Arrhythmia classification of 12-lead and reduced-lead electrocardiograms via recurrent networks, scattering, and phase harmonic correlation
PHYSIOLOGICAL MEASUREMENT(2022)
摘要
We describe an automatic classifier of arrhythmias based on 12-lead and reduced-lead electrocardiograms. Our classifier comprises four modules: scattering transform (ST), phase harmonic correlation (PHC), depthwise separable convolutions (DSC), and a long short-term memory (LSTM) network. It is trained on PhysioNet/Computing in Cardiology Challenge 2021 data. The ST captures short-term temporal ECG modulations while the PHC characterizes the phase dependence of coherent ECG components. Both reduce the sampling rate to a few samples per typical heart beat. We pass the output of the ST and PHC to a depthwise-separable convolution layer (DSC) which combines lead responses separately for each ST or PHC coefficient and then combines resulting values across all coefficients. At a deeper level, two LSTM layers integrate local variations of the input over long time scales. We train in an end-to-end fashion as a multilabel classification problem with a normal and 25 arrhythmia classes. Lastly, we use canonical correlation analysis (CCA) for transfer learning from 12-lead ST and PHC representations to reduced-lead ones. After local cross-validation on the public data from the challenge, our team 'BitScattered' achieved the following results: 0.682 +/- 0.0095 for 12-lead; 0.666 +/- 0.0257 for 6-lead; 0.674 +/- 0.0185 for 4-lead; 0.661 +/- 0.0098 for 3-lead; and 0.662 +/- 0.0151 for 2-lead.
更多查看译文
关键词
electrocardiography, scattering transform, phase harmonic correlation, canonical correlation analysis, convolutional neural networks, long short-term memory networks
AI 理解论文
溯源树
样例
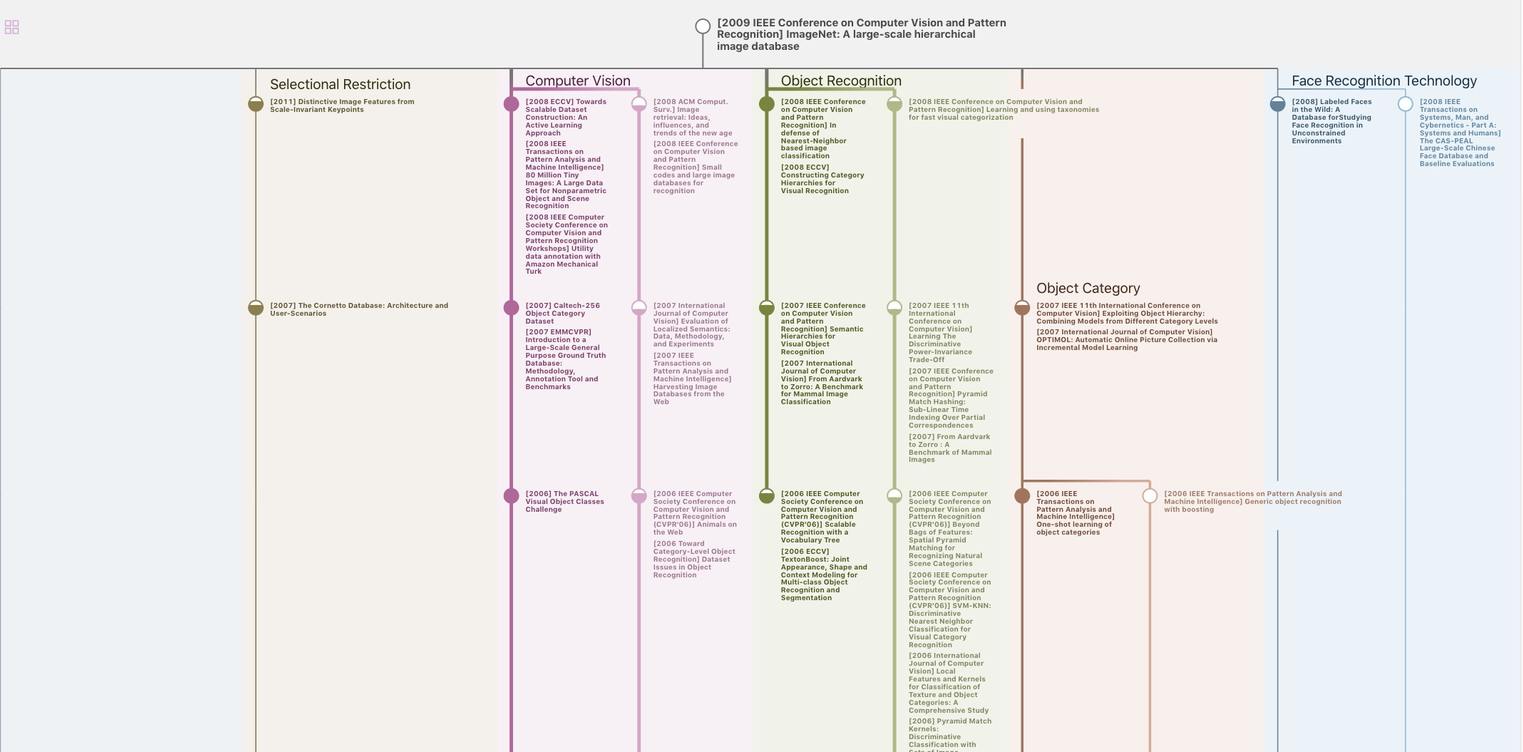
生成溯源树,研究论文发展脉络
Chat Paper
正在生成论文摘要