Trends in Satellite Sensors and Image Time Series Processing Methods for Crop Phenology Monitoring
Springer Optimization and Its ApplicationsInformation and Communication Technologies for Agriculture—Theme I: Sensors(2022)
摘要
National and International space agencies are determined to keep their fingers on the pulse of crop monitoring through Earth Observation (EO) satellites, which is typically tackled with optical imagery. In this regard, there has long been a trade-off between repetition time and spatial resolution. Another limitation of optical remotely sensed data is their typical discontinuity in time, caused by cloud cover or adverse atmospheric effects. Enduring clouds over agricultural fields can mask key stages of crop growth, leading to uncertainties in crop monitoring practices such as yield predictions. Gap-filling methods can provide a key solution for accurate crop phenology characterization. This chapter first provides a historical overview of EO missions dedicated to crop monitoring. Then, it addresses the rapidly evolving fields of gap-filling and land surface phenology (LSP) metrics calculation using a new in-house developed toolbox, DATimeS. These techniques have been put into practice for homogeneous and heterogeneous demonstration landscapes over the United States. Time series of Difference Vegetation Index (DVI) were processed from two EO data sources: high spatial resolution Sentinel-2 and, low spatial resolution MODIS data. LSP metrics such as start and end of season were calculated after gap filling processing at 1km resolution. Over the homogeneous area both S2 and MODIS are well able to capture the phenology trends of the dominant crop and LSP metrics were successfully mapped. Conversely, the MODIS dataset presented more difficulties than S2 to capture the phenology trend of winter wheat over heterogeneous landscape.
更多查看译文
关键词
satellite sensors,crop,monitoring
AI 理解论文
溯源树
样例
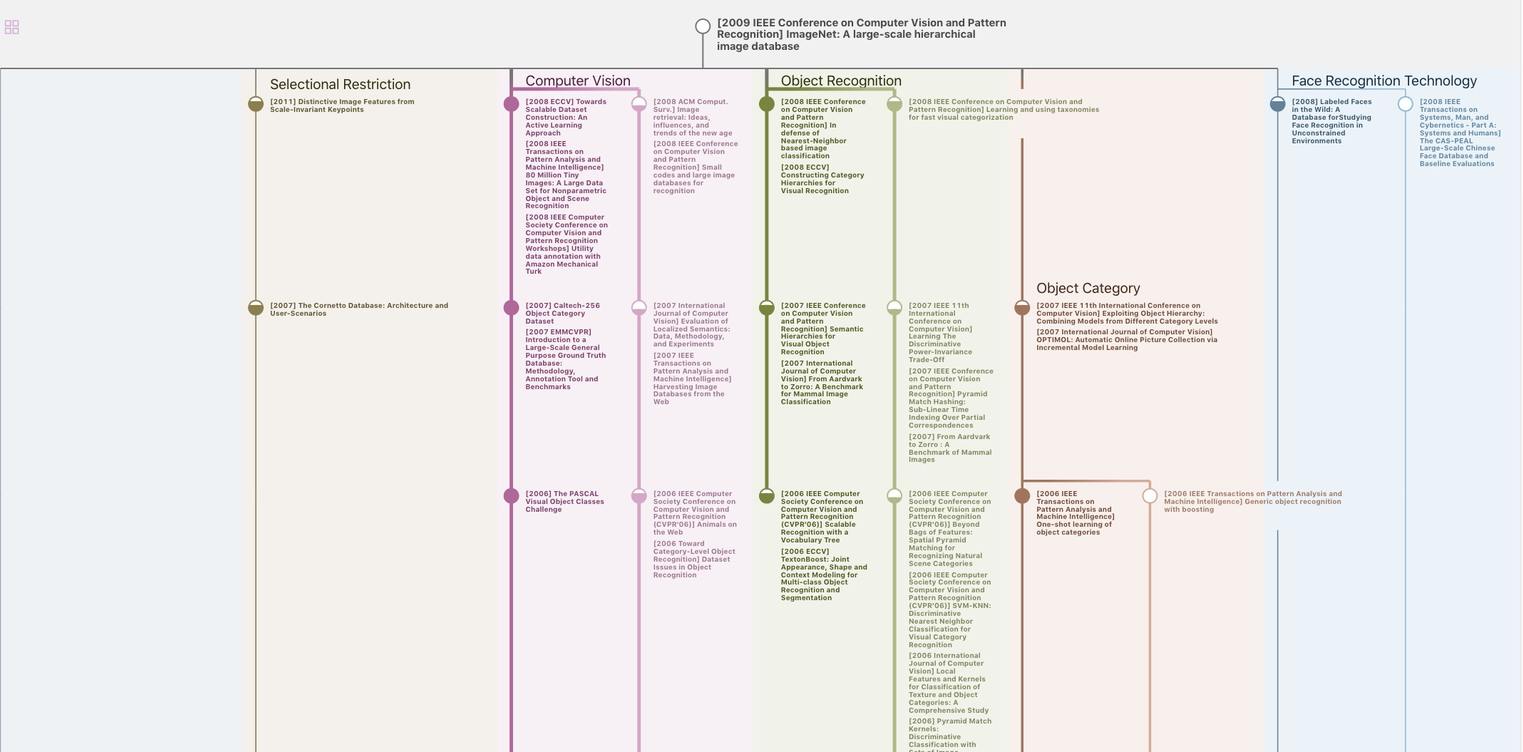
生成溯源树,研究论文发展脉络
Chat Paper
正在生成论文摘要