Fast Convergence of Inertial Dynamics with Hessian-Driven Damping Under Geometry Assumptions
Applied Mathematics and Optimization(2023)
Abstract
First-order optimization algorithms can be considered as a discretization of ordinary differential equations (ODEs) (Su et al. in Adv Neural Inf Process Syst 27, 2014). In this perspective, studying the properties of the corresponding trajectories may lead to convergence results which can be transfered to the numerical scheme. In this paper we analyse the following ODE introduced by Attouch et al. (J Differ Equ 261(10):5734–5783, 2016): ∀ t⩾ t_0, ẍ(t)+α/tẋ(t)+β H_F(x(t))ẋ(t)+∇ F(x(t))=0, where α >0 , β >0 and H_F denotes the Hessian of F . This ODE can be derived to build numerical schemes which do not require F to be twice differentiable as shown in Attouch et al. (Math Program 1–43, 2020) and Attouch et al. (Optimization 72:1–40, 2021). We provide strong convergence results on the error F(x(t))-F^* and integrability properties on ‖∇ F(x(t))‖ under some geometry assumptions on F such as quadratic growth around the set of minimizers. In particular, we show that the decay rate of the error for a strongly convex function is O(t^-α -ε) for any ε >0 . These results are briefly illustrated at the end of the paper.
MoreTranslated text
Key words
Convex optimization,Hessian-driven damping,Lyapunov analysis,Łojasiewicz property,ODEs
AI Read Science
Must-Reading Tree
Example
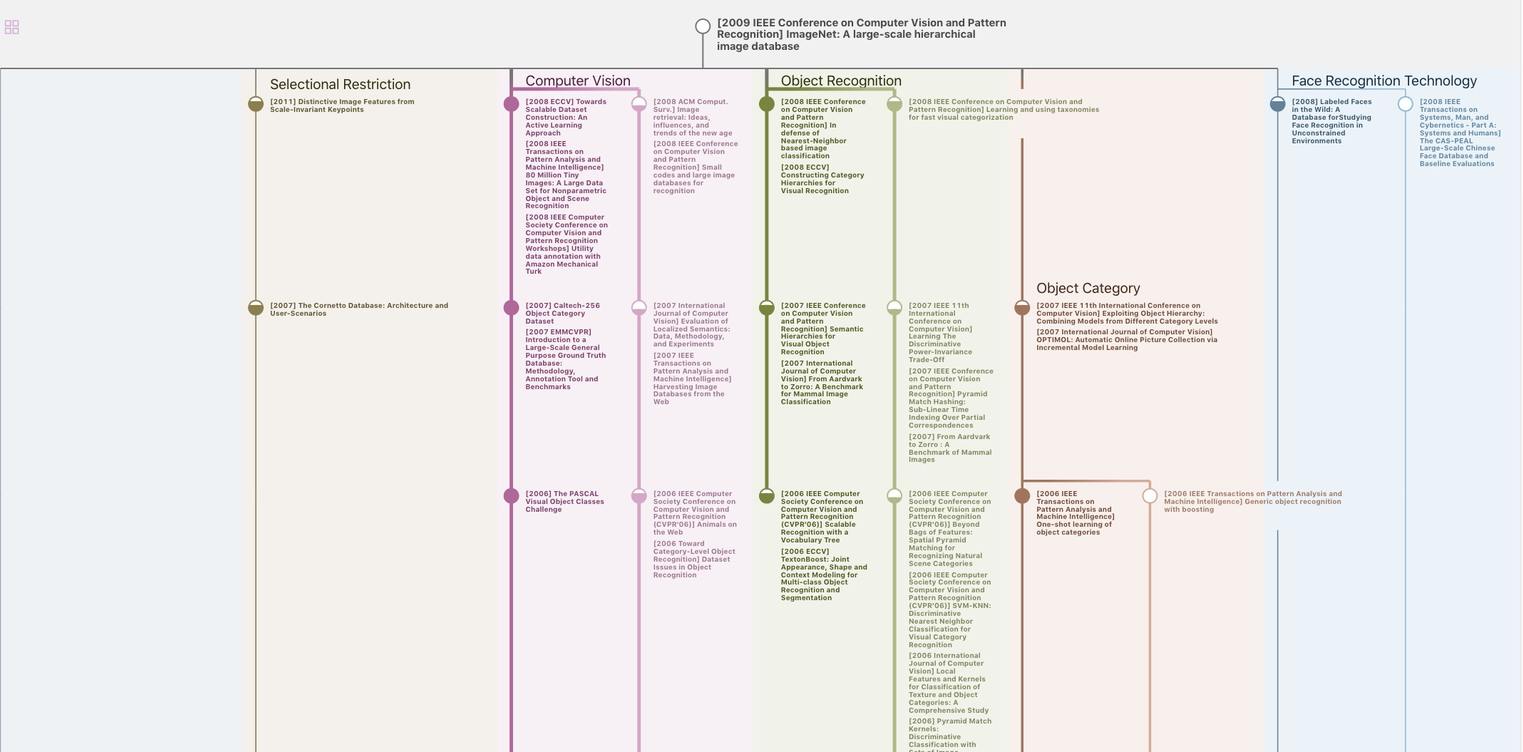
Generate MRT to find the research sequence of this paper
Chat Paper
Summary is being generated by the instructions you defined