Optimal Weak to Strong Learning
NeurIPS 2022(2022)
摘要
The classic algorithm AdaBoost allows to convert a weak learner, that is an algorithm that produces a hypothesis which is slightly better than chance, into a strong learner, achieving arbitrarily high accuracy when given enough training data. We present a new algorithm that constructs a strong learner from a weak learner but uses less training data than AdaBoost and all other weak to strong learners to achieve the same generalization bounds. A sample complexity lower bound shows that our new algorithm uses the minimum possible amount of training data and is thus optimal. Hence, this work settles the sample complexity of the classic problem of constructing a strong learner from a weak learner.
更多查看译文
关键词
boosting,weak learning,sample complexity,lower bound
AI 理解论文
溯源树
样例
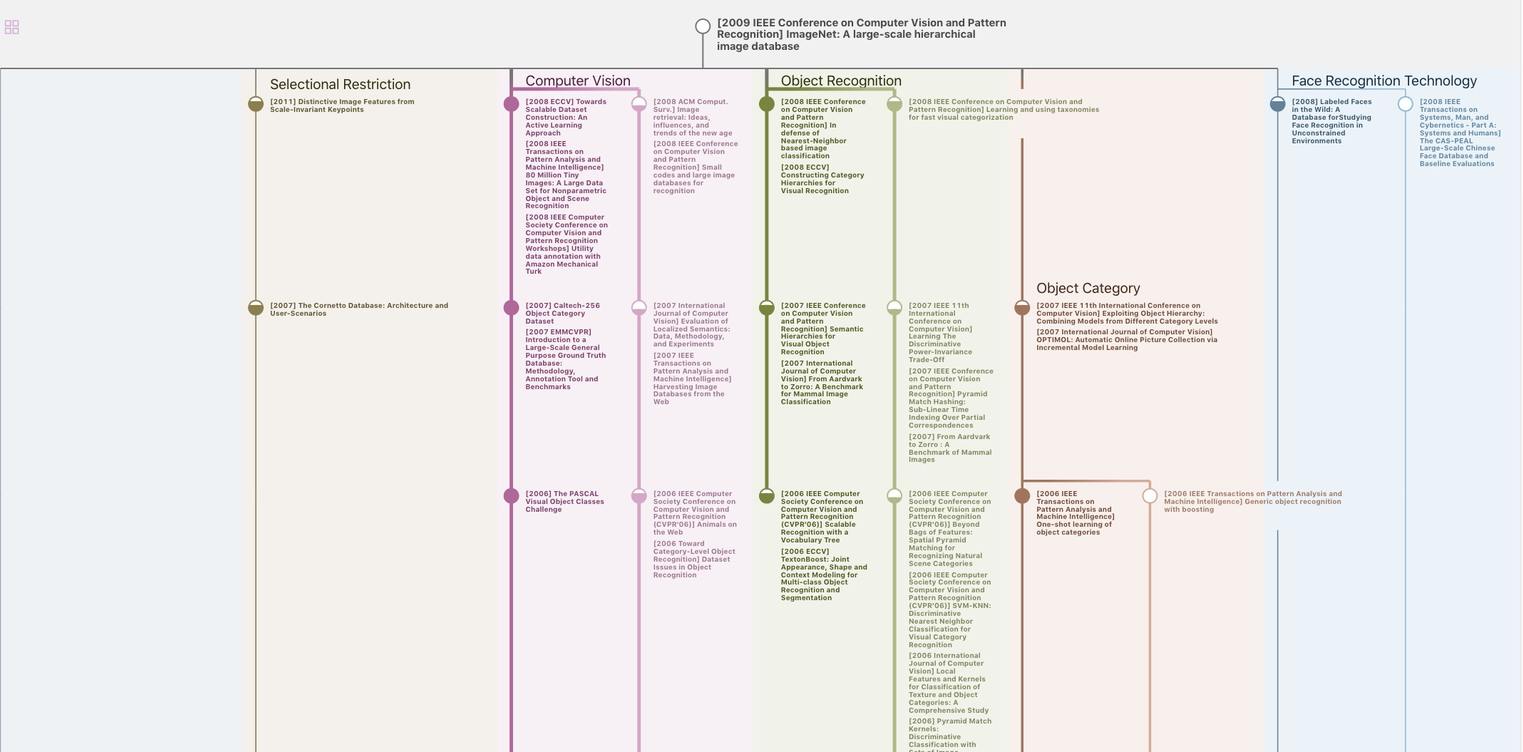
生成溯源树,研究论文发展脉络
Chat Paper
正在生成论文摘要