Depth estimation using an improved stereo network
Frontiers of Information Technology & Electronic Engineering(2022)
摘要
Self-supervised depth estimation approaches present excellent results that are comparable to those of the fully supervised approaches, by employing view synthesis between the target and reference images in the training data. ResNet, which serves as a backbone network, has some structural deficiencies when applied to downstream fields, because its original purpose was to cope with classification problems. The low-texture area also deteriorates the performance. To address these problems, we propose a set of improvements that lead to superior predictions. First, we boost the information flow in the network and improve the ability to learn spatial structures by improving the network structures. Second, we use a binary mask to remove the pixels in low-texture areas between the target and reference images to more accurately reconstruct the image. Finally, we input the target and reference images randomly to expand the dataset and pre-train it on ImageNet, so that the model obtains a favorable general feature representation. We demonstrate state-of-the-art performance on an Eigen split of the KITTI driving dataset using stereo pairs.
更多查看译文
关键词
Monocular depth estimation,Self-supervised,Image reconstruction
AI 理解论文
溯源树
样例
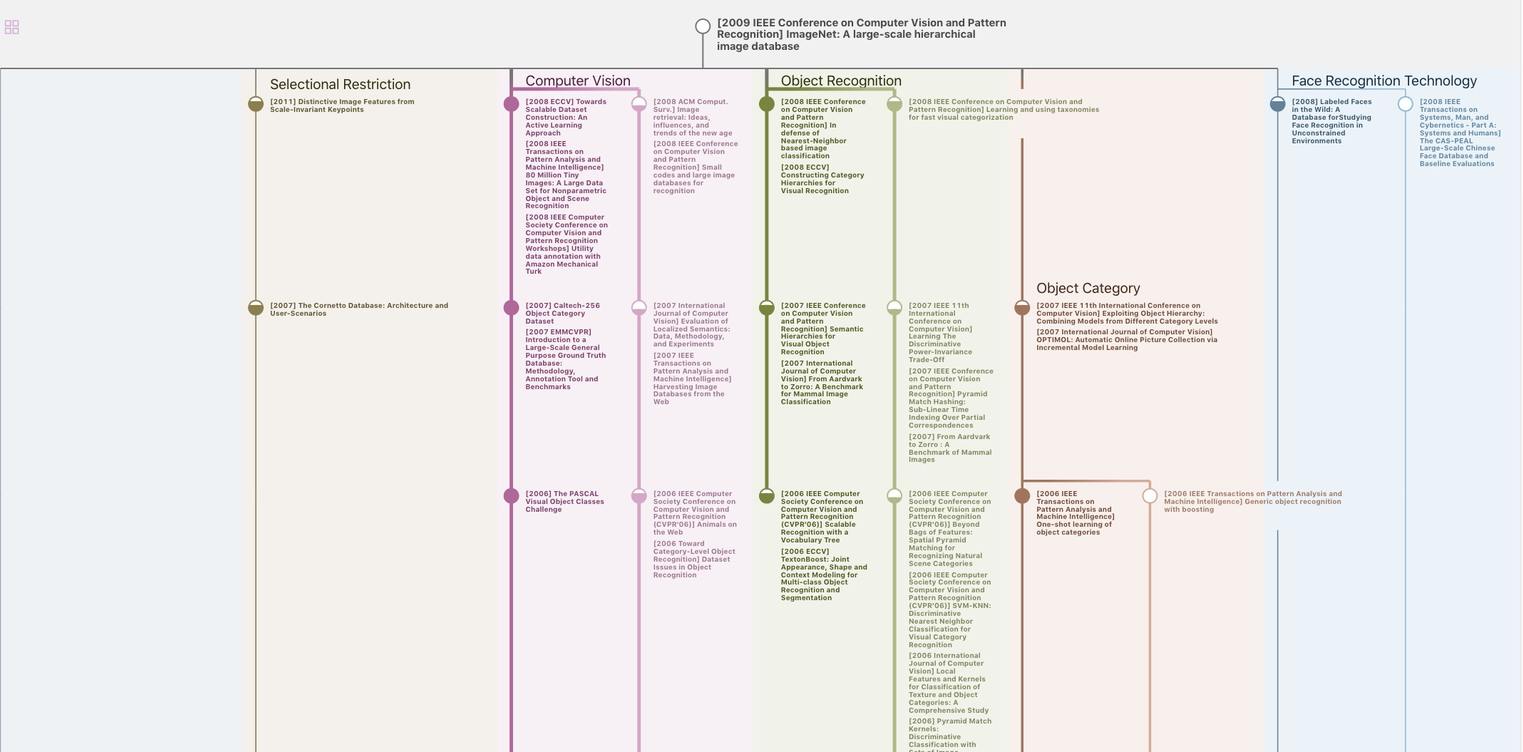
生成溯源树,研究论文发展脉络
Chat Paper
正在生成论文摘要