Stability prediction of gold nanoclusters with different ligands and doped metals: deep learning and experimental tests
JOURNAL OF MATERIALS CHEMISTRY A(2024)
Abstract
The stability or experimental accessibility of gold nanocluster, which is modulated by different ligands and doped metal atoms, is a prerequisite for potential applications in energy materials, catalysis, sensors, bio-imaging, and therapy. For screening out the stable candidates from the complex combination space formed by different metal cores and ligands, it is crucial to predict the stability of the Au nanoclusters before the actual synthesis. A comprehensive dataset is established by determining the formation energies (Eform) of various Au nanocluster structures (including 21 different core sizes, 22 kinds of ligands, 7 kinds of doped metal atoms) using density functional theory (DFT) calculations. A deep learning model, graph convolutional neural network (GCNN), is applied to achieve good performance for formation energy predictions. The applicability of GCNN is further tested using some external test sets, encompassing the synthesized nanoclusters of particular interest due to their responsiveness to stimuli and biocompatibility. The oil-water partition coefficient (log P) of the ligand has great influence on the solubility of Au clusters and cell activity. The predictive deep learning model also guided the successful synthesis of some novel structures, e.g., Au10(PPh3)7Cl3 and Au38OT24 (OT = octane-1-thiol), with the predicted formation energies close to those of known nanoclusters. The proposed machine learning scheme holds promise in facilitating the high-throughput discovery and synthesis of nanoclusters experimentally. The formation energy of gold nanoclusters could be predicted quickly by deep learning.
MoreTranslated text
AI Read Science
Must-Reading Tree
Example
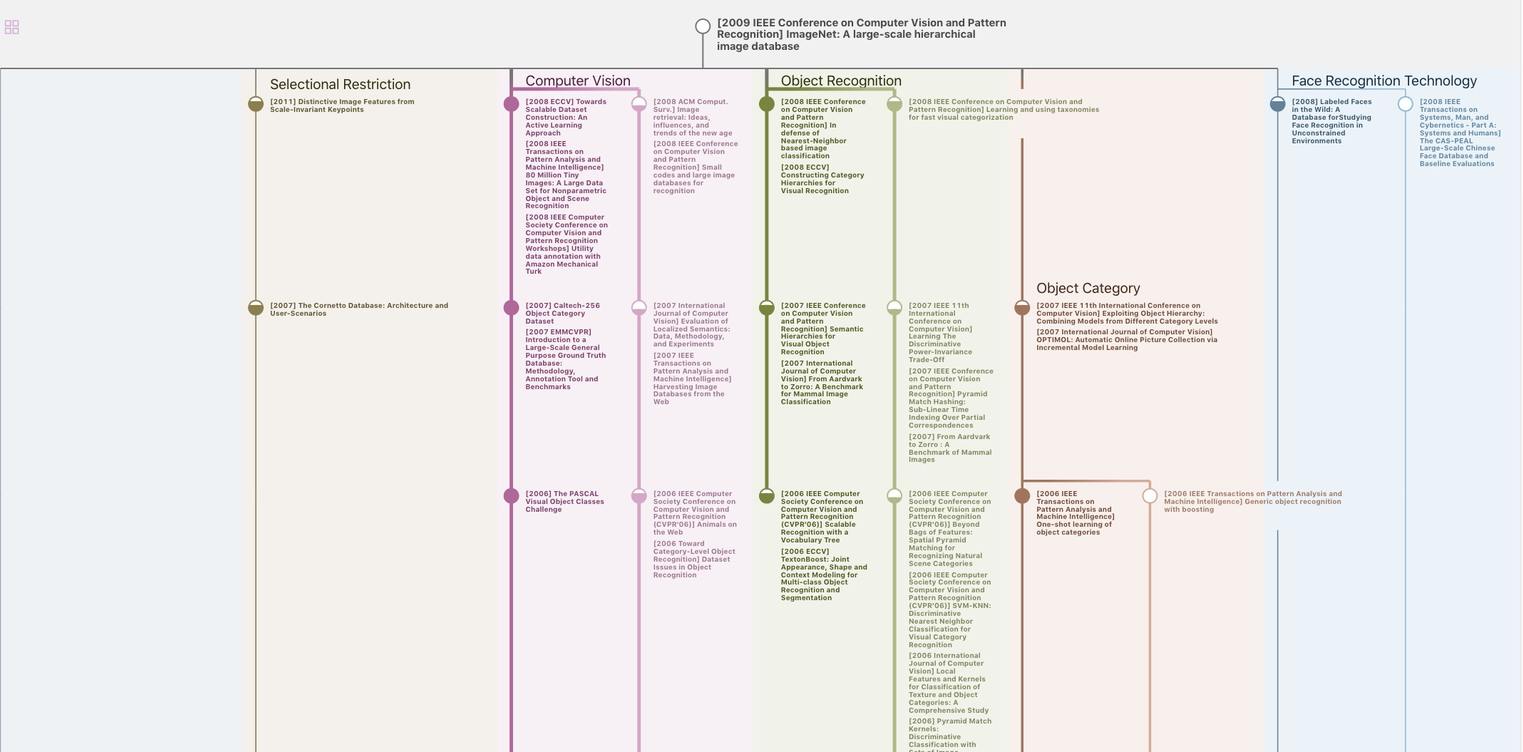
Generate MRT to find the research sequence of this paper
Chat Paper
Summary is being generated by the instructions you defined