Decentralized Aggregation for Energy-Efficient Federated Learning via D2D Communications
arxiv(2023)
摘要
Federated learning (FL) has emerged as a distributed machine learning (ML) technique to train models without sharing users’ private data. In this paper, we introduce a decentralized FL scheme that is called federated learning empowered overlapped clustering for decentralized aggregation (FL-EOCD). The introduced FL-EOCD leverages device-to-device (D2D) communications and overlapped clustering to enable decentralized aggregation, where a cluster is defined as a coverage zone of a typical device. The devices located on the overlapped clusters are called bridge devices (BDs). In the proposed FL-EOCD scheme, a clustering topology is envisioned where clusters are connected through BDs, so as the aggregated models of each cluster is disseminated to the other clusters in a decentralized manner without the need for a global aggregator or an additional hop of transmission. To evaluate our proposed FL-EOCD scheme as opposed to baseline FL schemes, we consider minimizing the overall energy-consumption of devices while maintaining the convergence rate of FL subject to its time constraint. To this end, a joint optimization problem, considering scheduling the local devices/BDs to the CHs and computation frequency allocation, is formulated, where an iterative solution to this joint problem is devised. Extensive simulations are conducted to verify the effectiveness of the proposed FL-EOCD algorithm over FL conventional schemes in terms of energy consumption, latency, and convergence rate.
更多查看译文
关键词
federated,communications,energy-efficient
AI 理解论文
溯源树
样例
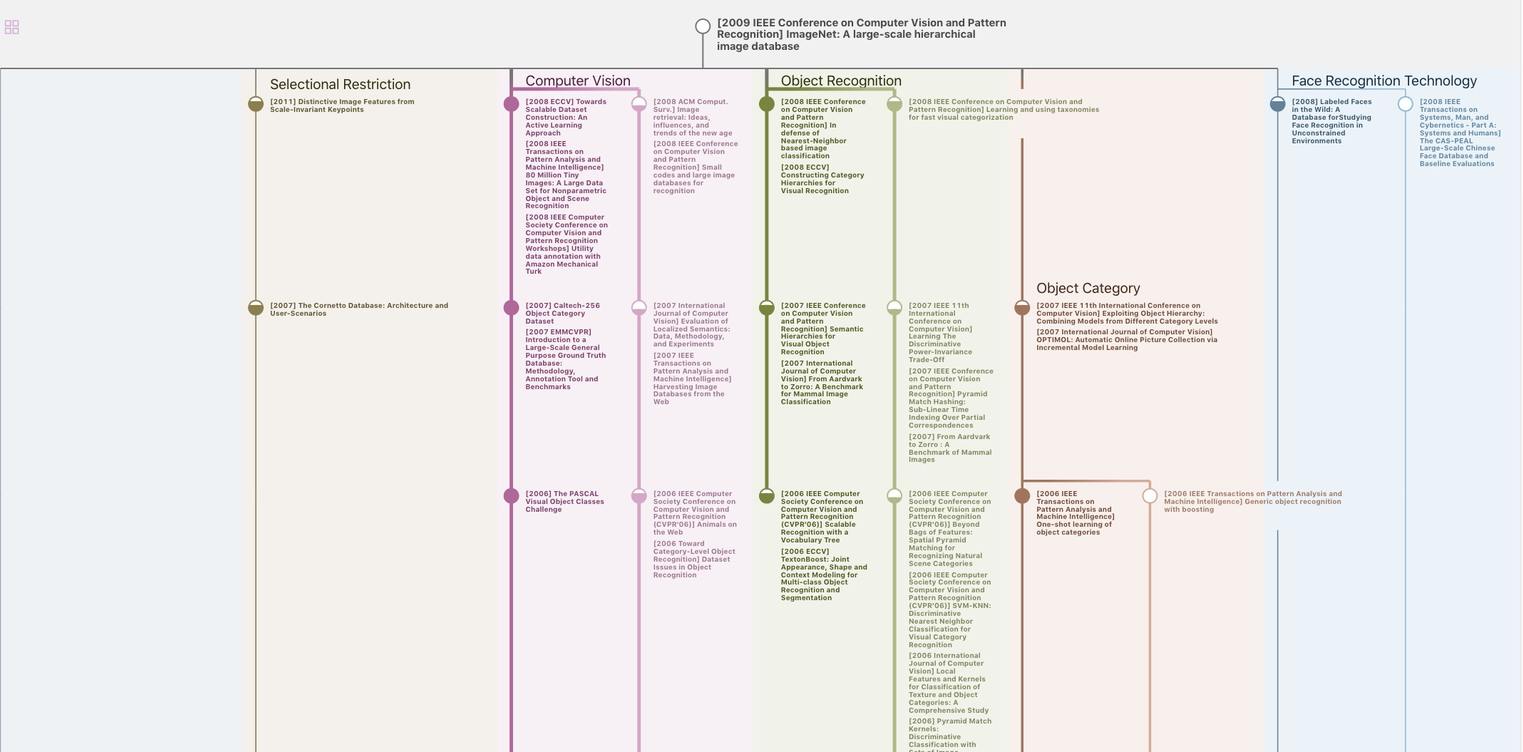
生成溯源树,研究论文发展脉络
Chat Paper
正在生成论文摘要