Personalized auto-segmentation for magnetic resonance imaging-guided adaptive radiotherapy of prostate cancer
MEDICAL PHYSICS(2022)
摘要
Purpose Fast and accurate delineation of organs on treatment-fraction images is critical in magnetic resonance imaging-guided adaptive radiotherapy (MRIgART). This study proposes a personalized auto-segmentation (AS) framework to assist online delineation of prostate cancer using MRIgART. Methods Image data from 26 patients diagnosed with prostate cancer and treated using hypofractionated MRIgART (5 fractions per patient) were collected retrospectively. Daily pretreatment T2-weighted MRI was performed using a 1.5-T MRI system integrated into a Unity MR-linac. First-fraction image and contour data from 16 patients (80 image-sets) were used to train the population AS model, and the remaining 10 patients composed the test set. The proposed personalized AS framework contained two main steps. First, a convolutional neural network was employed to train the population model using the training set. Second, for each test patient, the population model was progressively fine-tuned with manually checked delineations of the patient's current and previous fractions to obtain a personalized model that was applied to the next fraction. Results Compared with the population model, the personalized models substantially improved the mean Dice similarity coefficient from 0.79 to 0.93 for the prostate clinical target volume (CTV), 0.91 to 0.97 for the bladder, 0.82 to 0.92 for the rectum, and 0.91 to 0.93 for the femoral heads, respectively. Conclusions The proposed method can achieve accurate segmentation and potentially shorten the overall online delineation time of MRIgART.
更多查看译文
关键词
magnetic resonance imaging-guided adaptive radiotherapy, personalized segmentation, prostate cancer
AI 理解论文
溯源树
样例
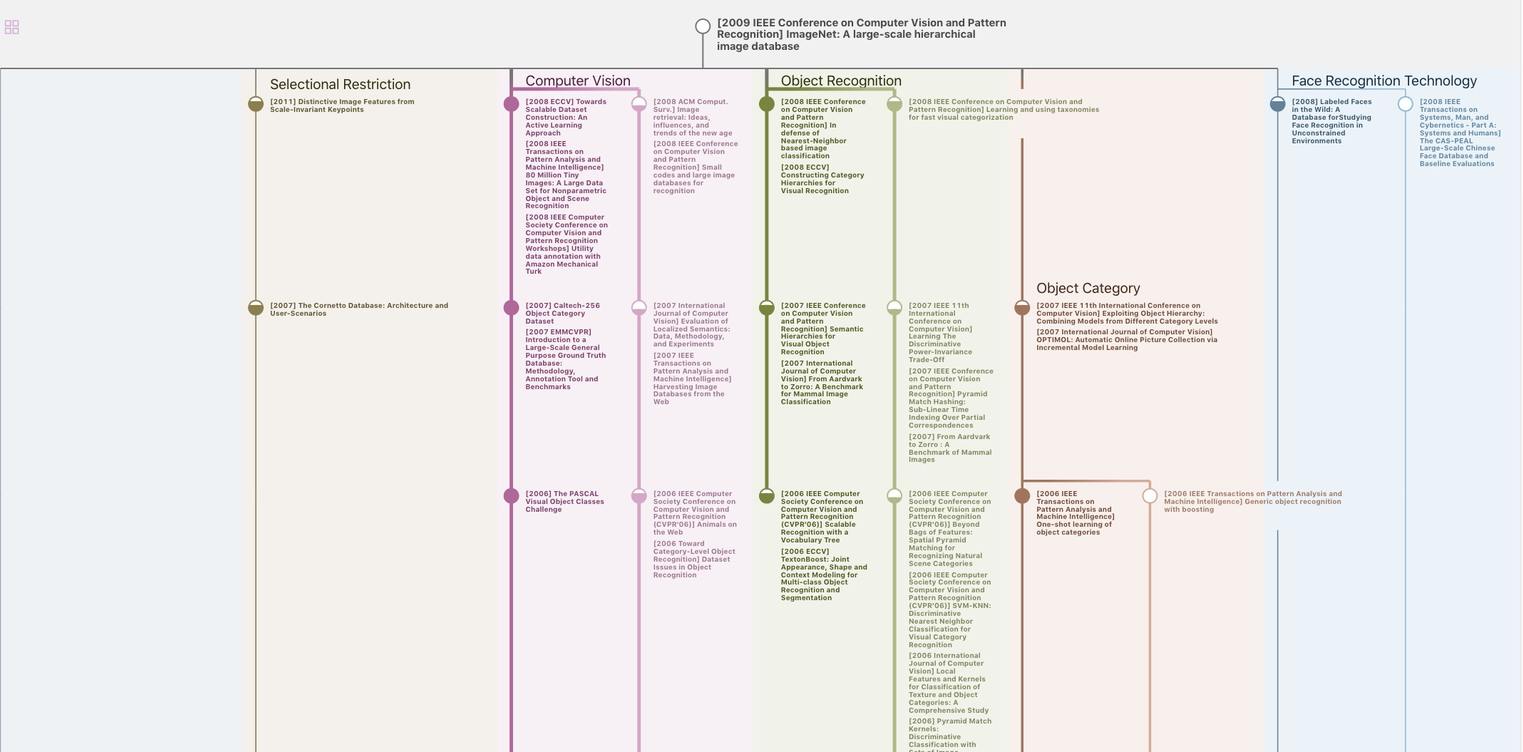
生成溯源树,研究论文发展脉络
Chat Paper
正在生成论文摘要