Composite Style Pixel and Point Convolution-Based Deep Fusion Neural Network Architecture for the Semantic Segmentation of Hyperspectral and Lidar Data
REMOTE SENSING(2022)
摘要
Multimodal hyperspectral and lidar data sets provide complementary spectral and structural data. Joint processing and exploitation to produce semantically labeled pixel maps through semantic segmentation has proven useful for a variety of decision tasks. In this work, we identify two areas of improvement over previous approaches and present a proof of concept network implementing these improvements. First, rather than using a late fusion style architecture as in prior work, our approach implements a composite style fusion architecture to allow for the simultaneous generation of multimodal features and the learning of fused features during encoding. Second, our approach processes the higher information content lidar 3D point cloud data with point-based CNN layers instead of the lower information content lidar 2D DSM used in prior work. Unlike previous approaches, the proof of concept network utilizes a combination of point and pixel-based CNN layers incorporating concatenation-based fusion necessitating a novel point-to-pixel feature discretization method. We characterize our models against a modified GRSS18 data set. Our fusion model achieved 6.6% higher pixel accuracy compared to the highest-performing unimodal model. Furthermore, it achieved 13.5% higher mean accuracy against the hardest to classify samples (14% of total) and equivalent accuracy on the other test set samples.
更多查看译文
关键词
data fusion, multimodal, hyperspectral, lidar, remote sensing, neural network, point convolution
AI 理解论文
溯源树
样例
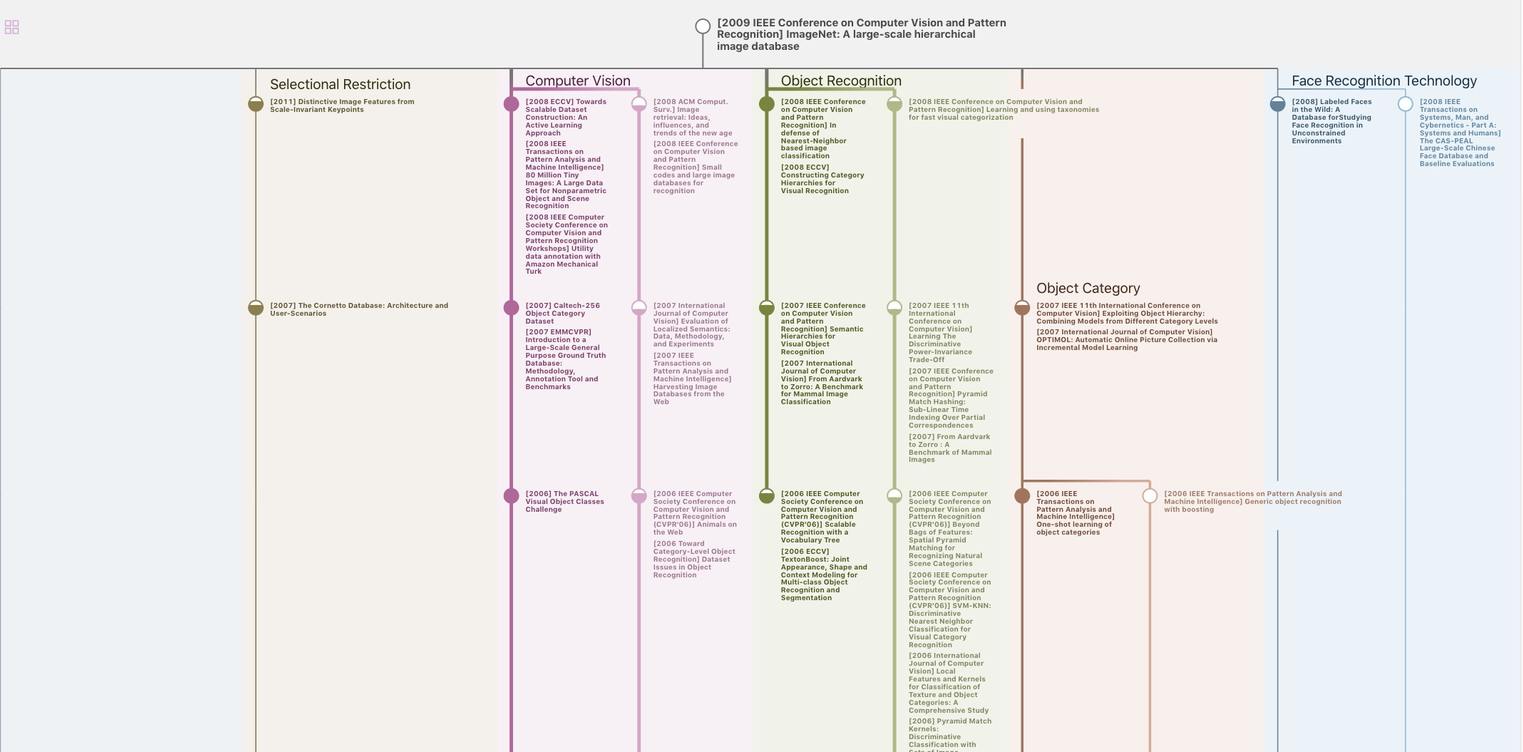
生成溯源树,研究论文发展脉络
Chat Paper
正在生成论文摘要