OA08 Development of an automated deep learning-based system for distinguishing between ‘systemic sclerosis' and ‘normal' capillaries
Rheumatology(2022)
摘要
Abstract Background/Aims Nailfold capillaroscopy is a key investigation in differentiating between primary and systemic sclerosis (SSc)-related Raynaud’s phenomenon (RP). However, interpreting capillaroscopy images (i.e. distinguishing ‘normal’ capillaries of primary RP [PRP] from abnormal capillaries of SSc) can be challenging. Our objectives were: (1) to develop a complete deep learning-based system to identify and classify capillary apices, and compute useful parameters such as width, shape score and vessel density; and (2) use the measured parameters to train and test a logistic regressor to distinguish between subjects with SSc and those with ‘normal’ capillary appearance. Methods 911 mosaic images were included for image-level feature extraction. 456 mosaic images (104 healthy control [HC], 83 PRP, 269 SSc) were used for training and the remaining 455 mosaic images (104 HC, 83 PRP and 268 SSc) were used for validation and testing the deep learning model. For subject-level classification 133 subjects (105 [79%] female, median age [range] 57 [19-80]) were used; 87 (25 HC, 14 PRP, and 48 SSc) were used for training and the remaining 46 (12 HC, 3 PRP, 31 SSc) for testing the logistic regressor. Images from patients with PRP and healthy subjects were grouped for all cases. The deep learning system uses a UNet architecture for capillary detection and vessel segmentation (density and shape score estimation). A ResNet-34 framework is used for capillary classification (distal vs. non-distal) and computing vessel widths. Area under Receiver Operating Characteristic (ROC) curves (AUC) measures the classification accuracy when assigning individuals to SSc and HC/PRP groups. Repeatability experiments were performed with different random seeds to validate the reproducibility of the results. Results For apex detection, the sensitivity and average false positive count from the Free-response Receiver Operating Characteristic (FROC) curve were 0.91 and 127.6, respectively. Apex classification accuracy (distal apices) was 93.8%, with an AUC of 0.92 and sensitivity of 0.9603. Overall AUC for subject level classification (HC/PRP vs SSc) for the current approach was 0.9739 ± 0.0207. Conclusion We have developed a completely automated nailfold capillary detection and analysis system based on deep learning. Apex detection, distal classification, and subsequent computation of distal apex measurements from nailfold capillaroscopy images are all performed with no human input. We have combined image-level features and have built a subject-level classifier model which successfully discriminates between SSc and HC/PRP with an improved AUC (i.e. improved sensitivity and specificity) compared to our previous work. We have performed the repeatability experiments with different random seeds and the performance of system is consistent with all the seeds. Disclosure P. Gurunath Bharathi: None. M. Berks: None. A. Herrick: None. G. Dinsdale: None. A. Murray: None. J. Manning: None. S. Wilkinson: None. C. Taylor: None.
更多查看译文
AI 理解论文
溯源树
样例
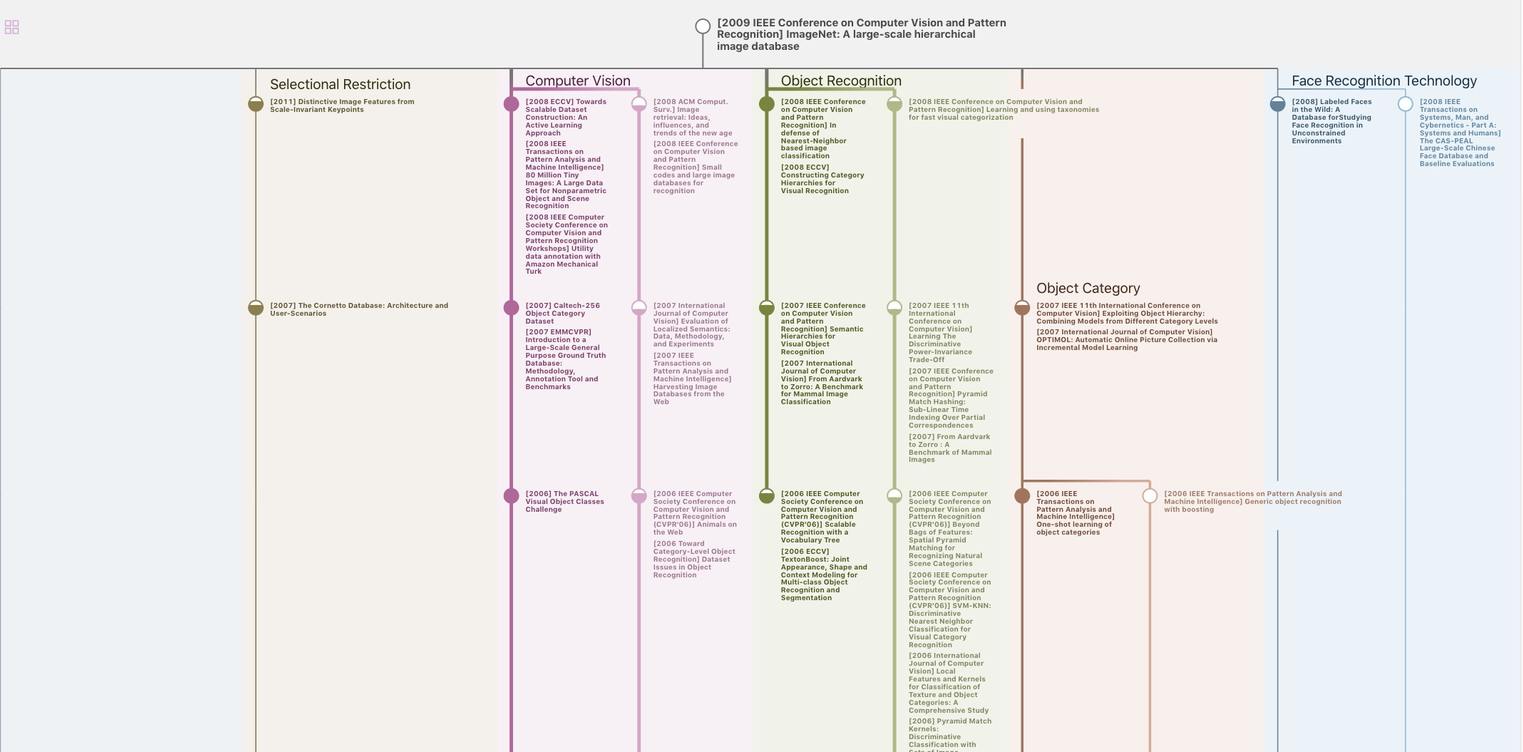
生成溯源树,研究论文发展脉络
Chat Paper
正在生成论文摘要