Nerfels: Renderable Neural Codes for Improved Camera Pose Estimation
IEEE Conference on Computer Vision and Pattern Recognition(2022)
Abstract
This paper presents a framework that combines traditional keypoint-based camera pose optimization with an invertible neural rendering mechanism. Our proposed 3D scene representation, Nerfels, is locally dense yet globally sparse. As opposed to existing invertible neural rendering systems which overfit a model to the entire scene, we adopt a feature-driven approach for representing scene-agnostic, local 3D patches with renderable codes. By modelling a scene only where local features are detected, our framework effectively generalizes to unseen local regions in the scene via an optimizable code conditioning mechanism in the neural renderer, all while maintaining the low memory footprint of a sparse 3D map representation. Our model can be incorporated to existing state-of-the-art hand-crafted and learned local feature pose estimators, yielding improved performance when evaluating on ScanNet for wide camera baseline scenarios.
MoreTranslated text
Key words
neural renderer,sparse 3D map representation,existing state-of-the-art hand-crafted,wide camera baseline scenarios,Nerfels,renderable neural codes,improved camera pose estimation,traditional keypoint-based camera,invertible neural rendering mechanism,existing invertible neural rendering systems,entire scene,feature-driven approach,renderable codes,local feature,unseen local regions,optimizable code conditioning mechanism
AI Read Science
Must-Reading Tree
Example
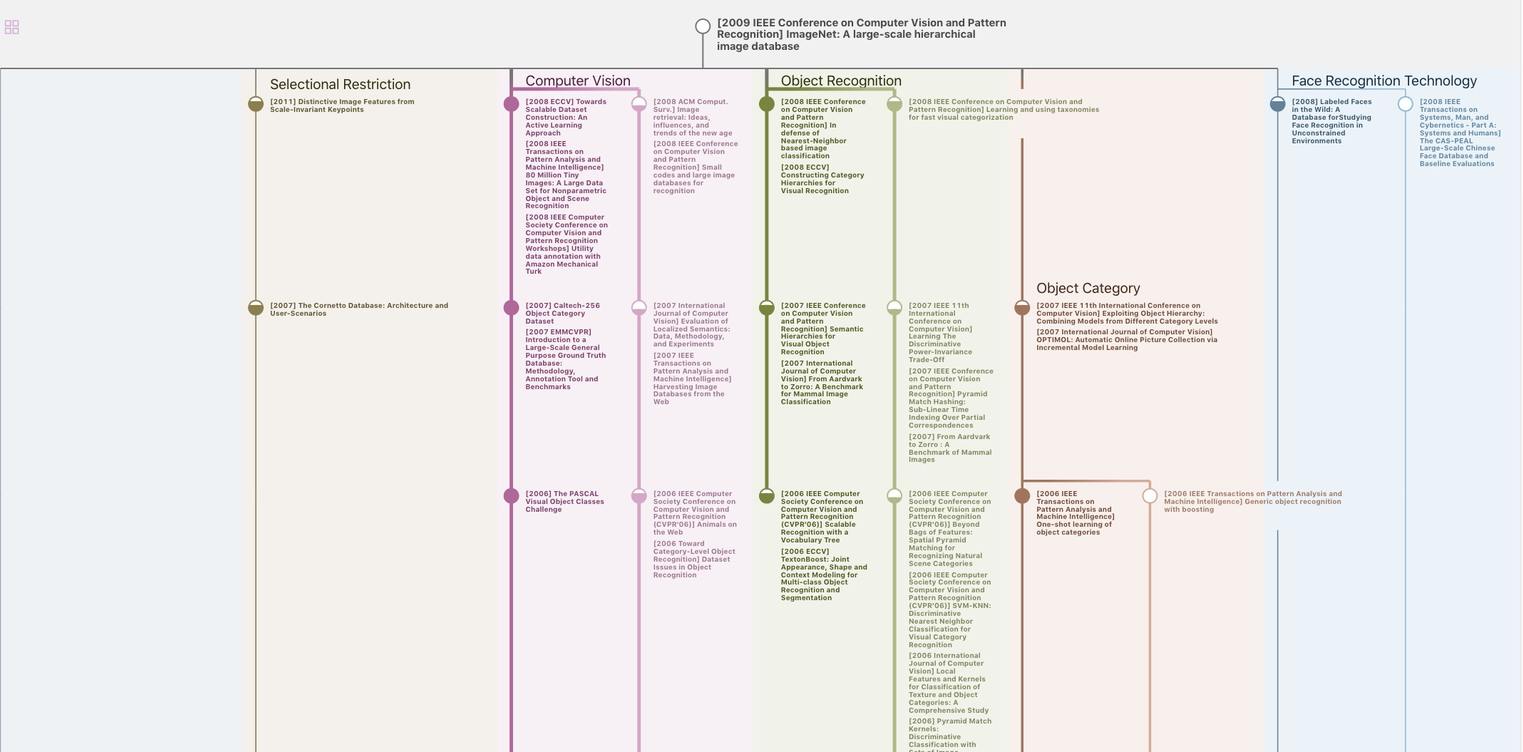
Generate MRT to find the research sequence of this paper
Chat Paper
Summary is being generated by the instructions you defined