Generative adversarial minority enlargement-A local linear over-sampling synthetic method
EXPERT SYSTEMS WITH APPLICATIONS(2024)
摘要
The imbalanced data problem is widely recognized in real-world datasets. To avoid learning bias on imbalanced data, the over-sampling strategy is well studied and adopted for generating synthetic minority samples. Wherein, the Synthetic Minority Over-sampling Technology and its improved algorithms become standard baselines. In recent years, the popular Generative Adversarial Networks and its enhanced variants, introduced from the computer vision community, are believed to generate better samples, by approximating the true data distribution. Yet, we notice that the synthetic samples for the minority category in these existing methods is usually restrained in a limited samples space known in advance, which may mislead the classifiers trained on them to take data in the unsampled region of the minority category as from the majority category. Given such limitations, we propose a Generative Adversarial Minority Enlargement (GAME) method to intentionally extend the sampling margin during data generative and adversarial phases. This is accomplished by adjusting the parameters of a local linear model to approach the majority category. We conduct an extensive evaluation on 28 datasets of different domains, extracted from the UCI real-world datasets. The results show that GAME can achieve more balanced and stable results efficiently than 18 state-of-the-art methods.
更多查看译文
关键词
Imbalanced data problem,Generative adversarial model,Synthetic numerical samples,Local linear property of samples,Over-sampling strategy
AI 理解论文
溯源树
样例
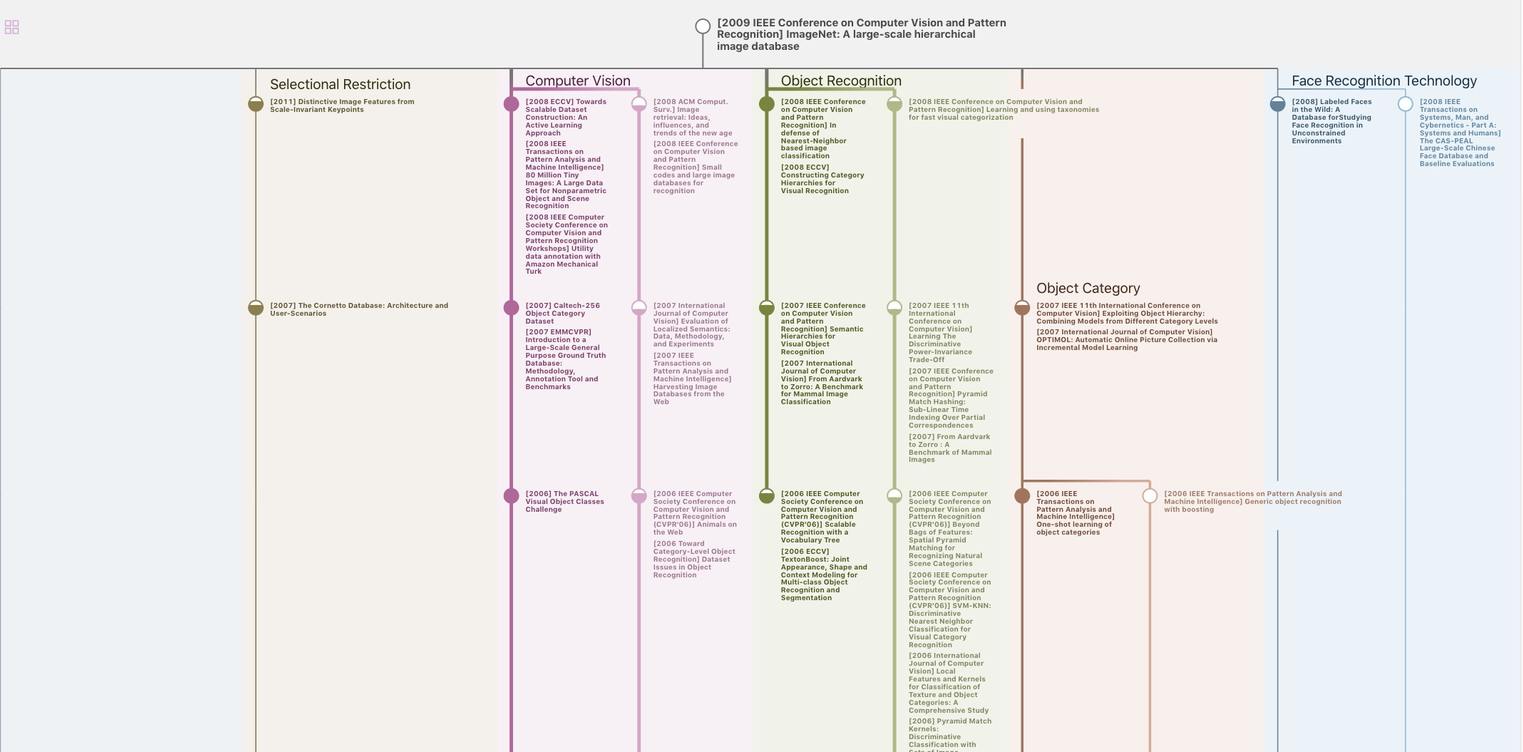
生成溯源树,研究论文发展脉络
Chat Paper
正在生成论文摘要