Subsurface Digital Models for Automated Drilling Risk Prediction in West Kuwait Jurassic Oilfields
Day 1 Mon, May 02, 2022(2022)
摘要
Abstract The objective of this work is to showcase the application of subsurface digital models for the prediction of drilling risks through a systematic analysis of historic drilling events, the modeling of subsurface fluid hydrodynamic and geomechanical processes, and the post-processing of results using artificial intelligence (AI). We demonstrate the field application of this approach for deep drilling risk prediction into Jurassic formations of 3 oilfields of West Kuwait, including a series of blind tests with a newly drilled well. The study departs from the cataloging of historical drilling data for 3 neighboring fields and its segmentation into distinct indicators of in-situ formation response to drilling operations through prestine and depleting formations. The historical data was limited to the subset of events that have known causal relationships with priors such as faults/ fracture or overpressured/ depleted zones coming from subsurface measurements. Thus, the analysis was complemented with the collection and analysis of core scratch tests, rock mechanics laboratory tests, and the processing logging datasets of 40 wells distributed across all fields. By spatially distributing this information via seismic inversion data, we created subsurface models with sufficient detail to perform large scale finite element simulation of in-situ forces and used these results to train a drilling risk prediction model to replicate observations from historical drilling experience. Once a good fit was obtained, we proceeded to make predictions on future wells of the field development plan. The first set of results confirmed our ability to properly replicate historical drilling risks for all 40 wells based on the appropriate prediction model fine-tuning. Predictions are reported as risk probabilities of 4 main event type categories, namely kick, losses, instability, and mechanical. The system was implemented as a bespoke software toolbox and exposed to subsurface experts involved in the study of all 3 fields. A series of blind tests were then carried out where risk predictions were made on a newly drilled well in order to compare with actual drilling performance. Predictions were associated with the likelihood of distinct drilling risks for different effective circulation densities (ECD) values along the length of the well. Interestingly, our results matched the unseen observations with a surprising level of detail. The main contribution of this work is a machine learning based expert system to systematically assimilate historical drilling experience in order to train subsurface models to predict drilling risks using subsurface priors encoded in core, well logging, and seismic data. In the same way, we demonstrate how, once a subsurface drilling risk model is in place, real-time monitoring of drilling risks can be enhanced with data and models that are traditionally not used in operational departments, thus serving as a prime example to achieve cost-effective and saferdrilling operations enabled by new digital and organizational processes.
更多查看译文
AI 理解论文
溯源树
样例
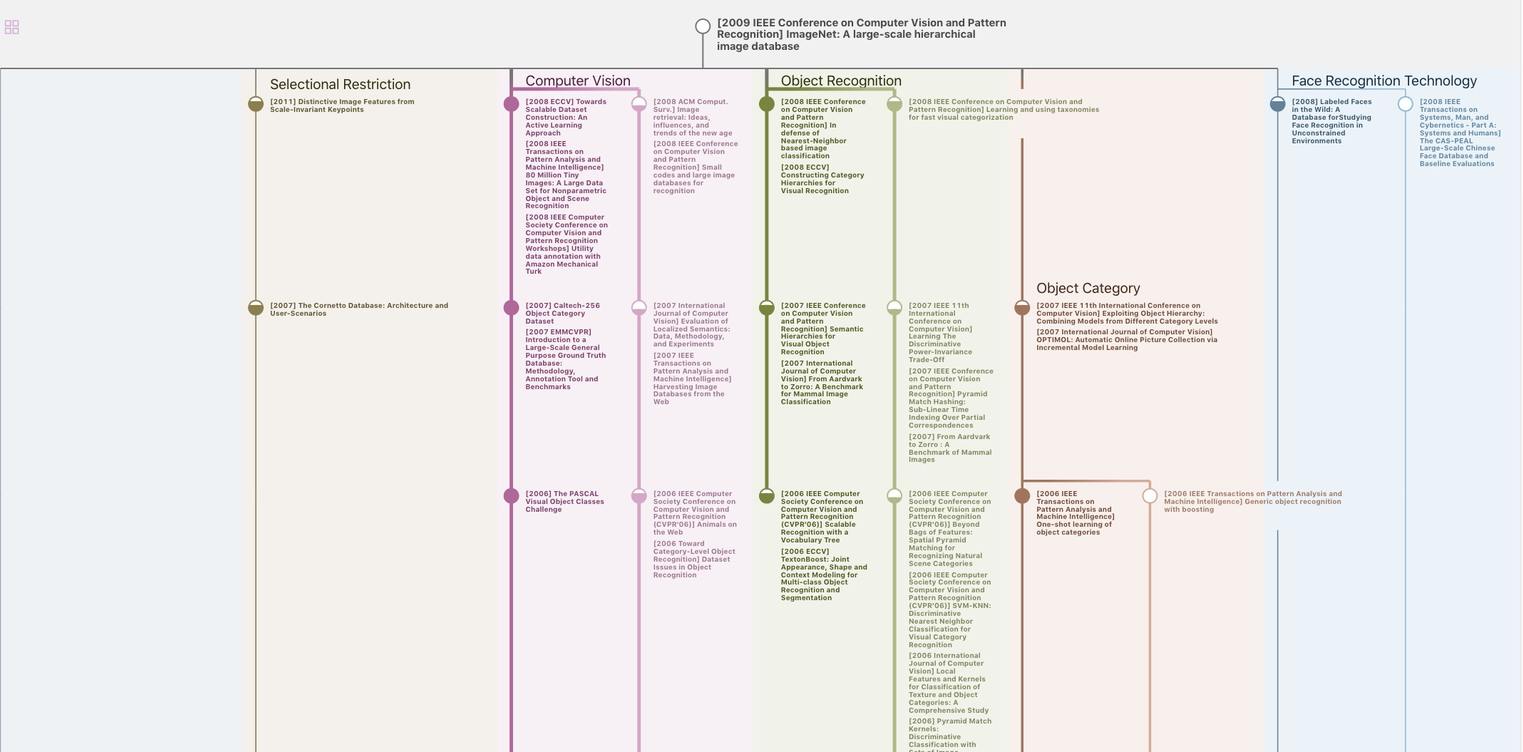
生成溯源树,研究论文发展脉络
Chat Paper
正在生成论文摘要