Beyond “sex prediction”: Estimating and interpreting multivariate sex differences and similarities in the brain
NeuroImage(2022)
Abstract
Previous studies have shown that machine-learning (ML) algorithms can “predict” sex based on brain anatomical/ functional features. The high classification accuracy achieved by ML algorithms is often interpreted as revealing large differences between the brains of males and females and as confirming the existence of “male/female brains”. However, classification and estimation are different concepts, and using classification metrics as surrogate estimates of between-group differences may result in major statistical and interpretative distortions. The present study avoids these distortions and provides a novel and detailed assessment of multivariate sex differences in gray matter volume (GMVOL) that does not rely on classification metrics. Moreover, appropriate regression methods were used to identify the brain areas that contribute the most to these multivariate differences, and clustering techniques and analyses of similarities (ANOSIM) were employed to empirically assess whether they assemble into two sex-typical profiles. Results revealed that multivariate sex differences in GMVOL: (1) are “large” if not adjusted for total intracranial volume (TIV) variation, but “small” when controlling for this variable; (2) differ in size between individuals and also depends on the ML algorithm used for their calculation (3) do not stem from two sex-typical profiles, and so describing them in terms of “male/female brains” is misleading.
MoreTranslated text
Key words
Sex differences,Sex similarities,MRI,Machine learning,Effect size,Gray matter,TIV-adjustment,Robust statistics
AI Read Science
Must-Reading Tree
Example
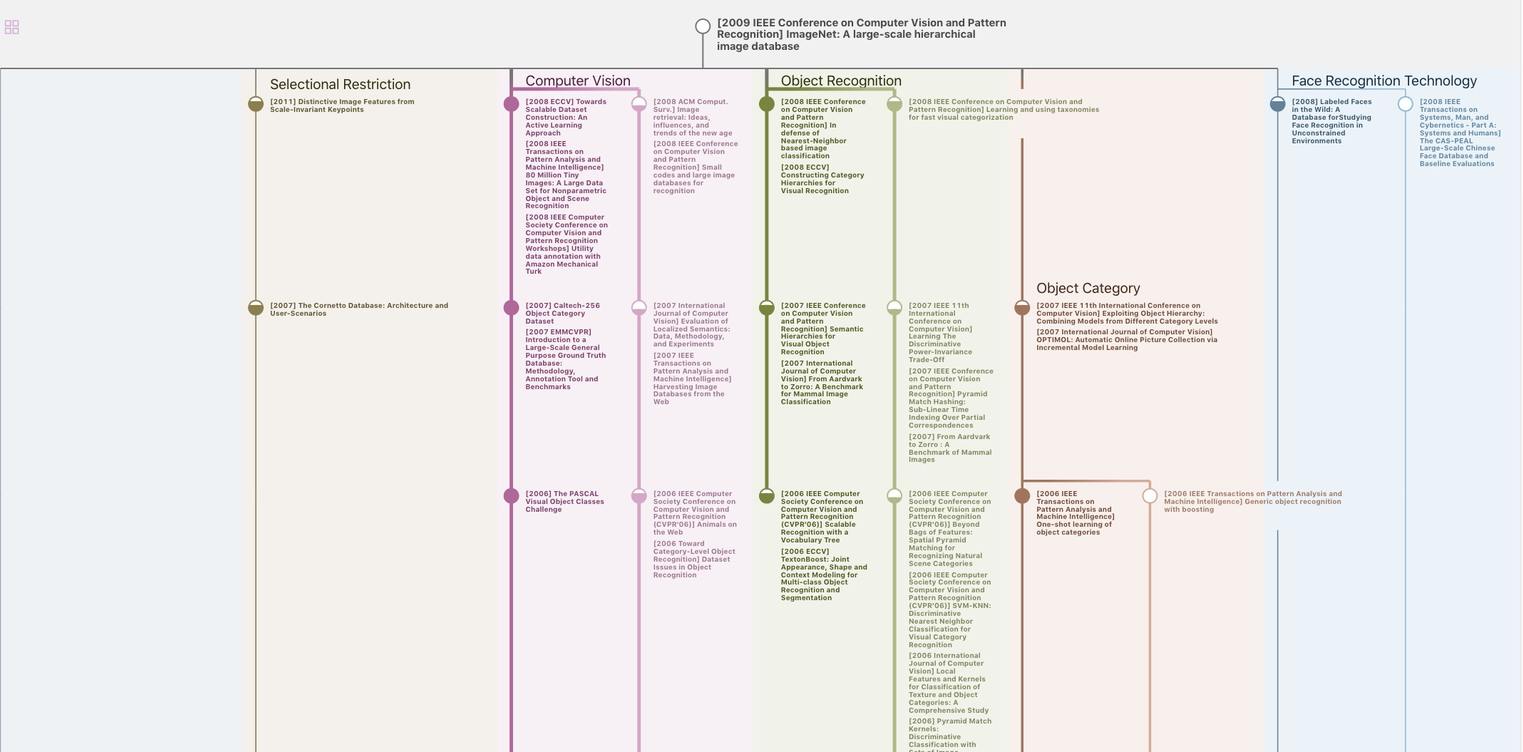
Generate MRT to find the research sequence of this paper
Chat Paper
Summary is being generated by the instructions you defined