Calibrating the Classical Hardness of the Quantum Approximate Optimization Algorithm
PRX QUANTUM(2023)
Abstract
Trading fidelity for scale enables approximate classical simulators such as matrix product states (MPS) to run quantum circuits beyond exact methods. A control parameter, the so-called bond dimension $\chi$ for MPS, governs the allocated computational resources and the output fidelity. Here, we characterize the fidelity for the quantum approximate optimization algorithm by the expectation value of the cost function it seeks to minimize and find that it follows a scaling law $F\bigl(\ln\chi\bigr/N\bigr)$ with $N$ the number of qubits. With $\ln\chi$ amounting to the entanglement that an MPS can encode, we show that the relevant variable for investigating the fidelity is the entanglement per qubit. Importantly, our results calibrate the classical computational power required to achieve the desired fidelity and benchmark the performance of quantum hardware in a realistic setup. For instance, we quantify the hardness of performing better classically than a noisy superconducting quantum processor by readily matching its output to the scaling function. Moreover, we relate the global fidelity to that of individual operations and establish its relationship with $\chi$ and $N$. We sharpen the requirements for noisy quantum computers to outperform classical techniques at running a quantum optimization algorithm in speed, size, and fidelity.
MoreTranslated text
Key words
classical hardness,quantum,optimization algorithm
AI Read Science
Must-Reading Tree
Example
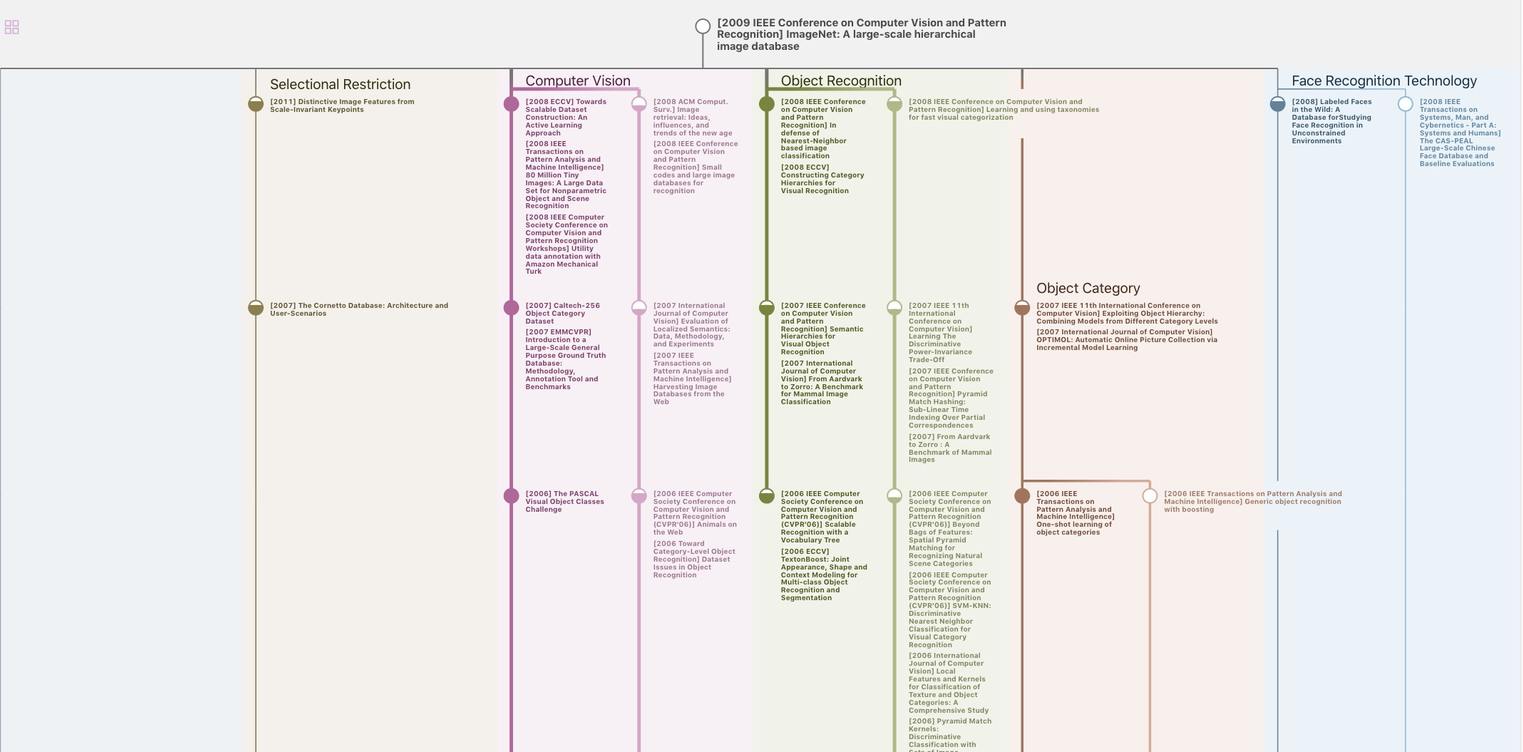
Generate MRT to find the research sequence of this paper
Chat Paper
Summary is being generated by the instructions you defined