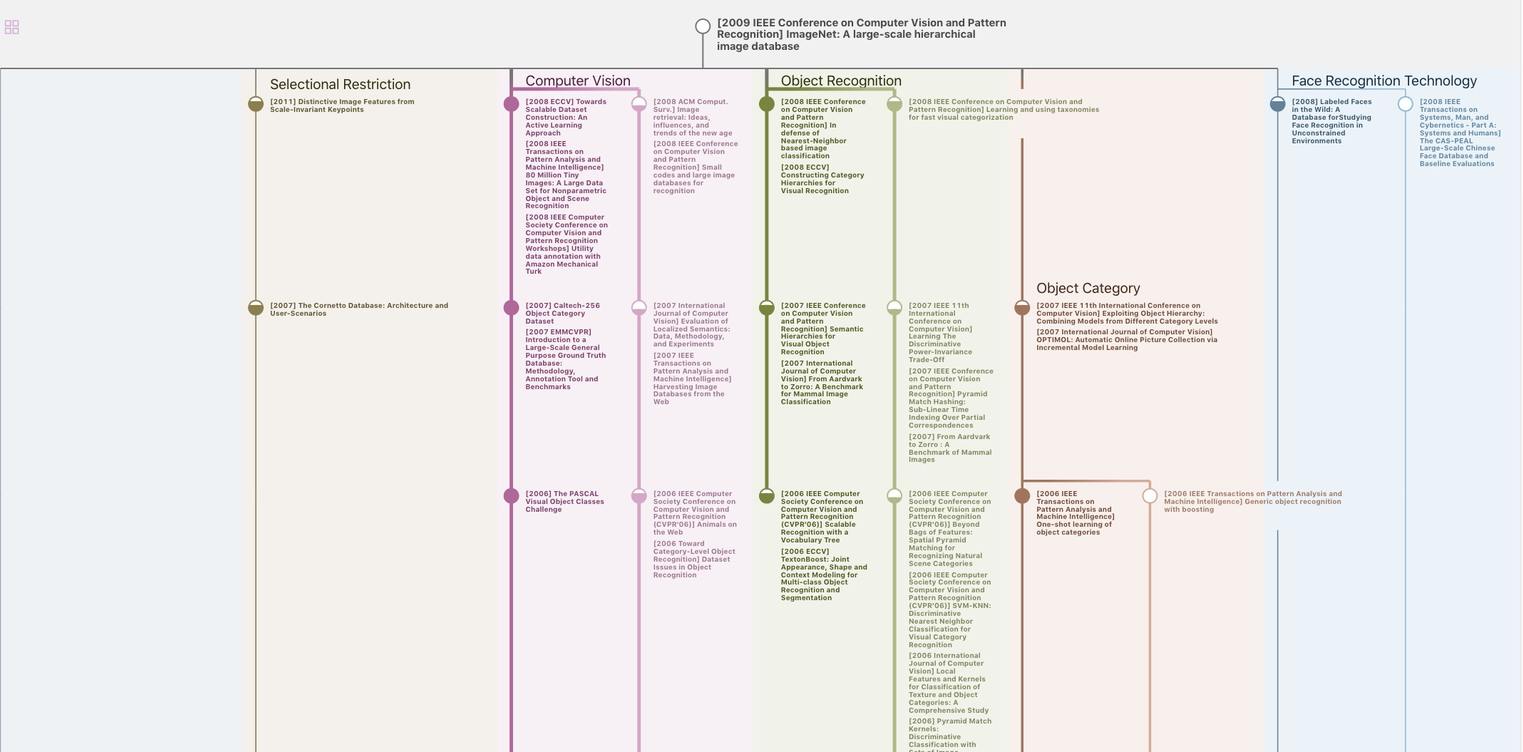
生成溯源树,研究论文发展脉络
Chat Paper
正在生成论文摘要