AGN X-ray spectroscopy with neural networks
MONTHLY NOTICES OF THE ROYAL ASTRONOMICAL SOCIETY(2022)
摘要
We explore the possibility of using machine learning to estimate physical parameters directly from active galactic nucleus (AGN) X-ray spectra without needing computationally expensive spectral fitting. Specifically, we consider survey quality data, rather than long pointed observations, to ensure that this approach works in the regime where it is most likely to be applied. We simulate Athena Wide Field Imager spectra of AGN with warm absorbers, and train simple neural networks to estimate the ionization and column density of the absorbers. We find that this approach can give comparable accuracy to spectral fitting, without the risk of outliers caused by the fit sticking in a false minimum, and with an improvement of around three orders of magnitude in speed. We also demonstrate that using principal component analysis to reduce the dimensionality of the data prior to inputting it into the neural net can significantly increase the accuracy of the parameter estimation for negligible computational cost, while also allowing a simpler network architecture to be used.
更多查看译文
关键词
accretion, accretion discs, black hole physics, techniques: spectroscopic, galaxies: active
AI 理解论文
溯源树
样例
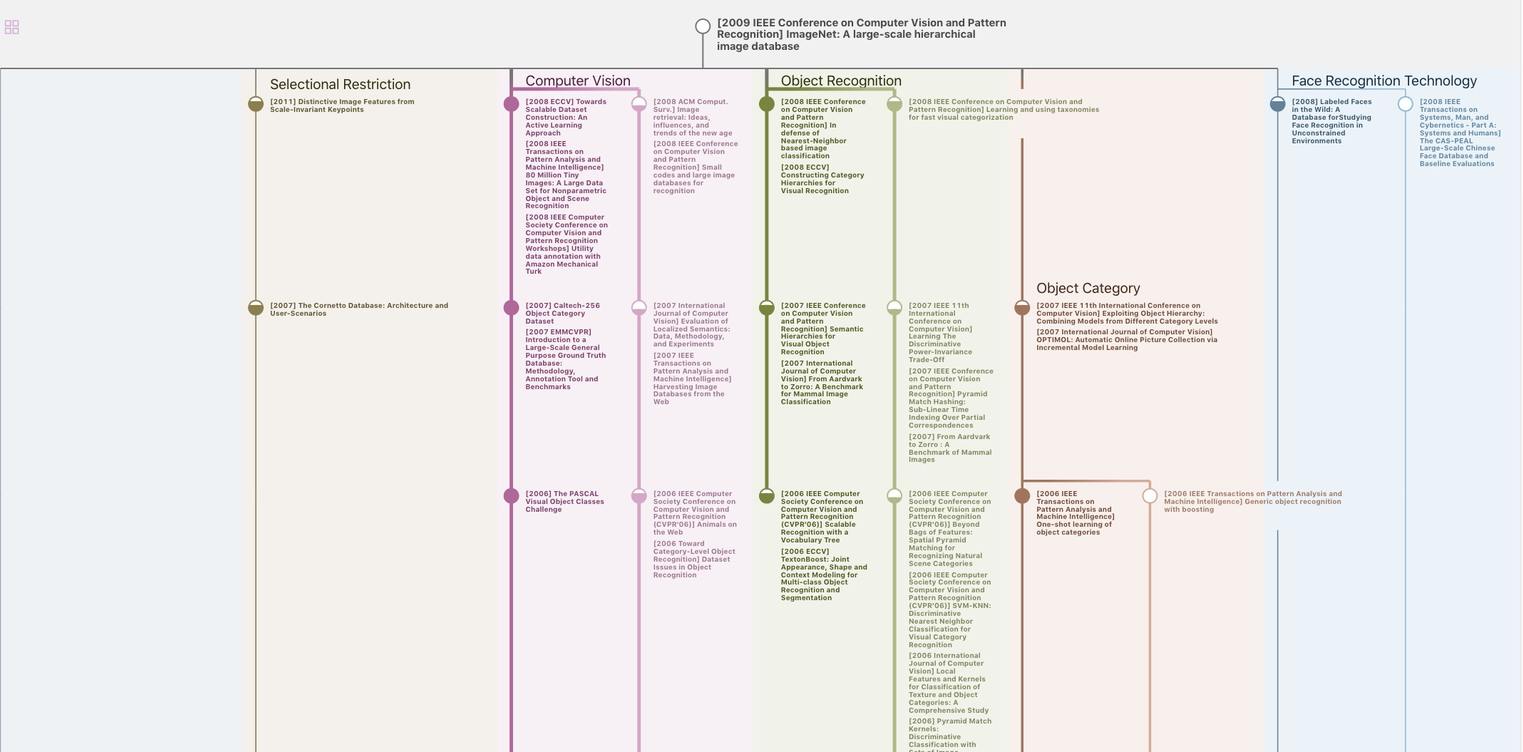
生成溯源树,研究论文发展脉络
Chat Paper
正在生成论文摘要