Exploring Structure-aware Transformer over Interaction Proposals for Human-Object Interaction Detection
IEEE Conference on Computer Vision and Pattern Recognition(2022)
摘要
Recent high-performing Human-Object Interaction (HOI) detection techniques have been highly influenced by Transformer-based object detector (i.e., DETR). Nevertheless, most of them directly map parametric interaction queries into a set of HOI predictions through vanilla Transformer in a one-stage manner. This leaves rich interor intra-interaction structure under-exploited. In this work, we design a novel Transformer-style HOI detector, i.e., Structure-aware Transformer over Interaction Proposals (STIP), for HOI detection. Such design decomposes the process of HOI set prediction into two subsequent phases, i.e., an interaction proposal generation is first performed, and then followed by transforming the non-parametric interaction proposals into HOI predictions via a structure-aware Transformer. The structure-aware Transformer upgrades vanilla Transformer by encoding additionally the holistically semantic structure among interaction proposals as well as the locally spatial structure of human/object within each interaction proposal, so as to strengthen HOI predictions. Extensive experiments conducted on V-COCO and HICO-DET benchmarks have demonstrated the effectiveness of STIP, and superior results are reported when comparing with the state-of-the-art HOI detectors. Source code is available at https://github.com/zyong812/STIP.
更多查看译文
关键词
Scene analysis and understanding
AI 理解论文
溯源树
样例
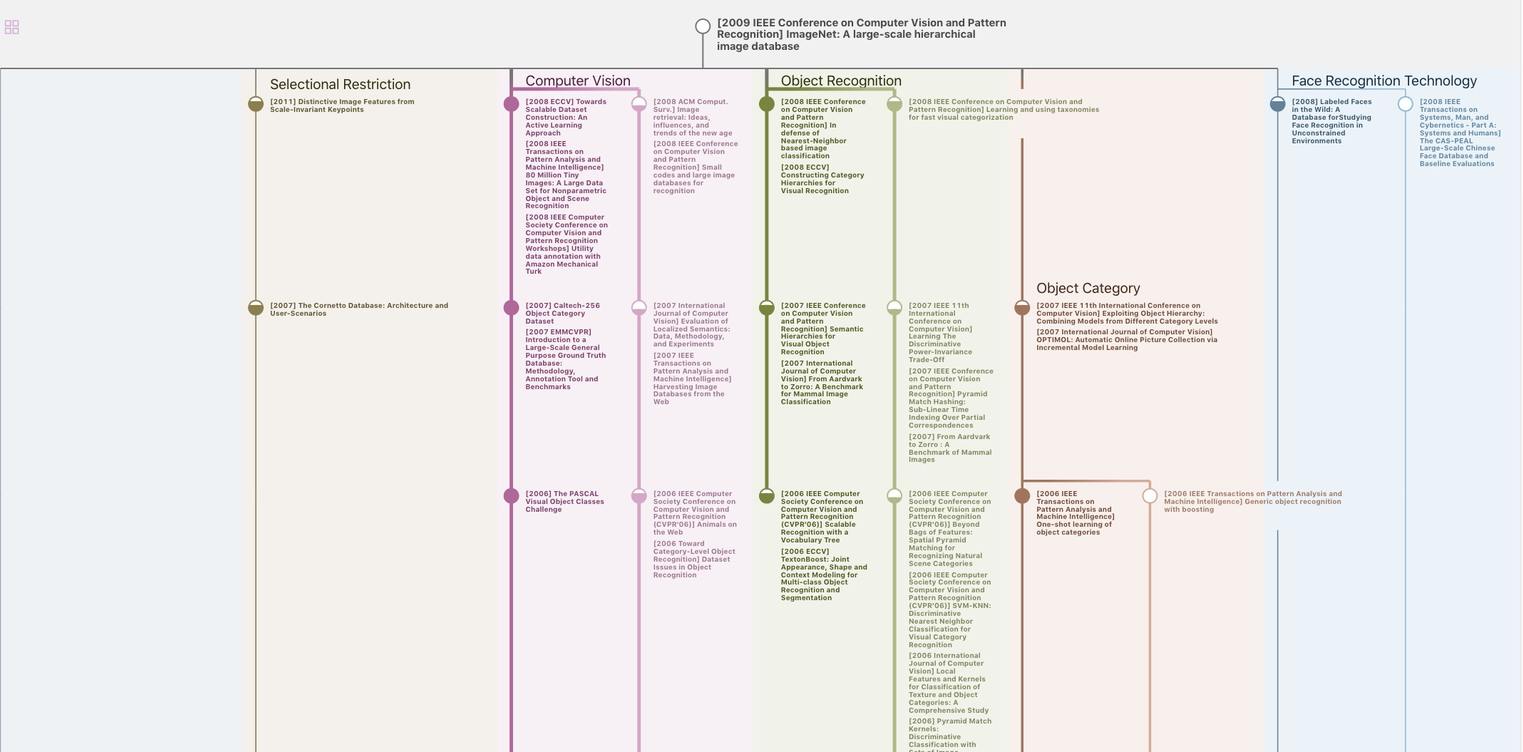
生成溯源树,研究论文发展脉络
Chat Paper
正在生成论文摘要