Bayesian design with sampling windows for complex spatial processes
JOURNAL OF THE ROYAL STATISTICAL SOCIETY SERIES C-APPLIED STATISTICS(2024)
Abstract
Optimal design facilitates intelligent data collection. In this paper, we introduce a fully Bayesian design approach for spatial processes with complex covariance structures, like those typically exhibited in natural ecosystems. Coordinate exchange algorithms are commonly used to find optimal design points. However, collecting data at specific points is often infeasible in practice. Currently, there is no provision to allow for flexibility in the choice of design. Accordingly, we also propose an approach to find Bayesian sampling windows, rather than points, via Gaussian process emulation to identify regions of high design efficiency across a multi-dimensional space. These developments are motivated by two ecological case studies: monitoring water temperature in a river network system in the northwestern United States and monitoring submerged coral reefs off the north-west coast of Australia.
MoreTranslated text
Key words
Gaussian process,generalised linear spatial model,Kullback-Leibler divergence,optimal sampling,stochastic optimisation
AI Read Science
Must-Reading Tree
Example
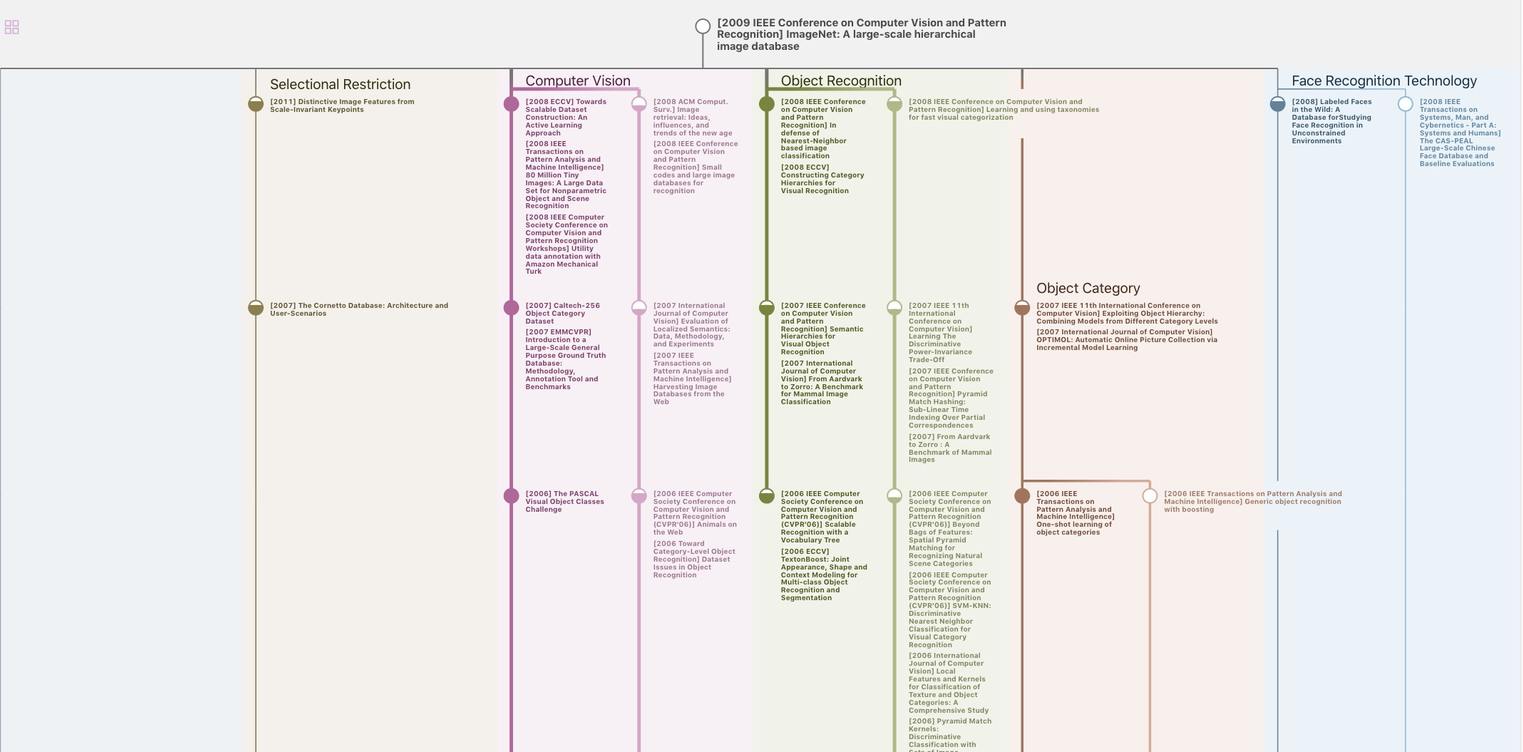
Generate MRT to find the research sequence of this paper
Chat Paper
Summary is being generated by the instructions you defined