GeoInFuse - A data-driven information fusion for intra-urban form classification in data-scarce heterogeneous cities
Cities(2022)
摘要
Understanding the variability in intra-urban forms is essential for protecting future-proofing cities from climate volatilities. However, the classification of urban forms is costly due to its reliance on high-resolution datasets, limited in the Global South's low-income cities. Additionally, the current classifiers are constrained in characterizing urban forms in heterogeneous cities where the built environment has an isomorphic footprint but vary in urban canopy parameters. Existing methodologies have constraints on using unstructured low-resolution datasets like images and portable document formats to classify intra-urban forms. This study breaks ground by forwarding a novel end-to-end multi-channel supervised data fusion framework, GeoInFuse, to classify different urban form typologies in heterogeneous hyper-dense cities. It uses a state-of-the-art deep learning network on open-source image-based datasets to generate urban form classification. Three datasets (two for ground truth and one containing multi-dimensional urban canopy parameters) are used to demonstrate the framework. The parameters as channels were fused to generate urban form classes. Results show that the GeoInFuse could extract the heterogeneous urban form classes with 74.9.
更多查看译文
关键词
Information fusion,Convolution,Urban form classification,Multi-channel,Data-scare,Heterogeneity
AI 理解论文
溯源树
样例
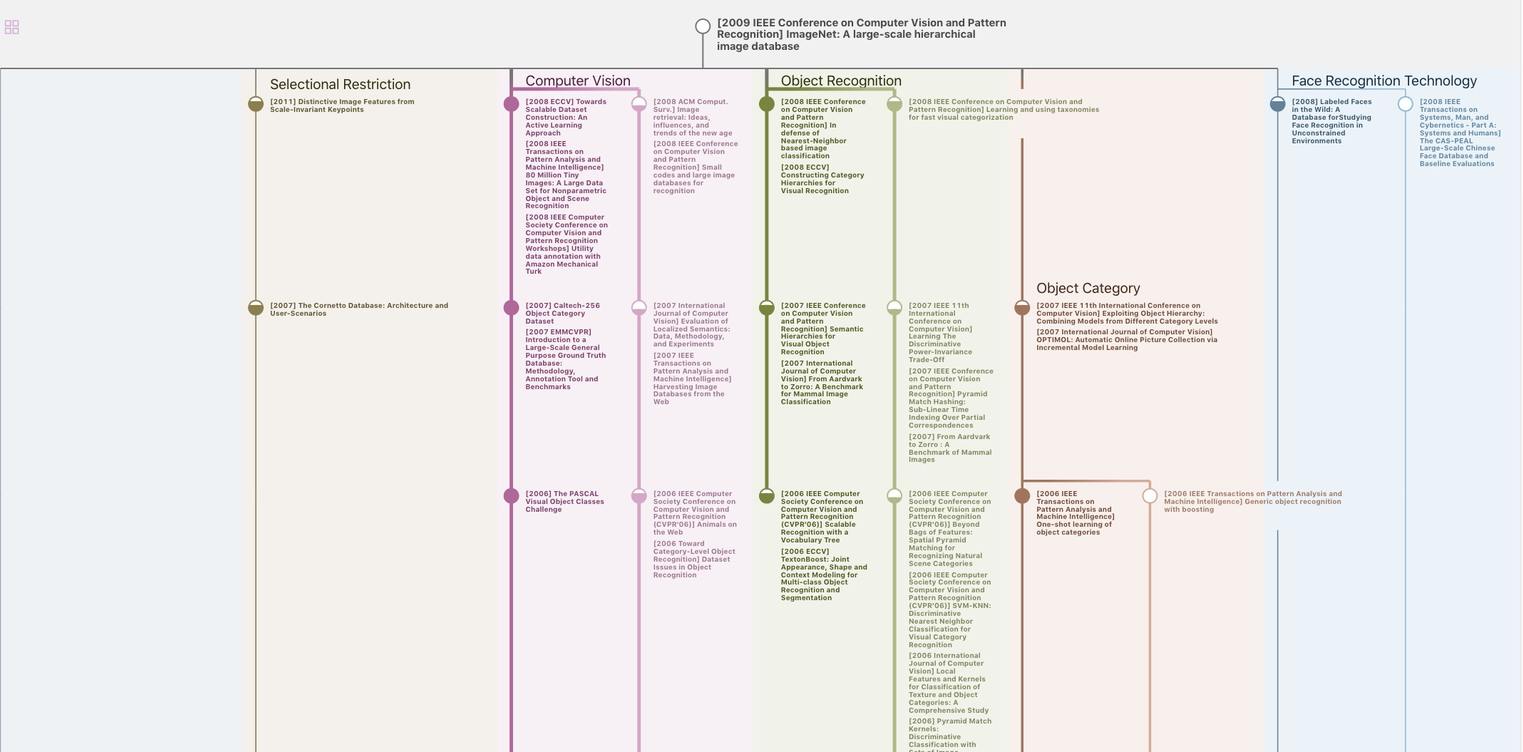
生成溯源树,研究论文发展脉络
Chat Paper
正在生成论文摘要