Dissecting Tensor Cores via Microbenchmarks: Latency, Throughput and Numeric Behaviors
IEEE Transactions on Parallel and Distributed Systems(2023)
摘要
Tensor Cores have been an important unit to accelerate Fused Matrix Multiplication Accumulation (MMA) in all NVIDIA GPUs since Volta Architecture. To program Tensor Cores, users have to use either legacy wmma APIs or current mma APIs. Legacy wmma APIs are more easy-to-use but can only exploit limited features and power of Tensor Cores. Specifically, wmma APIs support fewer operand shapes and can not leverage the new sparse matrix multiplication feature of the newest Ampere Tensor Cores. However, the performance of current programming interface has not been well explored. Furthermore, the computation numeric behaviors of low-precision floating points (TF32, BF16, and FP16) supported by the newest Ampere Tensor Cores are also mysterious. In this paper, we explore the throughput and latency of current programming APIs. We also intuitively study the numeric behaviors of Tensor Cores MMA and profile the intermediate operations including multiplication, addition of inner product, and accumulation. All codes used in this work can be found in https://github.com/sunlex0717/DissectingTensorCores.
更多查看译文
关键词
GPU,tensor cores,numeric profiling,ampere,turing,microbenchmark
AI 理解论文
溯源树
样例
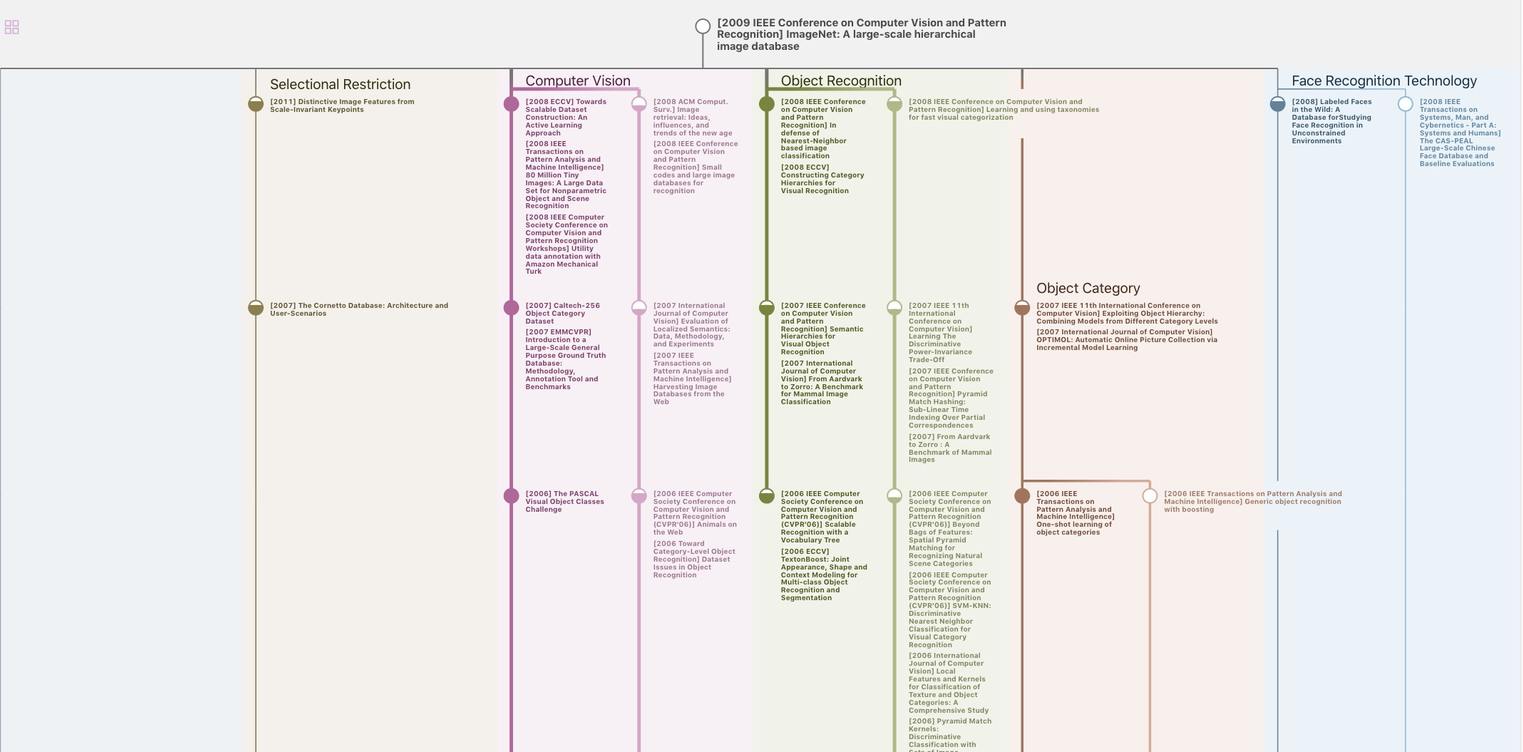
生成溯源树,研究论文发展脉络
Chat Paper
正在生成论文摘要