Infrared Small Vehicle Detection Based on Parallel Fusion Network
Acta Photonica Sinica(2022)
Abstract
The high-precision infrared small vehicle detection under disturbed background has a high application value. The existing small IR vehicle detection methods usually fail or cause a high probability of false alarm in the complex backgrounds, fuzzy targets and overlapping targets. A detection method base on parallel fusion network is proposed to solve the above problems. First, a network based on the parallel residual block is designed as the backbone to complete high-precision and robust detection of the targets. Then, an improving YOLOv3 algorithm based on cross-layer connection is established which can make full use of the underlying information to complete high-precision detection and positioning of small infrared vehicle targets. Finally, the soft-NMS is employed instead of the NMS to alleviate the problem of overlapping targets. Experimental results show that the method in this paper can accurately detect infrared small vehicles in complex moving backgrounds and achieve a high detection rate in the case of a low false alarm rate. The false alarm rate is only 0.01% and the missed detection rate is only 1.36%.The PaRNet backbone network proposed in this paper has high accuracy and convergence rate, and more importantly, it has a certain degree of robustness, and also has a certain ability to recognize fuzzy targets in complex environments. On the other hand, our improved algorithm adds cross-layer connections and deeper feature fusion, so that the network has a fuller understanding of the low-level small goals. The finally introduced soft-NMS algorithm also solves the overlap problem caused by the overlapping cars to a certain extent.
MoreTranslated text
Key words
Pattern recognition, Infrared image, Vehicle detection, Small object detection, Parallel fusion
AI Read Science
Must-Reading Tree
Example
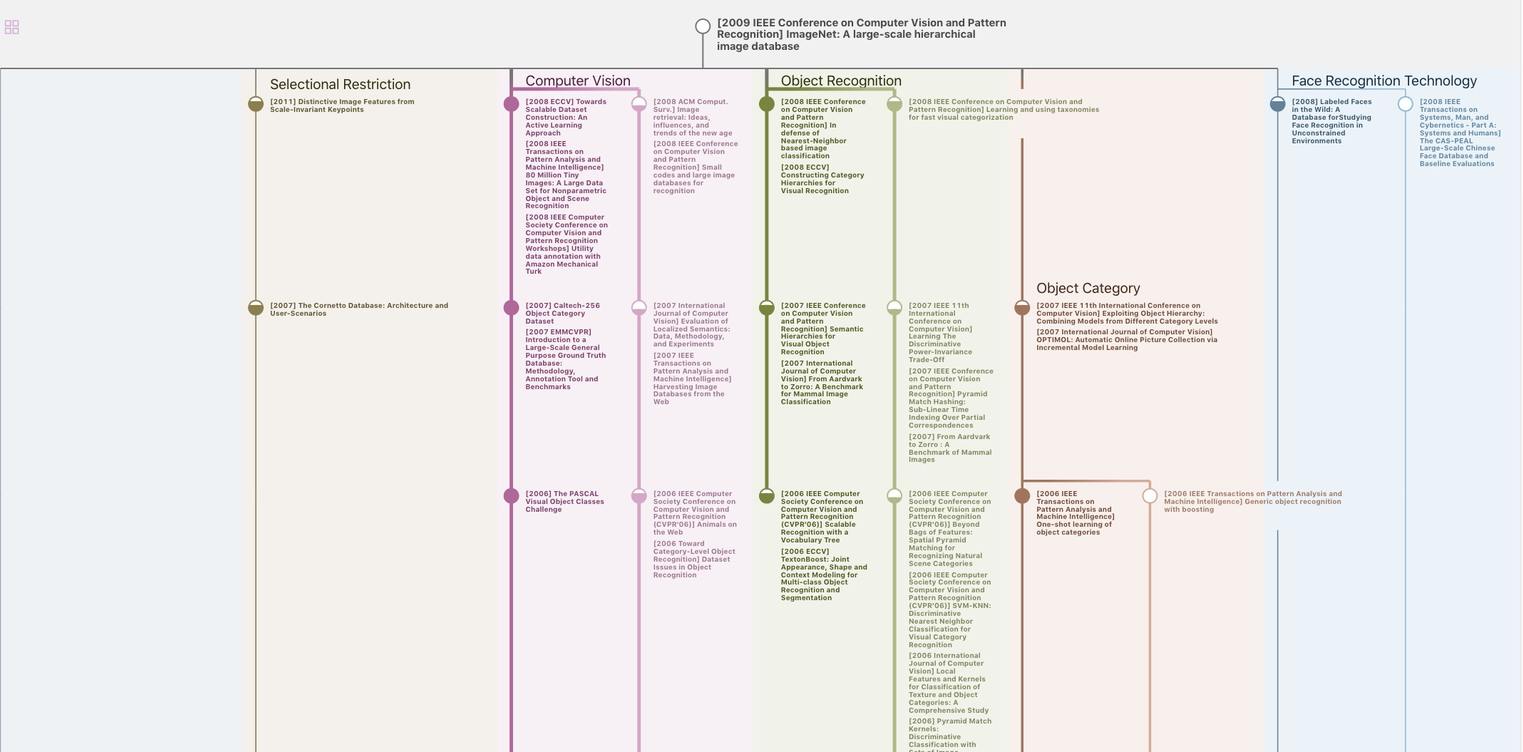
Generate MRT to find the research sequence of this paper
Chat Paper
Summary is being generated by the instructions you defined