When Personalization Harms: Reconsidering the Use of Group Attributes in Prediction
arxiv(2023)
摘要
Machine learning models are often personalized with categorical attributes that are protected, sensitive, self-reported, or costly to acquire. In this work, we show models that are personalized with group attributes can reduce performance at a group level. We propose formal conditions to ensure the "fair use" of group attributes in prediction tasks by training one additional model -- i.e., collective preference guarantees to ensure that each group who provides personal data will receive a tailored gain in performance in return. We present sufficient conditions to ensure fair use in empirical risk minimization and characterize failure modes that lead to fair use violations due to standard practices in model development and deployment. We present a comprehensive empirical study of fair use in clinical prediction tasks. Our results demonstrate the prevalence of fair use violations in practice and illustrate simple interventions to mitigate their harm.
更多查看译文
关键词
personalization harms,group attributes,prediction
AI 理解论文
溯源树
样例
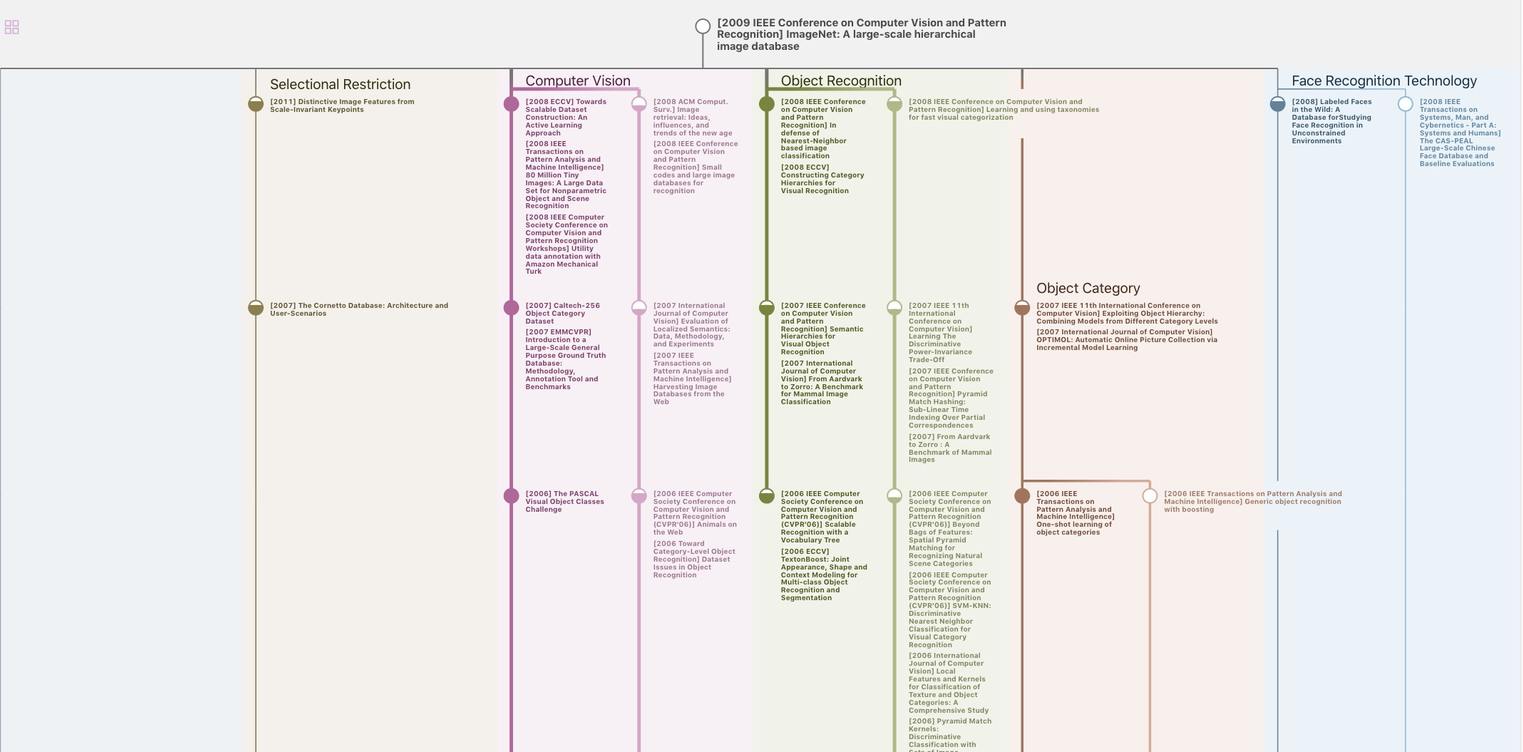
生成溯源树,研究论文发展脉络
Chat Paper
正在生成论文摘要