Automated analysis of continuum fields from atomistic simulations using statistical machine learning
arxiv(2022)
摘要
Atomistic simulations of the molecular dynamics/statics kind are regularly used to study small scale plasticity. Contemporary simulations are performed with tens to hundreds of millions of atoms, with snapshots of these configurations written out at regular intervals for further analysis. Continuum scale constitutive models for material behavior can benefit from information on the atomic scale, in particular in terms of the deformation mechanisms, the accommodation of the total strain and partitioning of stress and strain fields in individual grains. In this work we develop a methodology using statistical data mining and machine learning algorithms to automate the analysis of continuum field variables in atomistic simulations. We focus on three important field variables: total strain, elastic strain and microrotation. Our results show that the elastic strain in individual grains exhibits a unimodal log-normal distribution, whilst the total strain and microrotation fields evidence a multimodal distribution. The peaks in the distribution of total strain are identified with a Gaussian mixture model and methods to circumvent overfitting problems are presented. Subsequently, we evaluate the identified peaks in terms of deformation mechanisms in a grain, which e.g., helps to quantify the strain for which individual deformation mechanisms are responsible. The overall statistics of the distributions over all grains are an important input for higher scale models, which ultimately also helps to be able to quantitatively discuss the implications for information transfer to phenomenological models.
更多查看译文
关键词
atomistic simulations,clustering,data mining,Gaussian mixture model,machine learning,nanocrystalline materials,statistical distribution functions
AI 理解论文
溯源树
样例
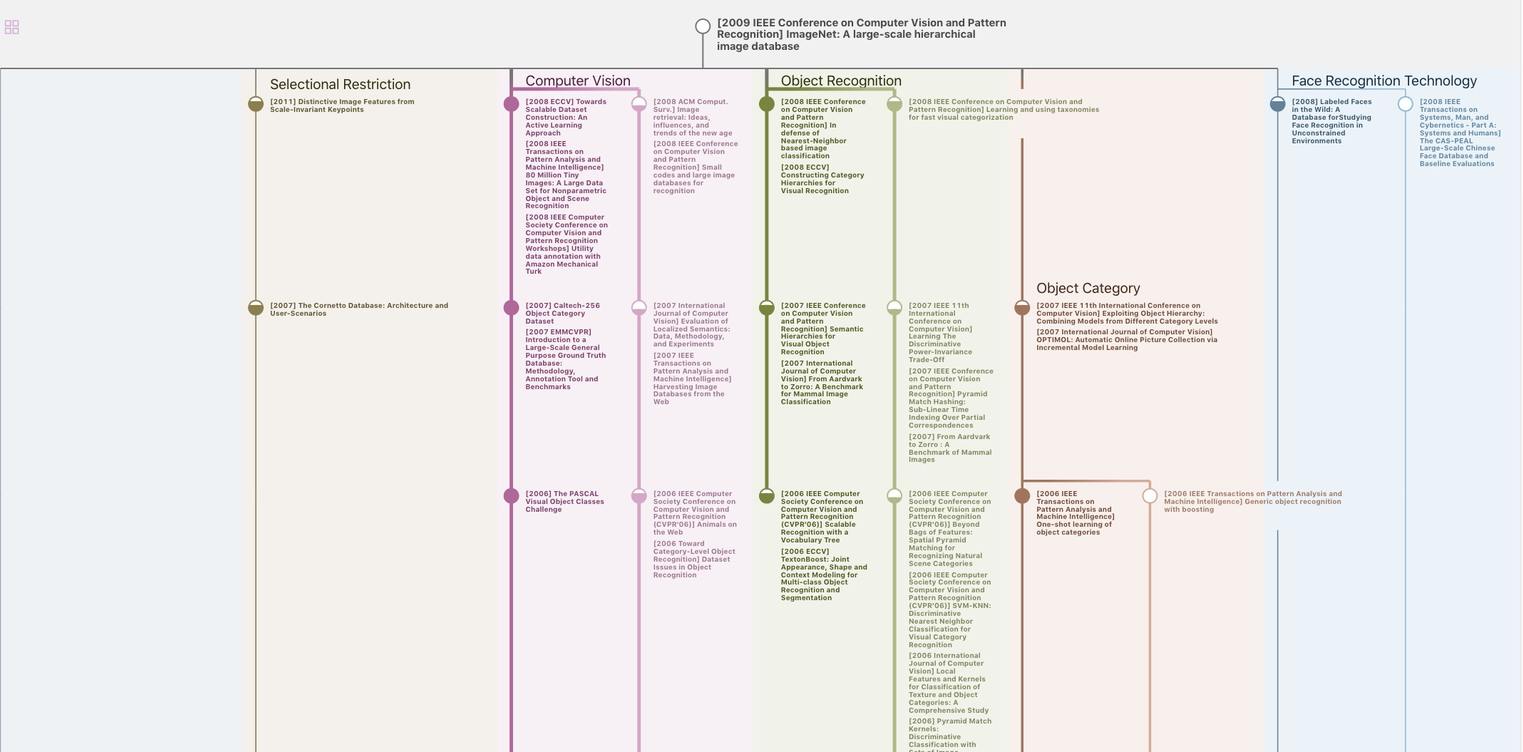
生成溯源树,研究论文发展脉络
Chat Paper
正在生成论文摘要