Dual-Graph Global and Local Concept Factorization for Data Clustering
IEEE TRANSACTIONS ON NEURAL NETWORKS AND LEARNING SYSTEMS(2024)
摘要
Considering a wide range of applications of nonnegative matrix factorization (NMF), many NMF and their variants have been developed. Since previous NMF methods cannot fully describe complex inner global and local manifold structures of the data space and extract complex structural information, we propose a novel NMF method called dual-graph global and local concept factorization (DGLCF). To properly describe the inner manifold structure, DGLCF introduces the global and local structures of the data manifold and the geometric structure of the feature manifold into CF. The global manifold structure makes the model more discriminative, while the two local regularization terms simultaneously preserve the inherent geometry of data and features. Finally, we analyze convergence and the iterative update rules of DGLCF. We illustrate clustering performance by comparing it with latest algorithms on four real-world datasets.
更多查看译文
关键词
Manifolds,Linear programming,Convergence,Clustering algorithms,Data models,Principal component analysis,Learning systems,Concept factorization (CF),global regularization,image clustering,local regularization
AI 理解论文
溯源树
样例
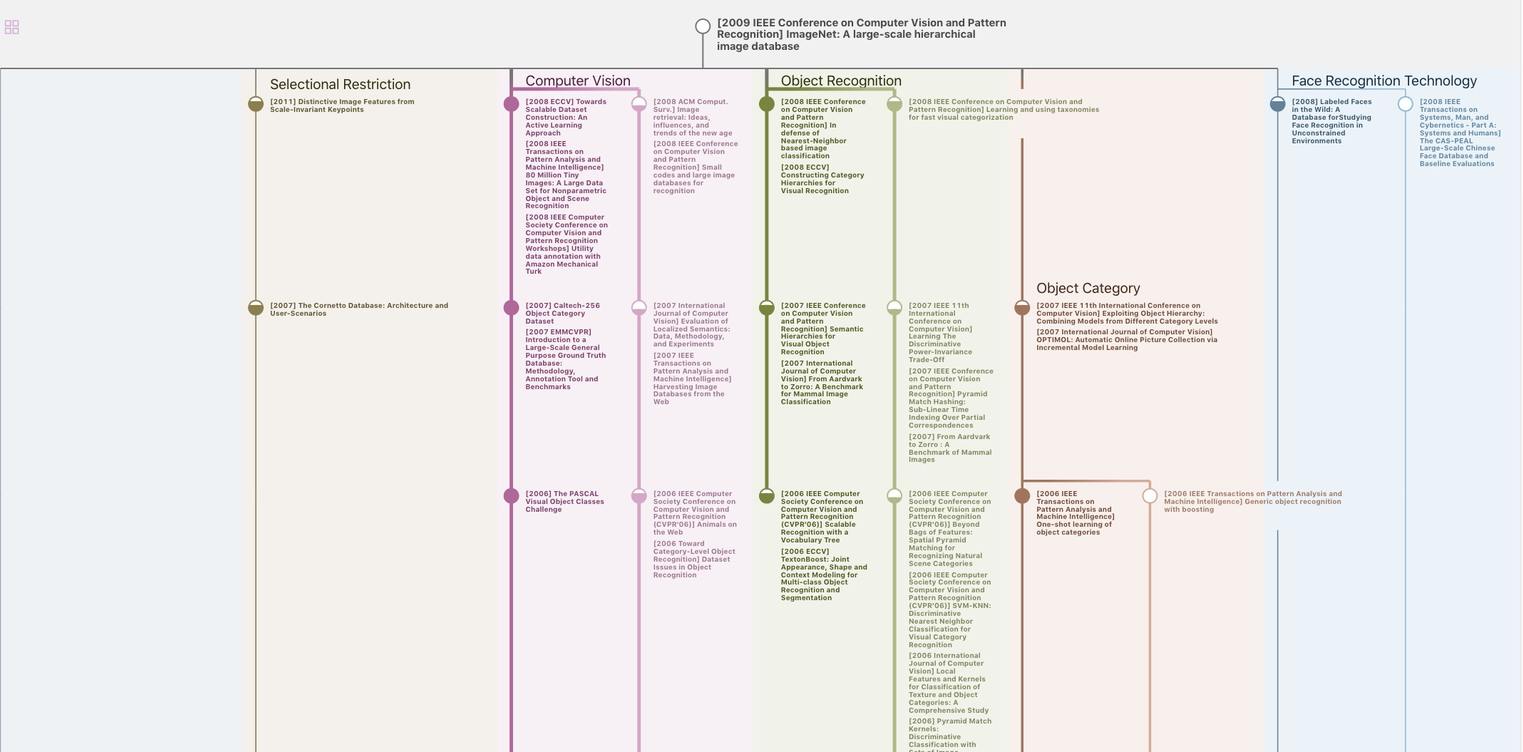
生成溯源树,研究论文发展脉络
Chat Paper
正在生成论文摘要