Fusing Feature Engineering and Deep Learning: A Case Study for Malware Classification
arxiv(2022)
摘要
Machine learning has become an appealing signature-less approach to detect
and classify malware because of its ability to generalize to never-before-seen
samples and to handle large volumes of data. While traditional feature-based
approaches rely on the manual design of hand-crafted features based on experts
knowledge of the domain, deep learning approaches replace the manual feature
engineering process by an underlying system, typically consisting of a neural
network with multiple layers, that perform both feature learning and
classification altogether. However, the combination of both approaches could
substantially enhance detection systems. In this paper we present an hybrid
approach to address the task of malware classification by fusing multiple types
of features defined by experts and features learned through deep learning from
raw data. In particular, our approach relies on deep learning to extract N-gram
like features from the assembly language instructions and the bytes of malware,
and texture patterns and shapelet-based features from malwareś grayscale
image representation and structural entropy, respectively. These deep features
are later passed as input to a gradient boosting model that combines the deep
features and the hand-crafted features using an early-fusion mechanism. The
suitability of our approach has been evaluated on the Microsoft Malware
Classification Challenge benchmark and results show that the proposed solution
achieves state-of-the-art performance and outperforms gradient boosting and
deep learning methods in the literature.
更多查看译文
AI 理解论文
溯源树
样例
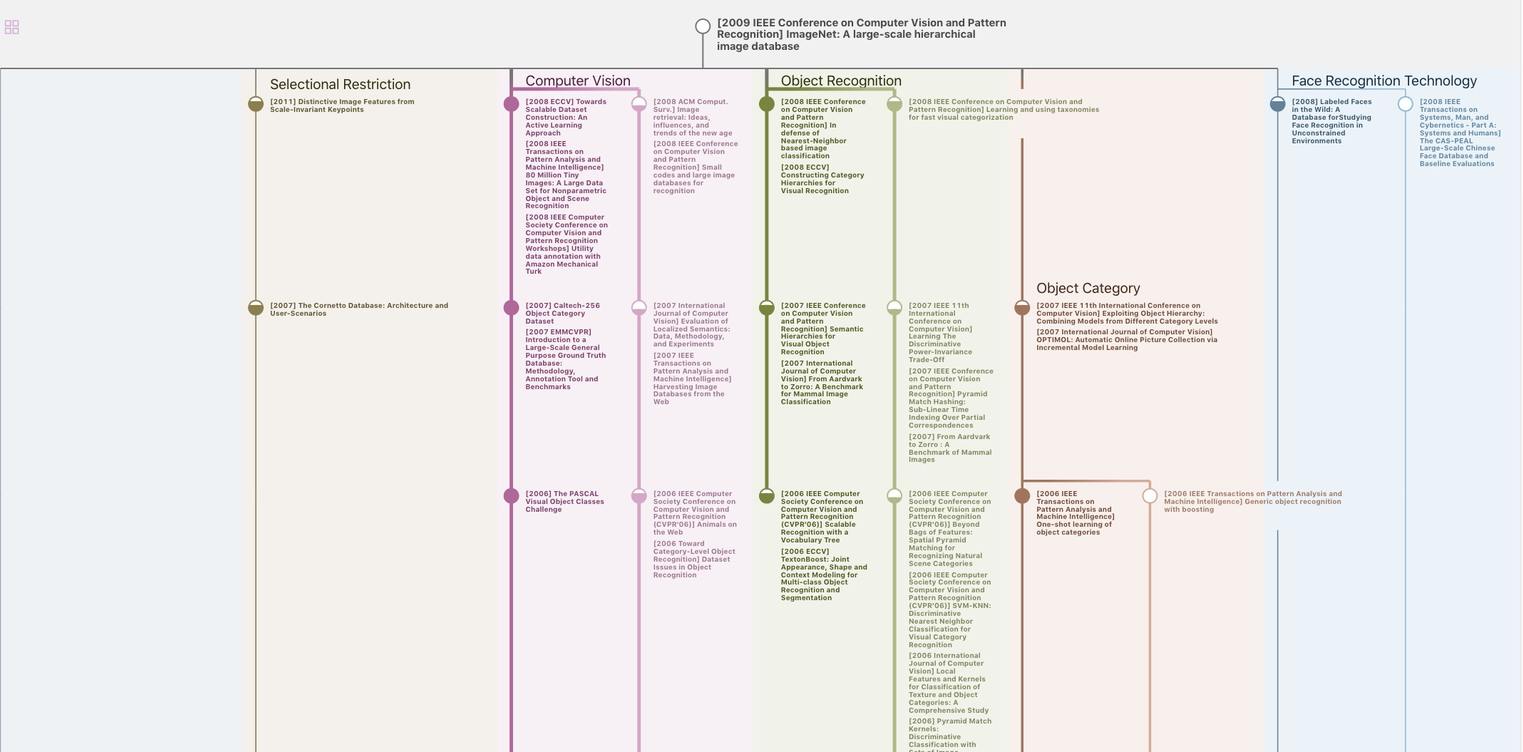
生成溯源树,研究论文发展脉络
Chat Paper
正在生成论文摘要