Cardinality estimation in DBMS
Proceedings of the VLDB Endowment(2021)
摘要
Cardinality estimation (CardEst) plays a significant role in generating high-quality query plans for a query optimizer in DBMS. In the last decade, an increasing number of advanced CardEst methods (especially ML-based) have been proposed with outstanding estimation accuracy and inference latency. However, there exists no study that systematically evaluates the quality of these methods and answer the fundamental problem: to what extent can these methods improve the performance of query optimizer in real-world settings, which is the ultimate goal of a CardEst method. In this paper, we comprehensively and systematically compare the effectiveness of CardEst methods in a real DBMS. We establish a new benchmark for CardEst, which contains a new complex real-world dataset STATS and a diverse query workload STATS-CEB. We integrate multiple most representative CardEst methods into an open-source DBMS PostgreSQL, and comprehensively evaluate their true effectiveness in improving query plan quality, and other important aspects affecting their applicability. We obtain a number of key findings under different data and query settings. Furthermore, we find that the widely used estimation accuracy metric (Q-Error) cannot distinguish the importance of different sub-plan queries during query optimization and thus cannot truly reflect the generated query plan quality. Therefore, we propose a new metric P-Error to evaluate the performance of CardEst methods, which overcomes the limitation of Q-Error and is able to reflect the overall end-to-end performance of CardEst methods. It could serve as a better optimization objective for future CardEst methods.
更多查看译文
关键词
cardinality estimation,dbms
AI 理解论文
溯源树
样例
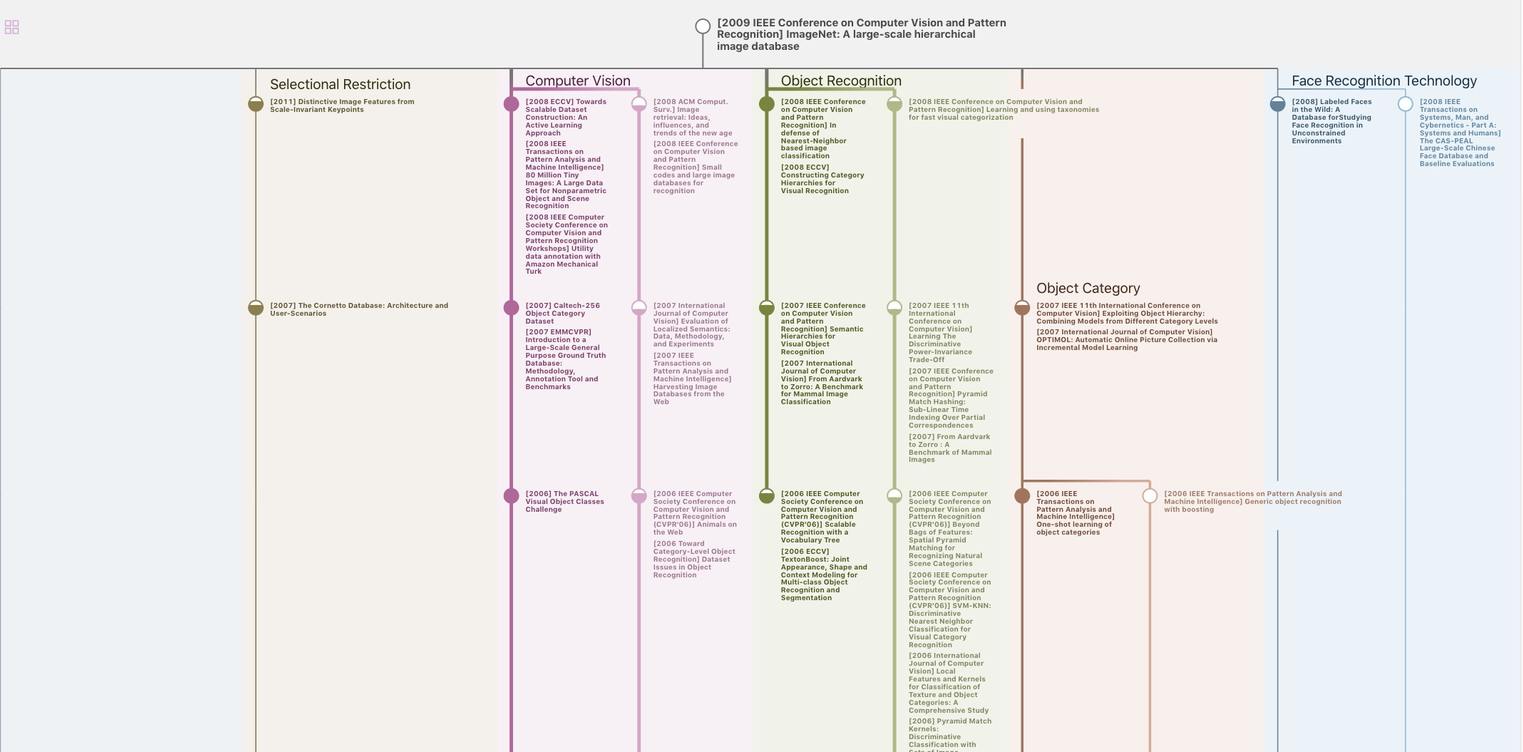
生成溯源树,研究论文发展脉络
Chat Paper
正在生成论文摘要