Deep learning models of the discrete component of the Galactic interstellar ?-ray emission
arxiv(2023)
摘要
A significant pointlike component from the small-scale (or discrete) structure in the H2 interstellar gas might be present in the Fermi-LAT data, but modeling this emission relies on observations of rare gas tracers only available in limited regions of the sky. Identifying this contribution is important to discriminate 7-ray point sources from interstellar gas, and to better characterize extended 7-ray sources. We design and train convolutional neural networks to predict this emission where observations of these rare tracers do not exist, and discuss the impact of this component on the analysis of the Fermi-LAT data. In particular, we evaluate prospects to exploit this methodology in the characterization of the Fermi-LAT Galactic center excess through accurate modeling of pointlike structures in the data to help distinguish between a pointlike or smooth nature for the excess. We show that deep learning may be effectively employed to model the 7-ray emission traced by these rare H2 proxies within statistical significance in data-rich regions, supporting prospects to employ these methods in yet unobserved regions.
更多查看译文
AI 理解论文
溯源树
样例
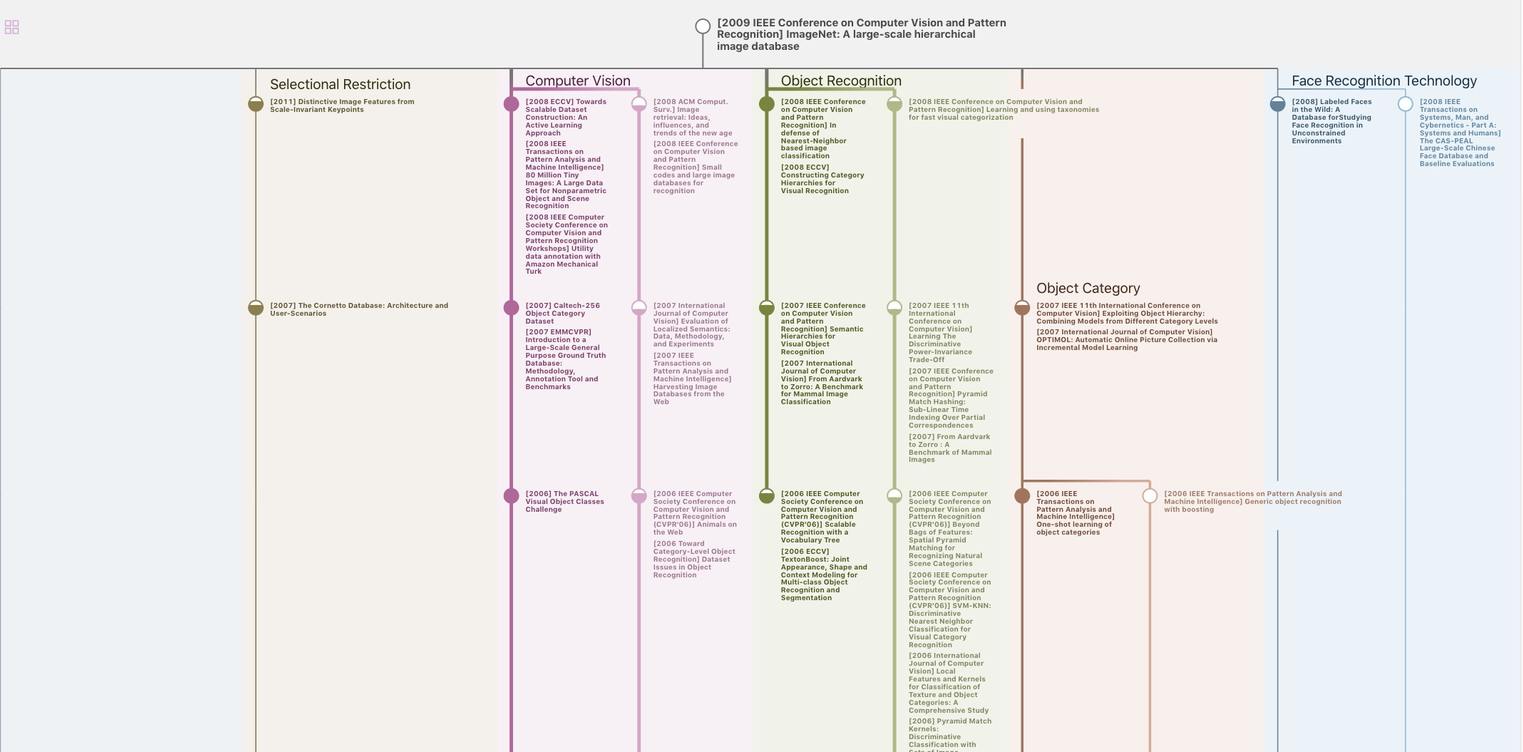
生成溯源树,研究论文发展脉络
Chat Paper
正在生成论文摘要