Deep learning for potential field edge detection
Chinese Journal of Geophysics(2022)
摘要
Edge detection is a fundamental technique in the potential field data processing. The current methodology for edge detection belongs to unsupervised machine operation, whose accuracy largely depends on the spatial distribution of geological bodies, especially for deep complex geological anomaly. In order to further improve the accuracy of edge detection, a dense skip connection network (PFD-Net) with multiple scale features and a global attention mechanism is proposed for potential field edge detection, which is inspired by the excellent nonlinear mapping ability of deep learning and the advantages of supervised learning. First, the improved U-net is employed as the backbone network to obtain the featured information of the potential field edge, followed by the dense skip connection that is applied among the nested standard convolution modules, reducing the semantic gap from the encoding stage to the decoding stage and preventing phenomenon such as gradient disappearance during training. The global attention mechanism module is then applied to integrate multiple scale high- and low-level feature information to further strengthen the global and detailed position of the edge. In this paper, a fast forward algorithm of potential field anomaly spatial domain based on grid point geometrical framework is proposed, thus constructing a sufficient and diverse sample data set. The network structure of PFD-Net is built, where the input layer is the potential field anomaly, and the output layer is the projection edge of the geological anomaly body on the horizontal plane, thereby allowing the training and parameter optimization to perform based on PFD-Net, as well as the edge detection. As shown in the tests of the four sets of models, the edge information of anomalies can be accurately distinguished via PFD-Net while the quality of prediction results for the noise-contaminated data is not influenced, demonstrating the strong generalization and robustness of the network. Finally, the method in this paper is applied to the aerial magnetic survey data of the western section of a railway tunnel in southeastern Tibet, and a good edge detection result and more interpretable information are obtained.
更多查看译文
关键词
Potential field edge detection, Multiple scale, Attention mechanism, Dense skip connection
AI 理解论文
溯源树
样例
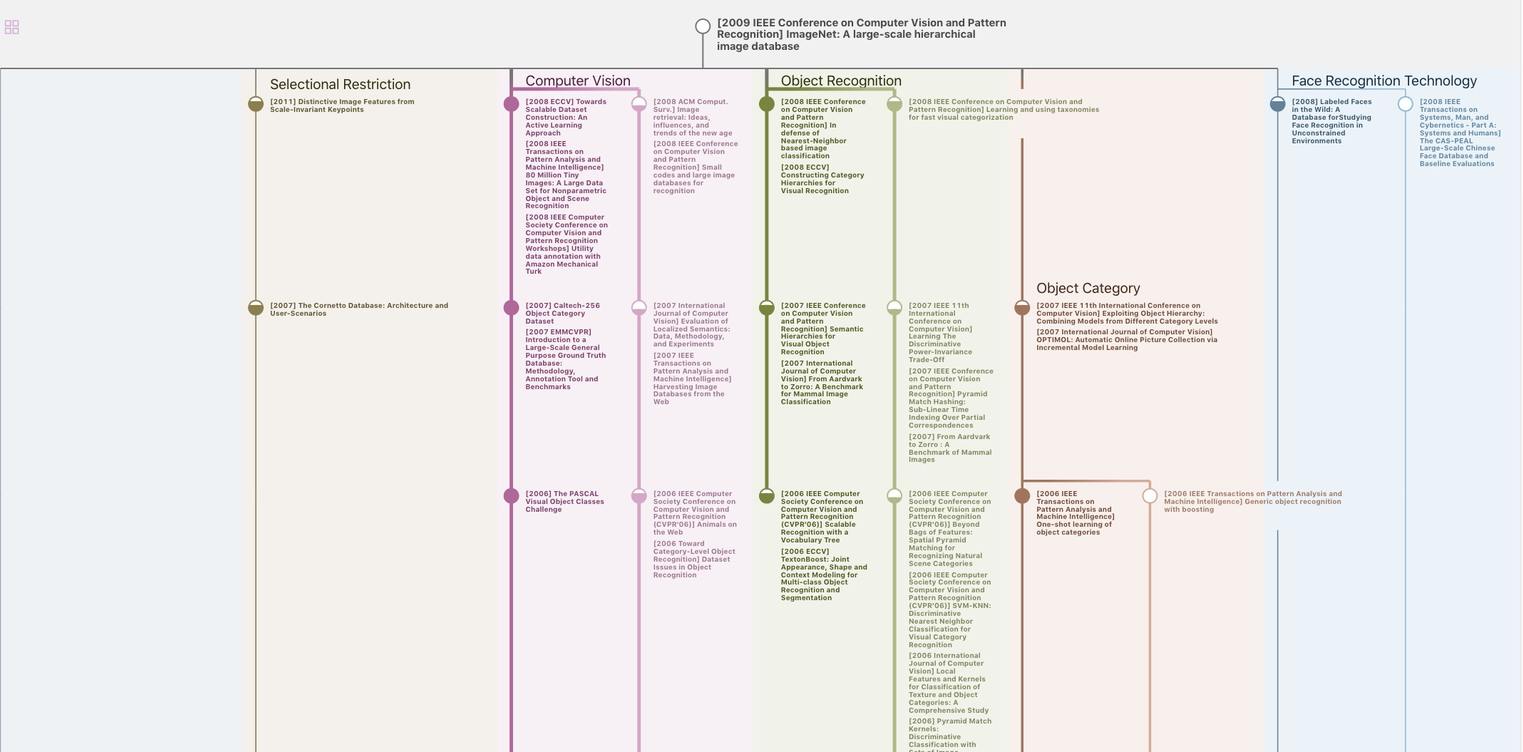
生成溯源树,研究论文发展脉络
Chat Paper
正在生成论文摘要