Quantum Federated Learning With Decentralized Data
IEEE Journal of Selected Topics in Quantum Electronics(2022)
摘要
Variational quantum algorithm (VQA) accesses the centralized data to train the model, and using distributed computing can significantly improve the training overhead; however, the data is privacy sensitive. In this paper, we propose communication-efficient learning of VQA from decentralized data, which is so-called quantum federated learning (QFL). Motivated by the classical federated learning algorithm, we improve data privacy by aggregating updates from local computation to share model parameters. Here, aiming to find approximate optima in the parameter landscape, we develop an extension of the conventional VQA. Finally, we deploy on the TensorFlow Quantum processor within variational quantum tensor networks classifiers, approximate quantum optimization for the Ising model, and variational quantum eigensolver for molecular hydrogen. Our algorithm demonstrates model accuracy from decentralized data, which have higher performance on near-term processors. Importantly, QFL may inspire new investigations in the field of secure quantum machine learning.
更多查看译文
关键词
Quantum algorithm,quantum computing,quantum information,quantum machine learning
AI 理解论文
溯源树
样例
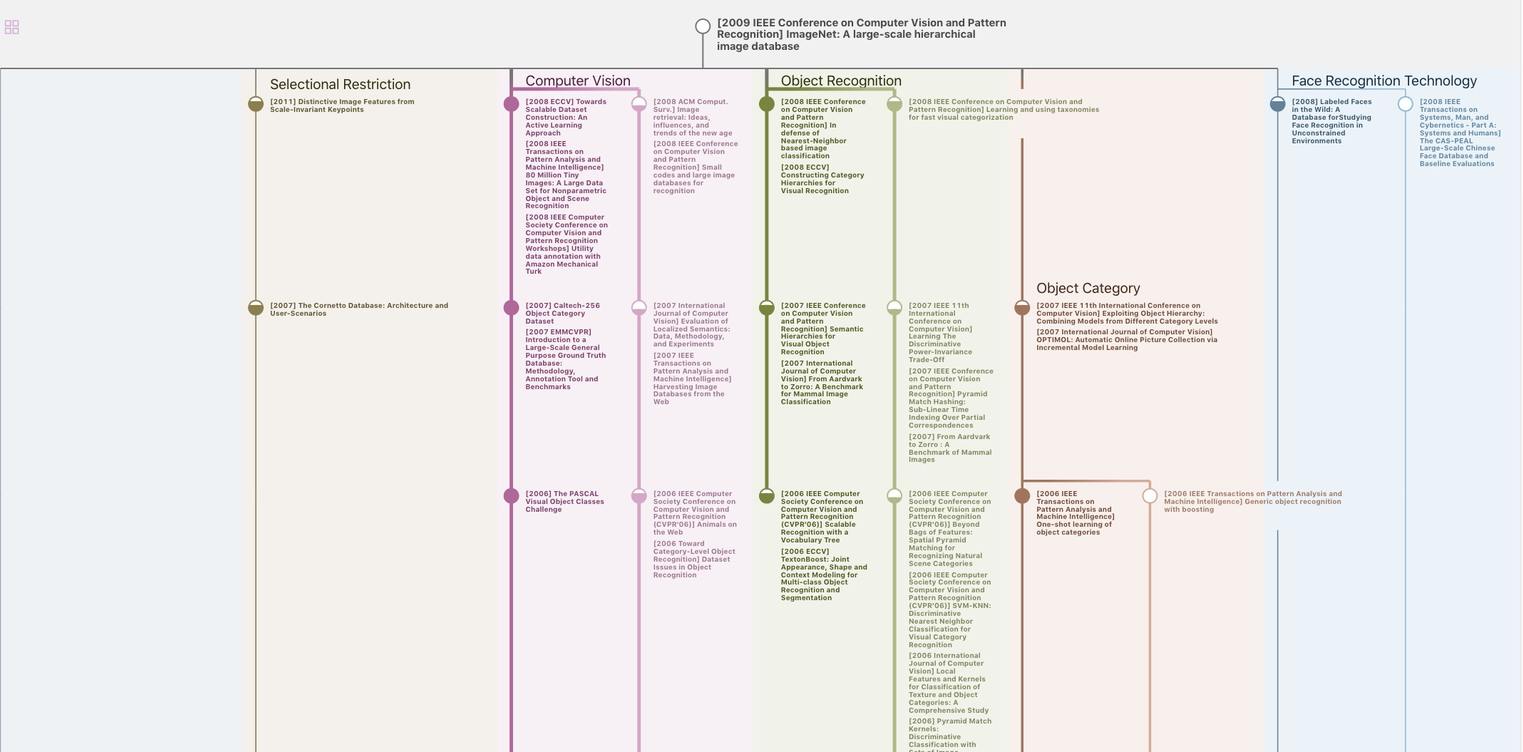
生成溯源树,研究论文发展脉络
Chat Paper
正在生成论文摘要