Computational modeling of threat learning reveals links with anxiety and neuroanatomy in humans
ELIFE(2022)
摘要
Influential theories implicate variations in the mechanisms supporting threat learning in the severity of anxiety symptoms. We use computational models of associative learning in conjunction with structural imaging to explicate links among the mechanisms underlying threat learning, their neuroanatomical substrates, and anxiety severity in humans. We recorded skin-conductance data during a threat-learning task from individuals with and without anxiety disorders (N=251; 8-50 years; 116 females). Reinforcement-learning model variants quantified processes hypothesized to relate to anxiety: threat conditioning, threat generalization, safety learning, and threat extinction. We identified the best-fitting models for these processes and tested associations among latent learning parameters, whole-brain anatomy, and anxiety severity. Results indicate that greater anxiety severity related specifically to slower safety learning and slower extinction of response to safe stimuli. Nucleus accumbens gray-matter volume moderated learning-anxiety associations. Using a modeling approach, we identify computational mechanisms linking threat learning and anxiety severity and their neuroanatomical substrates.
更多查看译文
关键词
computational modeling, neuroanatomy, anxiety, learning, fear, Human
AI 理解论文
溯源树
样例
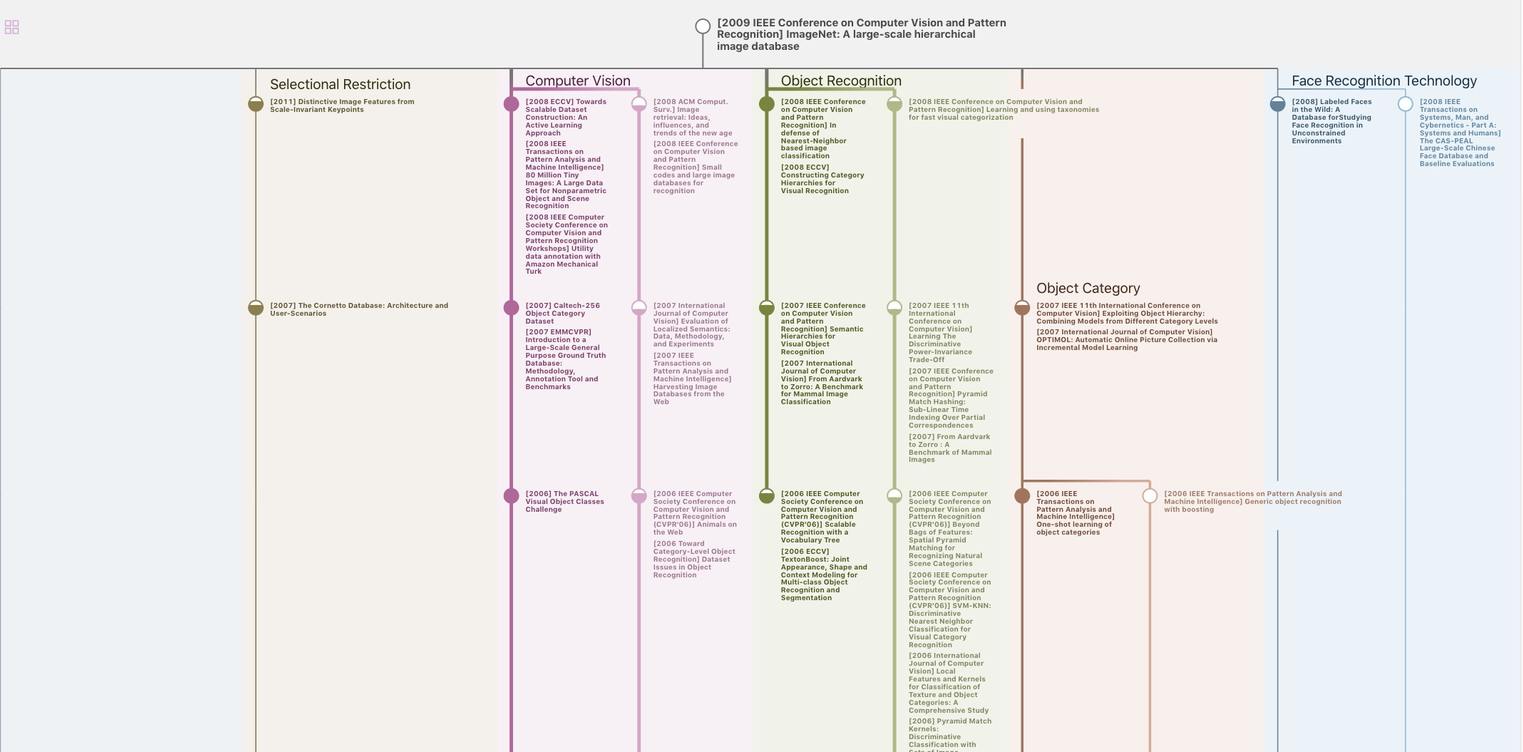
生成溯源树,研究论文发展脉络
Chat Paper
正在生成论文摘要