Semi-Private Computation of Data Similarity with Applications to Data Valuation and Pricing
arxiv(2023)
摘要
Consider two data providers that want to contribute data to a certain learning model. Recent works have shown that the value of the data of one of the providers is dependent on the similarity with the data owned by the other provider. It would thus be beneficial if the two providers can calculate the similarity of their data, while keeping the actual data private. In this work, we devise multiparty computation-protocols to compute similarity of two data sets based on correlation, while offering controllable privacy guarantees. We consider a simple model with two participating providers and develop methods to compute exact and approximate correlation, respectively, with controlled information leakage. Both protocols have computational and communication complexities that are linear in the number of data samples. We also provide general bounds on the maximal error in the approximation case, and analyse the resulting errors for practical parameter choices.
更多查看译文
关键词
data similarity,valuation,pricing,semi-private
AI 理解论文
溯源树
样例
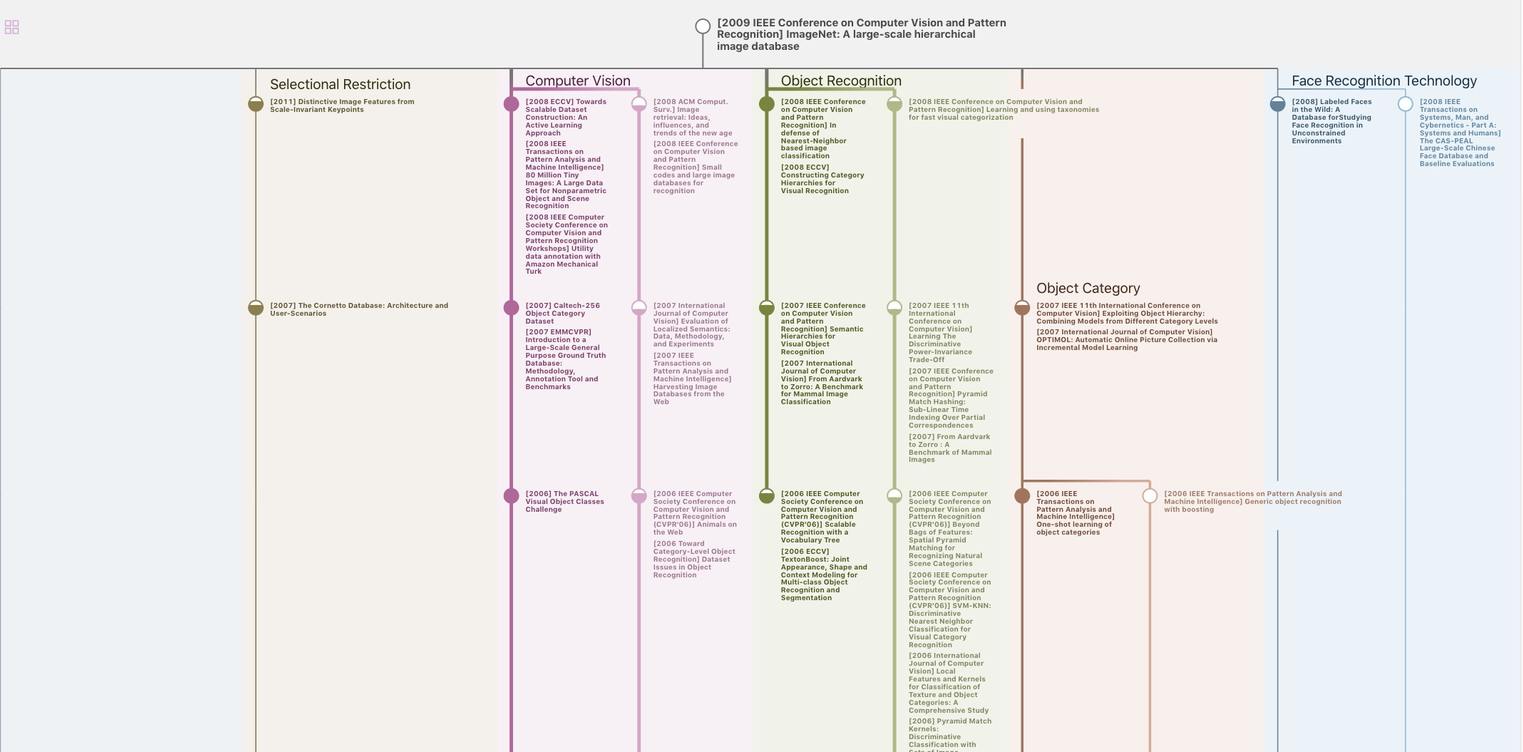
生成溯源树,研究论文发展脉络
Chat Paper
正在生成论文摘要