Compressive Fourier collocation methods for high-dimensional diffusion equations with periodic boundary conditions
IMA JOURNAL OF NUMERICAL ANALYSIS(2024)
摘要
High-dimensional partial differential equations (PDEs) are a popular mathematical modelling tool, with applications ranging from finance to computational chemistry. However, standard numerical techniques for solving these PDEs are typically affected by the curse of dimensionality. In this work, we tackle this challenge while focusing on stationary diffusion equations defined over a high-dimensional domain with periodic boundary conditions. Inspired by recent progress in sparse function approximation in high dimensions, we propose a new method called compressive Fourier collocation. Combining ideas from compressive sensing and spectral collocation, our method replaces the use of structured collocation grids with Monte Carlo sampling and employs sparse recovery techniques, such as orthogonal matching pursuit and $\ell <^>1$ minimization, to approximate the Fourier coefficients of the PDE solution. We conduct a rigorous theoretical analysis showing that the approximation error of the proposed method is comparable with the best $s$-term approximation (with respect to the Fourier basis) to the solution. Using the recently introduced framework of random sampling in bounded Riesz systems, our analysis shows that the compressive Fourier collocation method mitigates the curse of dimensionality with respect to the number of collocation points under sufficient conditions on the regularity of the diffusion coefficient. We also present numerical experiments that illustrate the accuracy and stability of the method for the approximation of sparse and compressible solutions.
更多查看译文
关键词
high-dimensional PDEs,compressive sensing,spectral collocation,bounded Riesz system
AI 理解论文
溯源树
样例
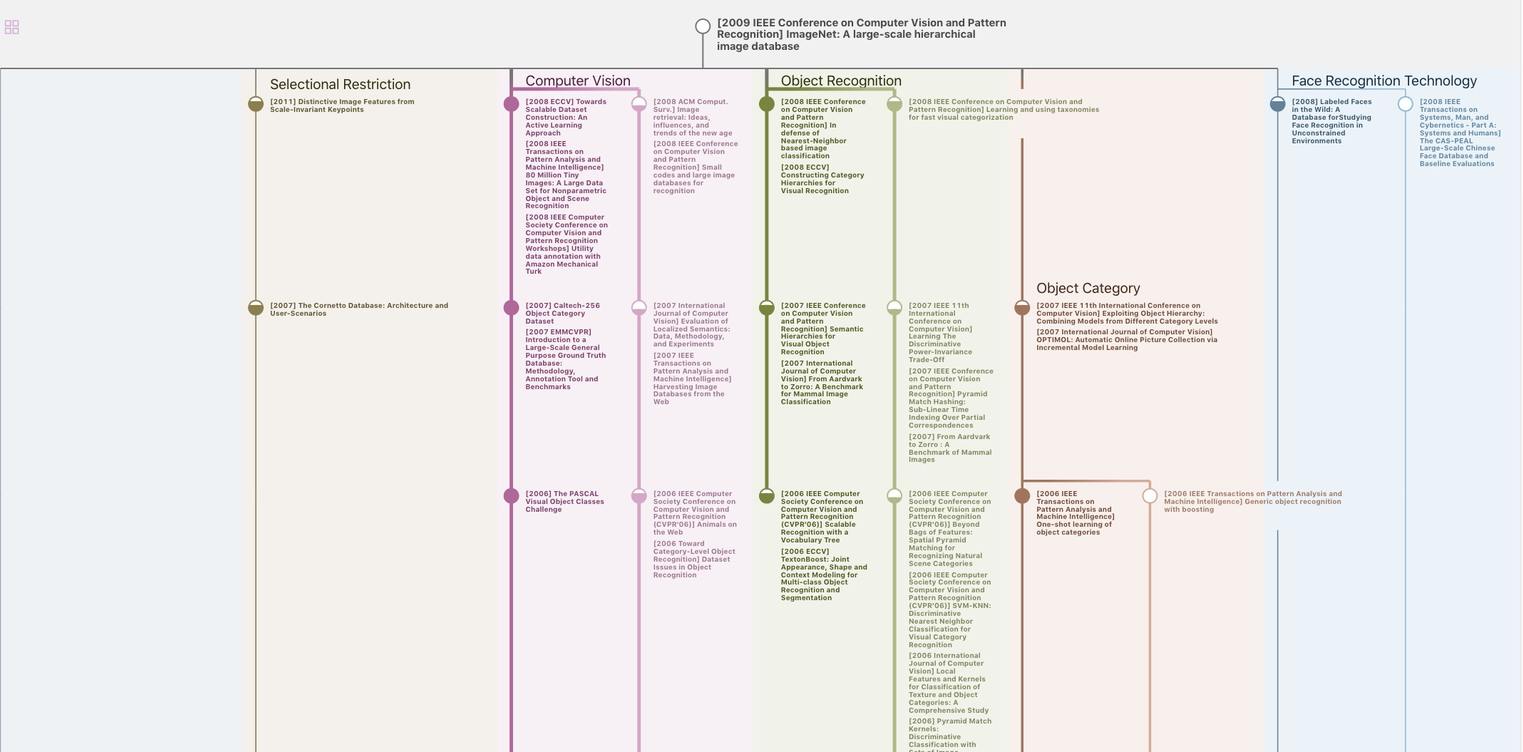
生成溯源树,研究论文发展脉络
Chat Paper
正在生成论文摘要