A novel MDPSO-SVR hybrid model for feature selection in electricity consumption forecasting
arxiv(2022)
摘要
Electricity consumption forecasting has vital importance for the energy planning of a country. Of the enabling machine learning models, support vector regression (SVR) has been widely used to set up forecasting models due to its superior generalization for unseen data. However, one key procedure for the predictive modeling is feature selection, which might hurt the prediction accuracy if improper features were selected. In this regard, a modified discrete particle swarm optimization (MDPSO) was employed for feature selection in this study, and then MDPSO-SVR hybrid mode was built to predict future electricity consumption. Compared with other well-established counterparts, MDPSO-SVR model consistently performs best in two real-world electricity consumption datasets, which indicates that MDPSO for feature selection can improve the prediction accuracy and the SVR equipped with the MDPSO can be a promised alternative for electricity consumption forecasting.
更多查看译文
关键词
feature selection,hybrid model,mdpso-svr
AI 理解论文
溯源树
样例
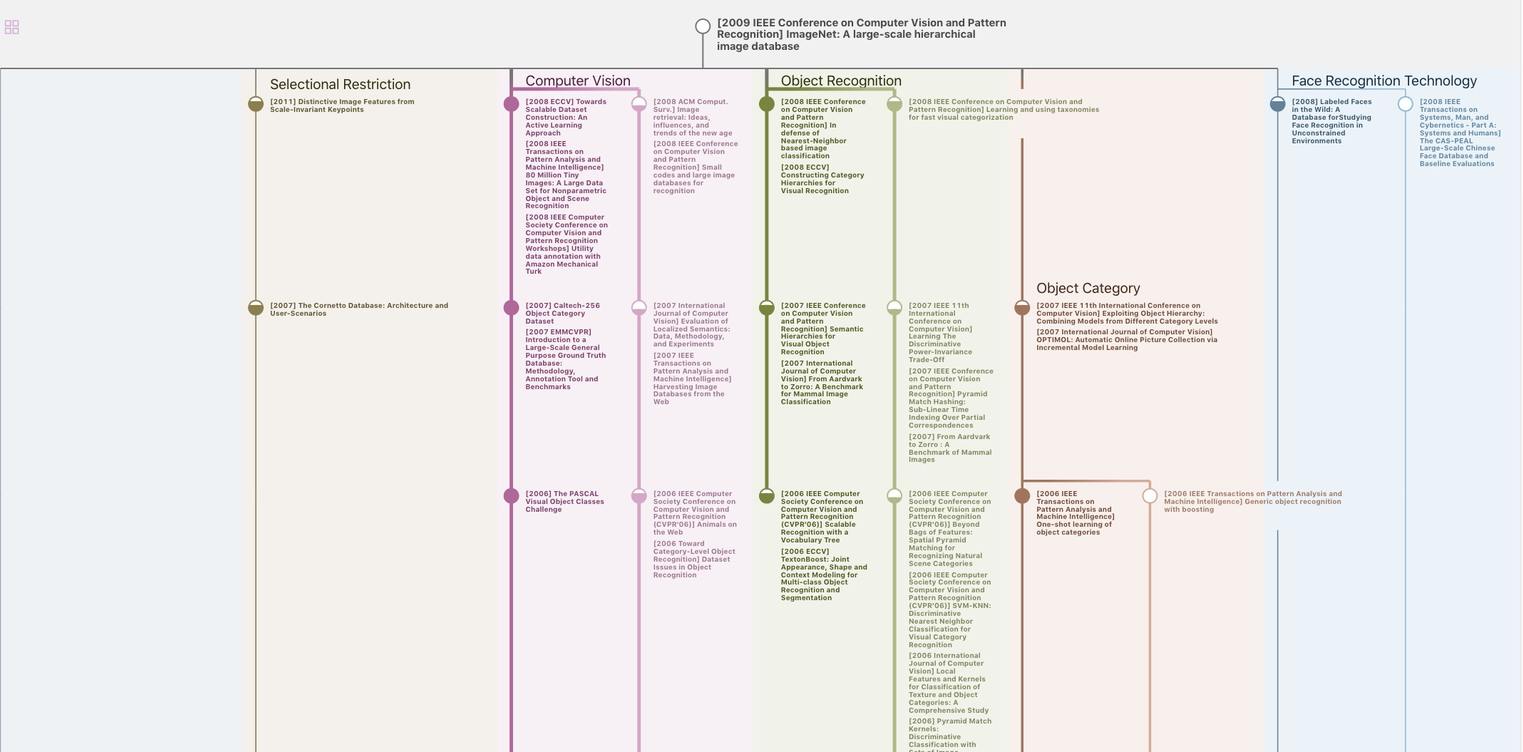
生成溯源树,研究论文发展脉络
Chat Paper
正在生成论文摘要