Local Identifiability of Deep ReLU Neural Networks: the Theory
NeurIPS 2022(2022)
摘要
Is a sample rich enough to determine, at least locally, the parameters of a neural network? To answer this question, we introduce a new local parameterization of a given deep ReLU neural network by fixing the values of some of its weights. This allows us to define local lifting operators whose inverses are charts of a smooth manifold of a high dimensional space. The function implemented by the deep ReLU neural network composes the local lifting with a linear operator which depends on the sample. We derive from this convenient representation a geometrical necessary and sufficient condition of local identifiability. Looking at tangent spaces, the geometrical condition provides: 1/ a sharp and testable necessary condition of identifiability and 2/ a sharp and testable sufficient condition of local identifiability. The validity of the conditions can be tested numerically using backpropagation and matrix rank computations.
更多查看译文
关键词
Deep Learning,ReLU networks,Conditions of identifiability,Lifting operator
AI 理解论文
溯源树
样例
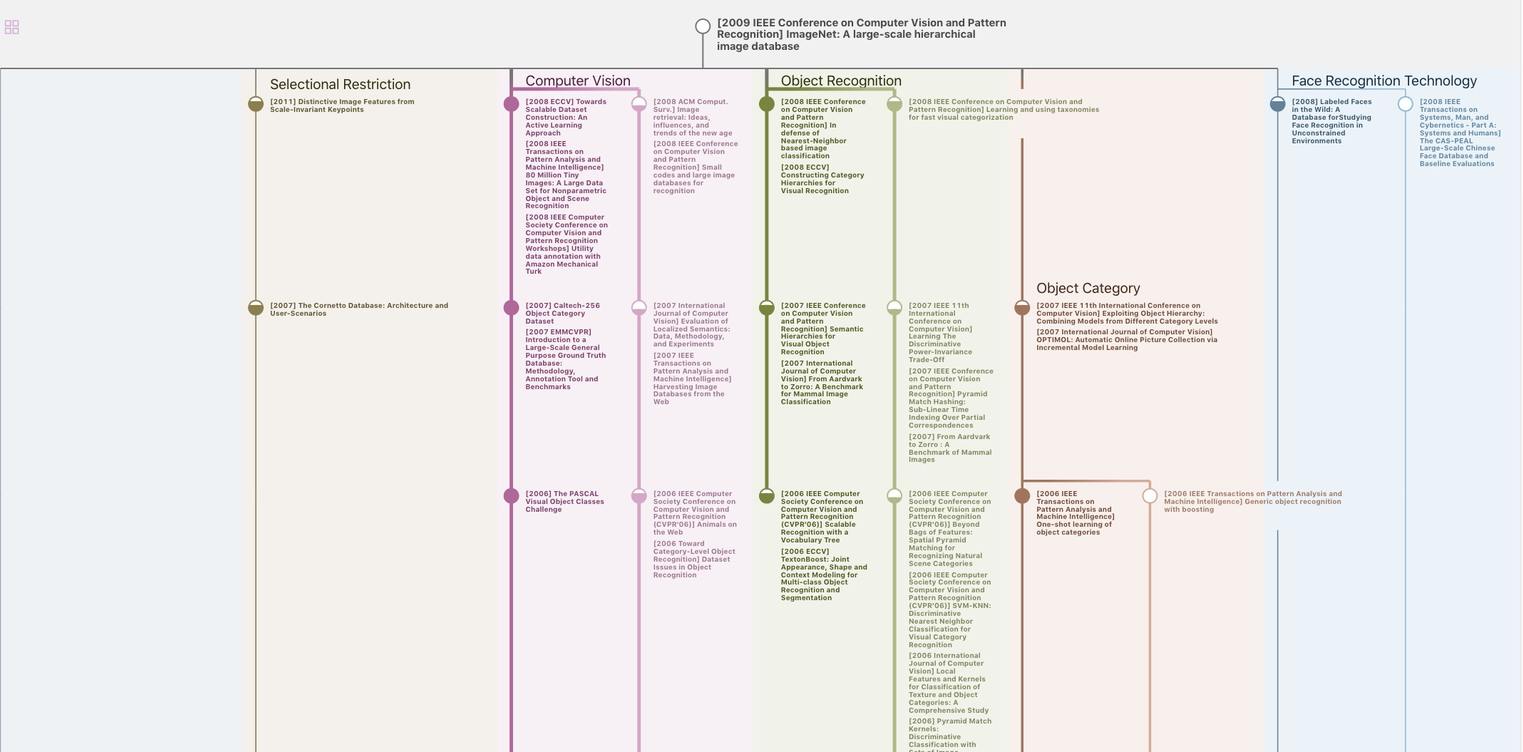
生成溯源树,研究论文发展脉络
Chat Paper
正在生成论文摘要