Incremental Recursive Ranking Grouping for Large-Scale Global Optimization
IEEE TRANSACTIONS ON EVOLUTIONARY COMPUTATION(2023)
摘要
Real-world optimization problems may have a different underlying structure. In black-box optimization, the dependencies between decision variables remain unknown. However, some techniques can discover such interactions accurately. In large-scale global optimization (LSGO), problems are high dimensional. It was shown effective to decompose LSGO problems into subproblems and optimize them separately. The effectiveness of such approaches may be highly dependent on the accuracy of problem decomposition. Many state-of-the-art decomposition strategies are derived from differential grouping (DG). However, if a given problem consists of nonadditively separable subproblems, DG-based strategies may discover many nonexisting interactions. On the other hand, monotonicity checking strategies proposed so far do not report nonexisting interactions for any separable subproblems but may miss discovering many of the existing ones. Therefore, we propose incremental recursive ranking grouping (IRRG) that suffers from none of these flaws. IRRG consumes more fitness function evaluations than the recent DG-based propositions, e.g., recursive DG 3 (RDG3). Nevertheless, the effectiveness of the considered cooperative co-evolution frameworks after embedding IRRG or RDG3 was similar for problems with additively separable subproblems that are suitable for RDG3. After replacing the additive separability with nonadditive, embedding IRRG leads to results of significantly higher quality.
更多查看译文
关键词
Large-scale global optimization (LSGO),monotonicity checking,nonadditive separability,problem decomposition
AI 理解论文
溯源树
样例
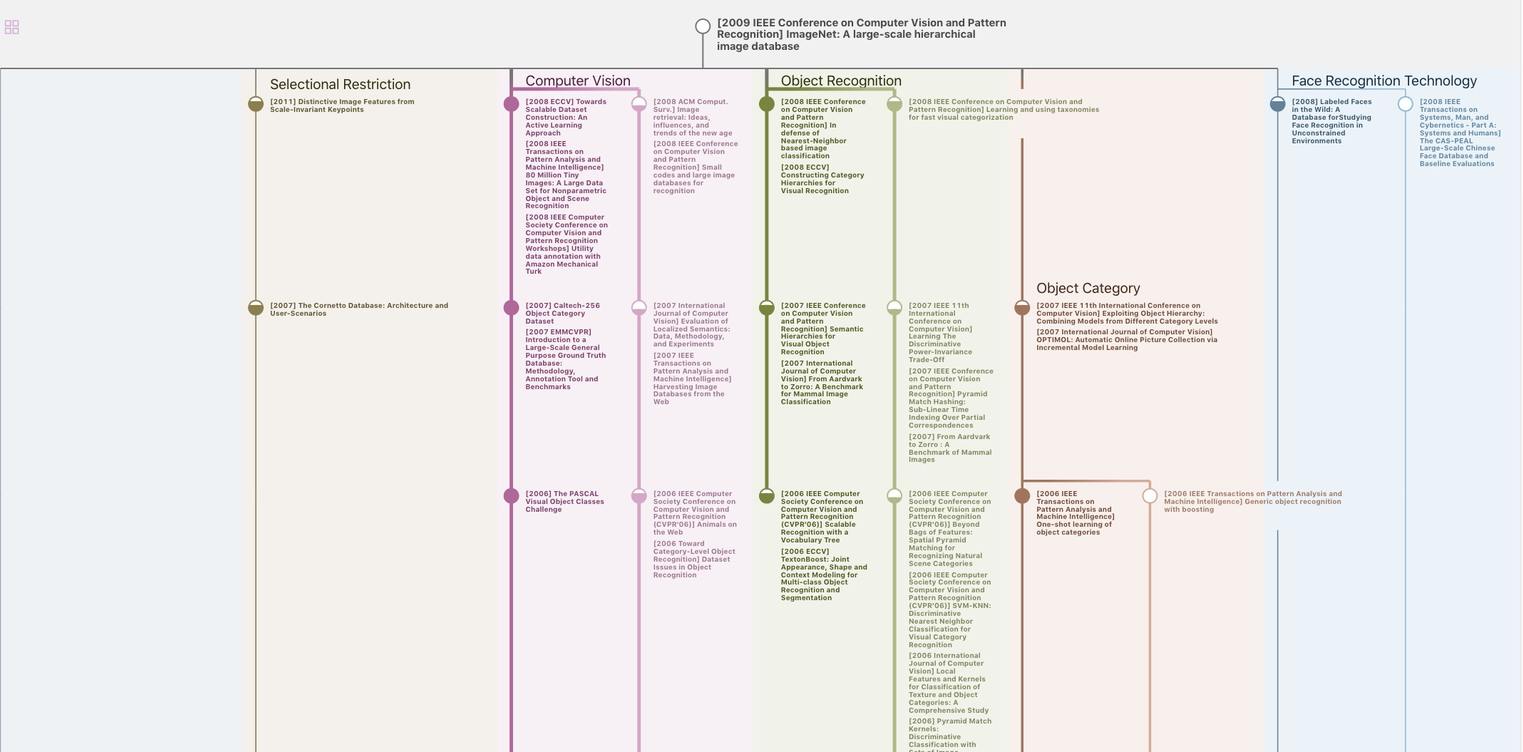
生成溯源树,研究论文发展脉络
Chat Paper
正在生成论文摘要