Machine learning reveals salivary glycopatterns as potential biomarkers for the diagnosis and prognosis of papillary thyroid cancer
International Journal of Biological Macromolecules(2022)
摘要
The diagnosis of thyroid cancer, especially papillary thyroid cancer (PTC), is increasing rapidly worldwide. In this study, we aimed to study the glycosylation of salivary proteins associated with PTC and assess the likelihood that salivary glycopatterns may be a potential biomarker of PTC diagnosis. Firstly, 22 benign thyroid nodule (BTN) samples, 27 PTC samples, and 30 healthy volunteers (HV) samples were collected to probe the difference of salivary glycopatterns associated with PTC using lectin microarrays. Then, five machine learning models including K-Nearest Neighbor (KNN), Multilayer Perceptron (MLP), Logistic Regression (LR), Random Forest (RF), and Support Vector Machine (SVM) were established to distinguish HV, BTN and PTC based on the changes of salivary glycopatterns. As a result, SVM had the best diagnostic effect with an accuracy rate of 92 % in testing set. Besides, lectin microarrays were used to explore the differences in salivary glycopatterns of 26 paired salivary samples of PTC patients before and after operation in order to probe into salivary glycopatterns as potential biomarkers for prognosis of PTC patients. The results showed that the levels of salivary glycopatterns recognized by 6 different lectins in patients after the operation almost convergenced with HVs. This study could help to screen and assess patients with PTC and their prognosis based on precise changes of salivary glycopatterns.
更多查看译文
关键词
Salivary glycopatterns,Papillary thyroid cancer,Machine learning
AI 理解论文
溯源树
样例
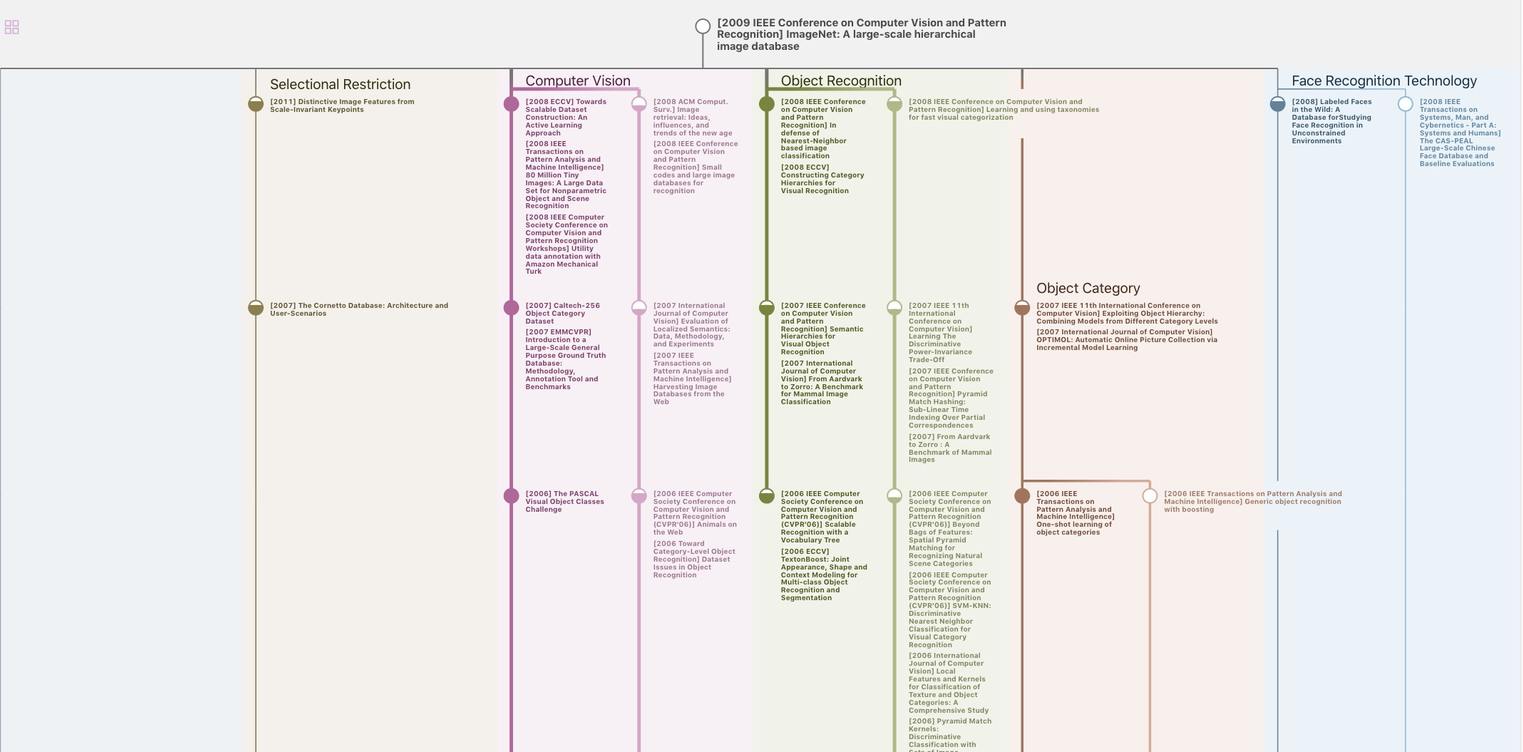
生成溯源树,研究论文发展脉络
Chat Paper
正在生成论文摘要