A Critical Review on the Use (and Misuse) of Differential Privacy in Machine Learning
arxiv(2022)
摘要
We review the use of differential privacy (DP) for privacy protection in machine learning (ML). We show that, driven by the aim of preserving the accuracy of the learned models, DP-based ML implementations are so loose that they do not offer the ex ante privacy guarantees of DP. Instead, what they deliver is basically noise addition similar to the traditional (and often criticized) statistical disclosure control approach. Due to the lack of formal privacy guarantees, the actual level of privacy offered must be experimentally assessed ex post, which is done very seldom. In this respect, we present empirical results showing that standard anti-overfitting techniques in ML can achieve a better utility/privacy/efficiency trade-off than DP.
更多查看译文
关键词
Differential privacy,machine learning,federated learning,data utility
AI 理解论文
溯源树
样例
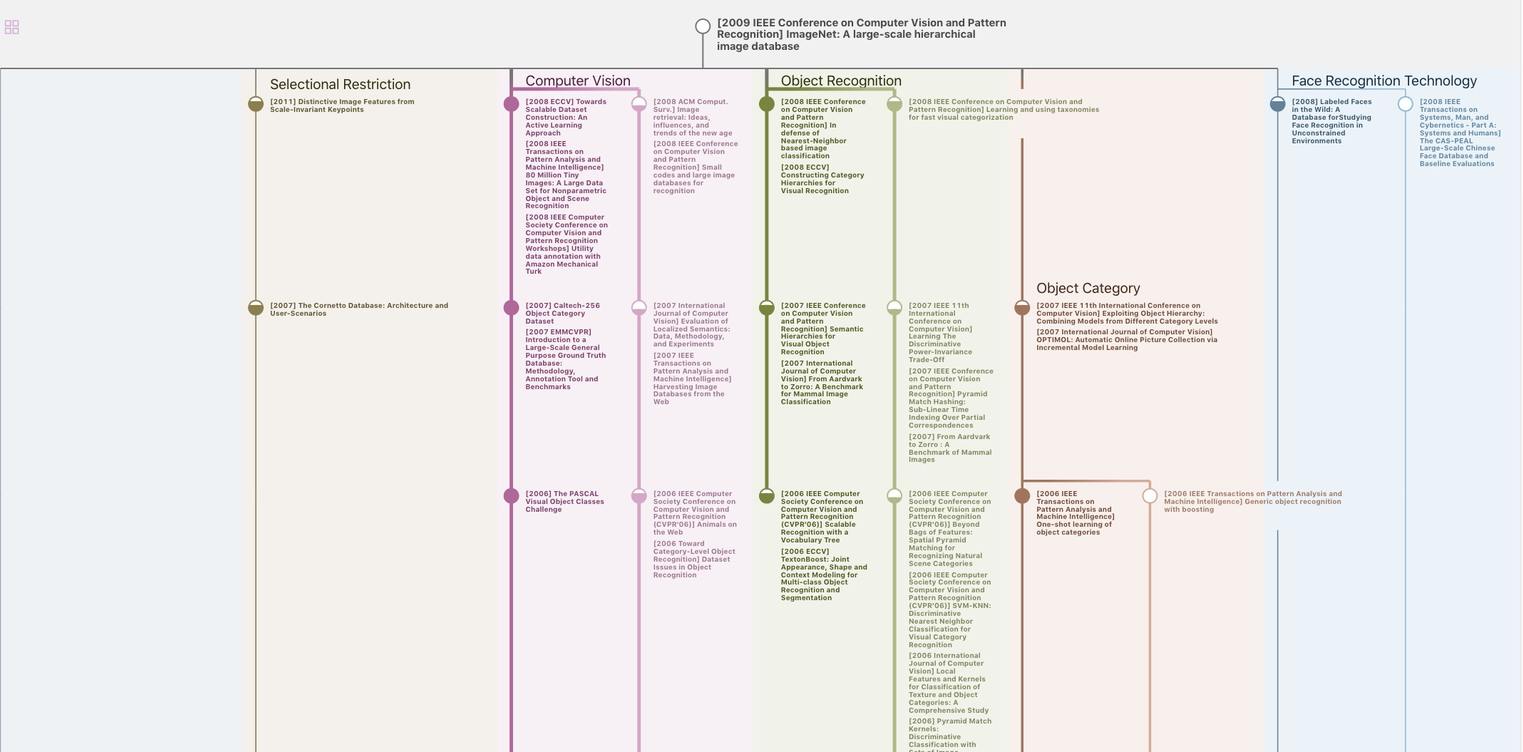
生成溯源树,研究论文发展脉络
Chat Paper
正在生成论文摘要