Spatio-temporal feature extraction in sensory electroneurographic signals
PHILOSOPHICAL TRANSACTIONS OF THE ROYAL SOCIETY A-MATHEMATICAL PHYSICAL AND ENGINEERING SCIENCES(2022)
Abstract
The recording and analysis of peripheral neural signal can provide insight for various prosthetic and bioelectronics medicine applications. However, there are few studies that investigate how informative features can be extracted from population activity electroneurographic (ENG) signals. In this study, five feature extraction frameworks were implemented on sensory ENG datasets and their classification performance was compared. The datasets were collected in acute rat experiments where multi-channel nerve cuffs recorded from the sciatic nerve in response to proprioceptive stimulation of the hindlimb. A novel feature extraction framework, which incorporates spatio-temporal focus and dynamic time warping, achieved classification accuracies above 90% while keeping a low computational cost. This framework outperformed the remaining frameworks tested in this study and has improved the discrimination accuracy of the sensory signals. Thus, this study has extended the tools available to extract features from sensory population activity ENG signals.This article is part of the theme issue 'Advanced neurotechnologies: translating innovation for health and well-being'.
MoreTranslated text
Key words
peripheral nerve recording, classification of sensory signals
AI Read Science
Must-Reading Tree
Example
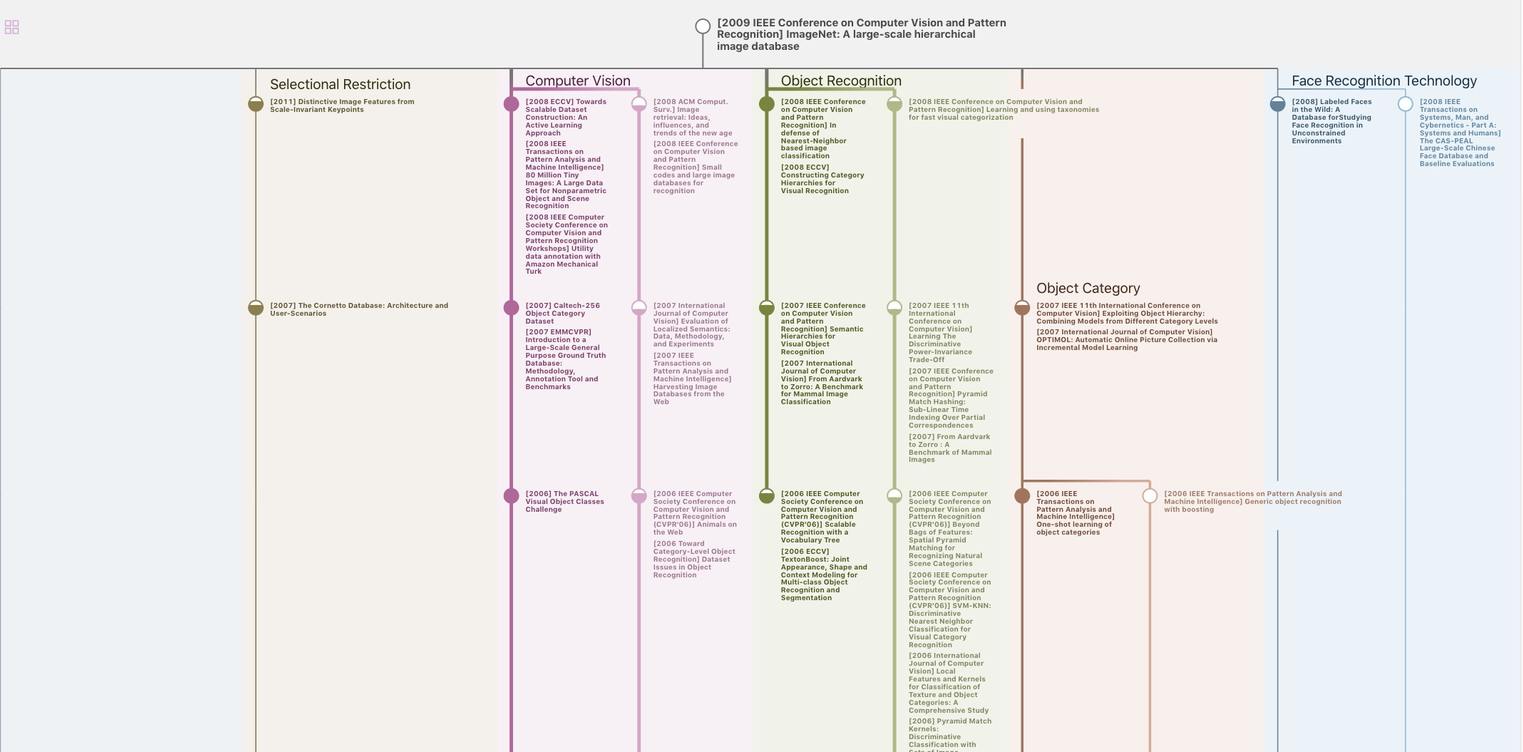
Generate MRT to find the research sequence of this paper
Chat Paper
Summary is being generated by the instructions you defined