Meta-Learning Parameterized Skills
arxiv(2023)
摘要
We propose a novel parameterized skill-learning algorithm that aims to learn transferable parameterized skills and synthesize them into a new action space that supports efficient learning in long-horizon tasks. We propose to leverage off-policy Meta-RL combined with a trajectory-centric smoothness term to learn a set of parameterized skills. Our agent can use these learned skills to construct a three-level hierarchical framework that models a Temporally-extended Parameterized Action Markov Decision Process. We empirically demonstrate that the proposed algorithms enable an agent to solve a set of difficult long-horizon (obstacle-course and robot manipulation) tasks.
更多查看译文
关键词
skills,meta-learning
AI 理解论文
溯源树
样例
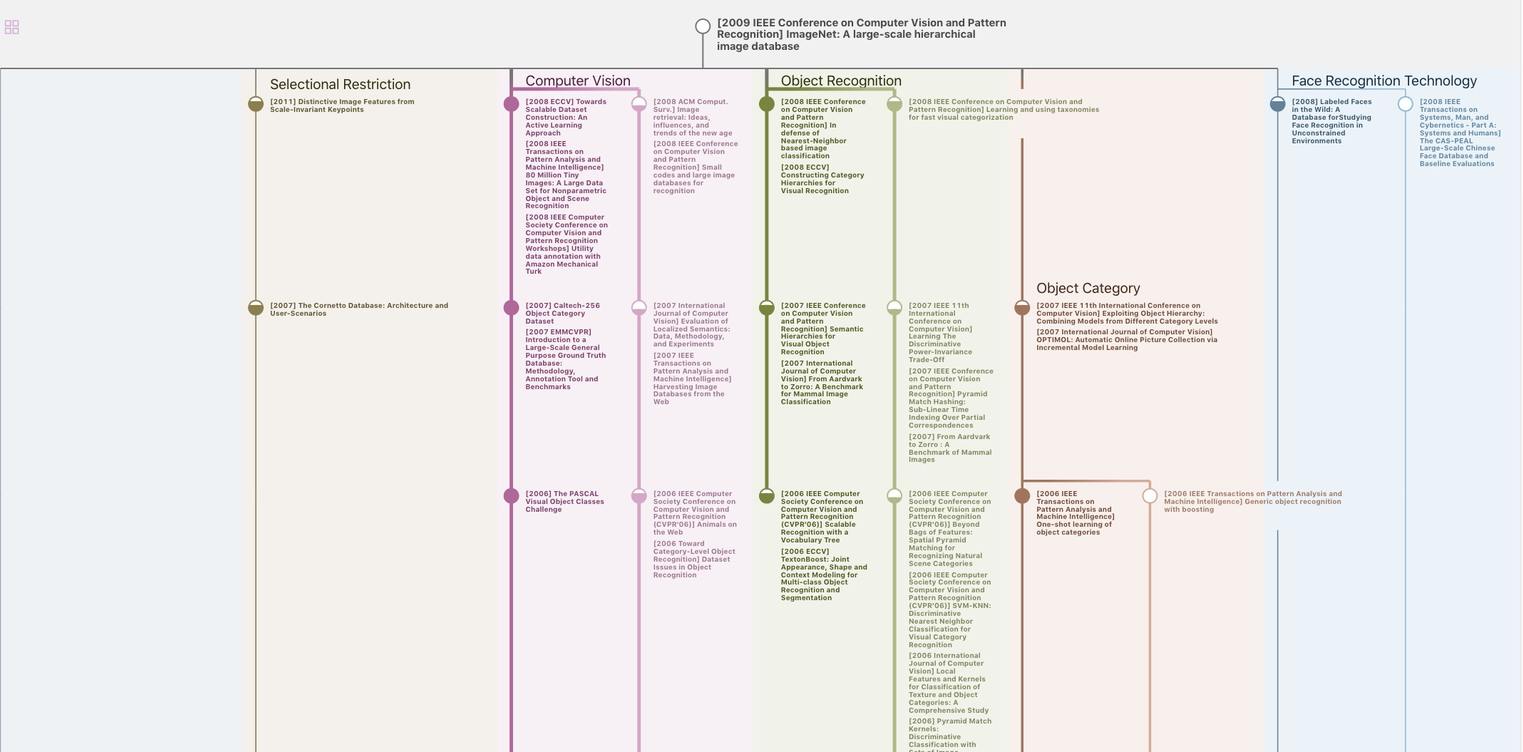
生成溯源树,研究论文发展脉络
Chat Paper
正在生成论文摘要