Assessment of Predicting Frontier Orbital Energies for Small Organic Molecules Using Knowledge-Based and Structural Information
ACS Engineering Au(2022)
摘要
A systematic comparison is demonstrated for the predictions of frontier orbital energies – HOMO (EH), LUMO (EL), and energy gap (ΔEHL) of the molecules in QM9 dataset, where it contains 120k-plus three-dimensional organic molecule structures determined by first-principle simulations. The target molecular properties (EH, EL, and ΔEHL) are predicted using the linear regression (LR), machine learning (random forest, RF), and continuous-filter convolutional neural network (SchNET) approaches. LR and RF models built upon various knowledge-based descriptors, being derived from SMILES of the molecules, can provide predictivity of the target properties with the mean-absolute-errors (MAEs) at 4-6 times of chemical accuracy (0.043 eV). The best approach – SchNET, using the graph representation derived from molecular Cartesian coordinates, is confirmed to provide MAEs of EH, EL, and ΔEHL at 0.051, 0.041, and 0.076 eV, respectively. With the introduction of bond-step matrix representation with SchNET model, the computational cost of dataset preparation can be substantially reduced, and the corresponding MAEs increases moderately to 2-3 times of chemical accuracy. The chemical interpretation of the important descriptors identified in the LR and RF models appear to align with the chemical knowledge of describing these molecular electronic properties, however, being accompanied with tolerable prediction errors. The combination of bond-step representation and SchNET model can provide an assessable-and-balanced option for the high-throughput screening of organic molecules and the preparation of data science approach.
更多查看译文
AI 理解论文
溯源树
样例
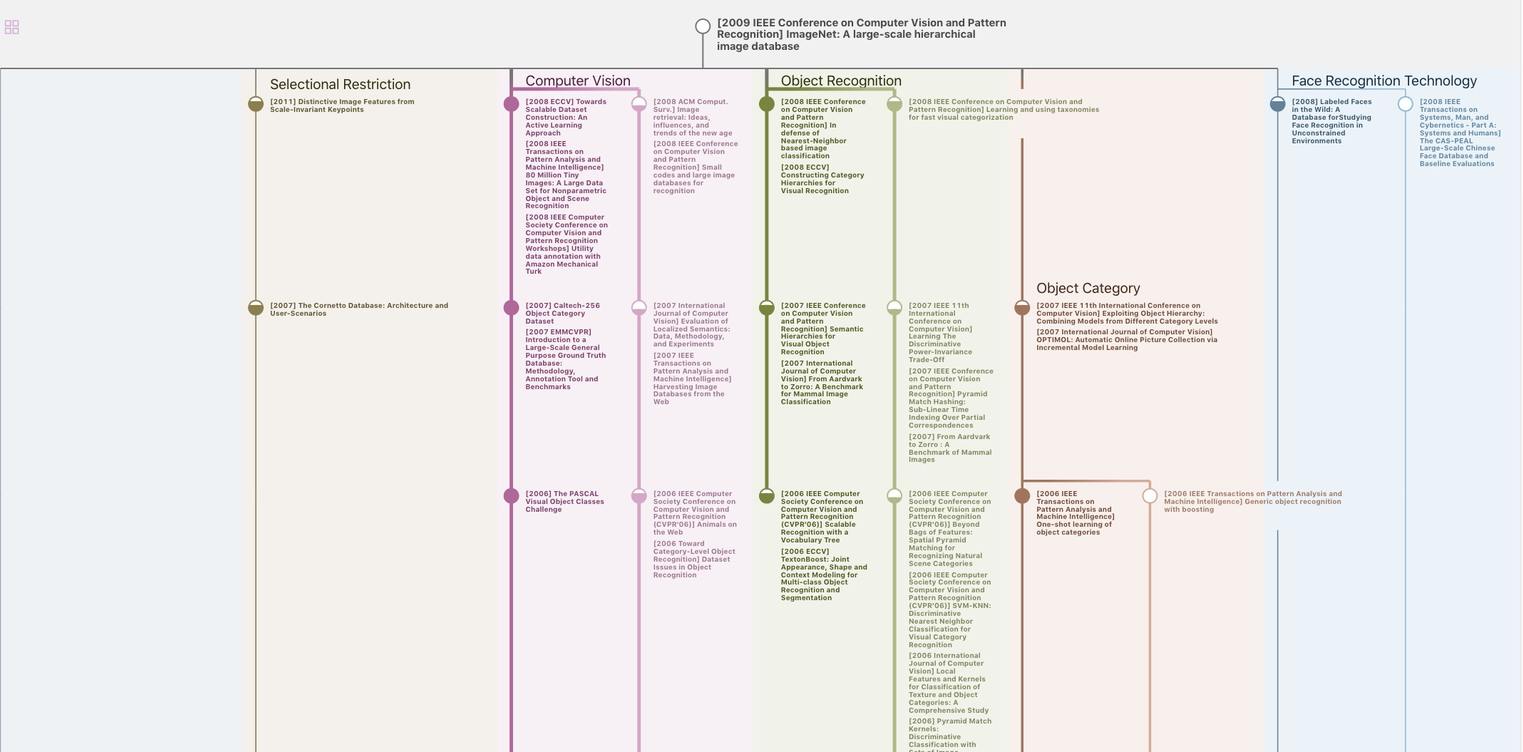
生成溯源树,研究论文发展脉络
Chat Paper
正在生成论文摘要