Strong Memory Lower Bounds for Learning Natural Models.
Annual Conference on Computational Learning Theory(2022)
摘要
We give lower bounds on the amount of memory required by a one-pass streaming algorithms for solving several natural learning problems. In a setting where examples lie in $\{0,1\}^d$ and the optimal classifier can be encoded using $\kappa$ bits, we show that algorithms which learn to constant error using a near-minimal number of examples, $\tilde O(\kappa)$, must use $\tilde \Omega( d\kappa)$ bits of space. Our space bounds match the dimension of the ambient space of the problem’s natural parametrization, even when it is quadratic in the size of examples and the final classifier. For instance, in the setting of $d$-sparse linear classifiers over degree-2 polynomial features, for which $\kappa=\Theta(d\log d)$, our space lower bound is $\tilde\Omega(d^2)$. Our bounds degrade gracefully with the stream length $N$, generally having the form $\tilde\Omega(d\kappa \cdot \frac{\kappa}{N})$. Bounds of the form $\Omega(d\kappa)$ were known for learning parity and other problems defined over finite fields. Bounds that apply in a narrow range of sample sizes are also known for linear regression. Ours are the first such bounds for problems of the type commonly seen in recent learning applications that apply for for a large range of input sizes.
更多查看译文
关键词
strong memory lower bounds,natural models,learning
AI 理解论文
溯源树
样例
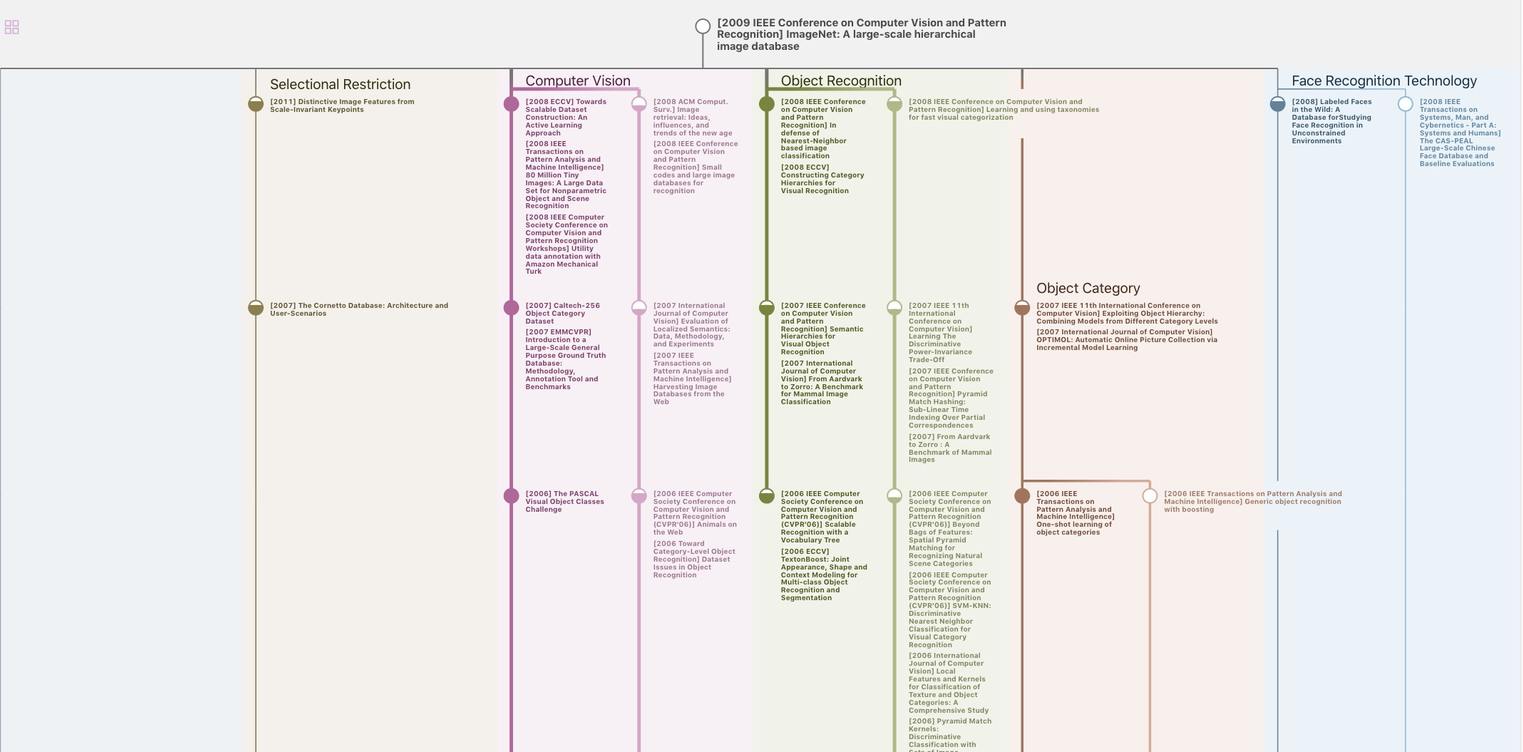
生成溯源树,研究论文发展脉络
Chat Paper
正在生成论文摘要