OmicSelector: automatic feature selection and deep learning modeling for omic experiments
biorxiv(2022)
摘要
A crucial phase of modern biomarker discovery studies is selecting the most promising features from high-throughput screening assays. Here, we present the OmicSelector - Docker-based web application and R package that facilitates the analysis of such experiments. OmicSelector provides a consistent and overfitting-resilient pipeline that integrates 94 feature selection approaches based on 25 distinct variable selection methods. It identifies and then ranks the best feature sets using 11 modeling techniques with hyperparameter optimization in hold-out or cross-validation. OmicSelector provides classification performance metrics for proposed feature sets, allowing researchers to choose the overfitting-resistant biomarker set with the highest diagnostic potential. Finally, it performs GPU-accelerated development, validation, and implementation of deep learning feedforward neural networks (up to 3 hidden layers, with or without autoencoders) on selected signatures. The application performs an extensive grid search of hyperparameters, including balancing and preprocessing of next-generation sequencing (e.g. RNA-seq, miRNA-seq) oraz qPCR data. The pipeline is applicable for determining candidate circulating or tissue miRNAs, gene expression data and methylomic, metabolomic or proteomic analyses. As a case study, we use OmicSelector to develop a diagnostic test for pancreatic and biliary tract cancer based on serum small RNA next-generation sequencing (miRNA-seq) data. The tool is open-source and available at
### Competing Interest Statement
The authors have declared no competing interest.
* ### List of Abbreviations
BTCa
: biliary tract cancer
CPU
: central processing unit
GEO
: Gene Expression Omnibus
GPU
: graphics processing unit
GUI
: graphical user interface
miRNA
: microRNA
miRNA-seq
: small RNA sequencing
PCa
: pancreatic cancer
RELU
: Rectified Linear Units
RNA
: Ribonucleic acid
RNA-seq
: RNA sequencing
ROC
: receiver operating curve
ROSE
: Random Over-Sampling Examples
SELU
: Scaled Exponential Linear Units
SMOTE
: Synthetic Minority Oversampling Technique
更多查看译文
关键词
omicselector,automatic feature selection,deep learning modeling,deep learning
AI 理解论文
溯源树
样例
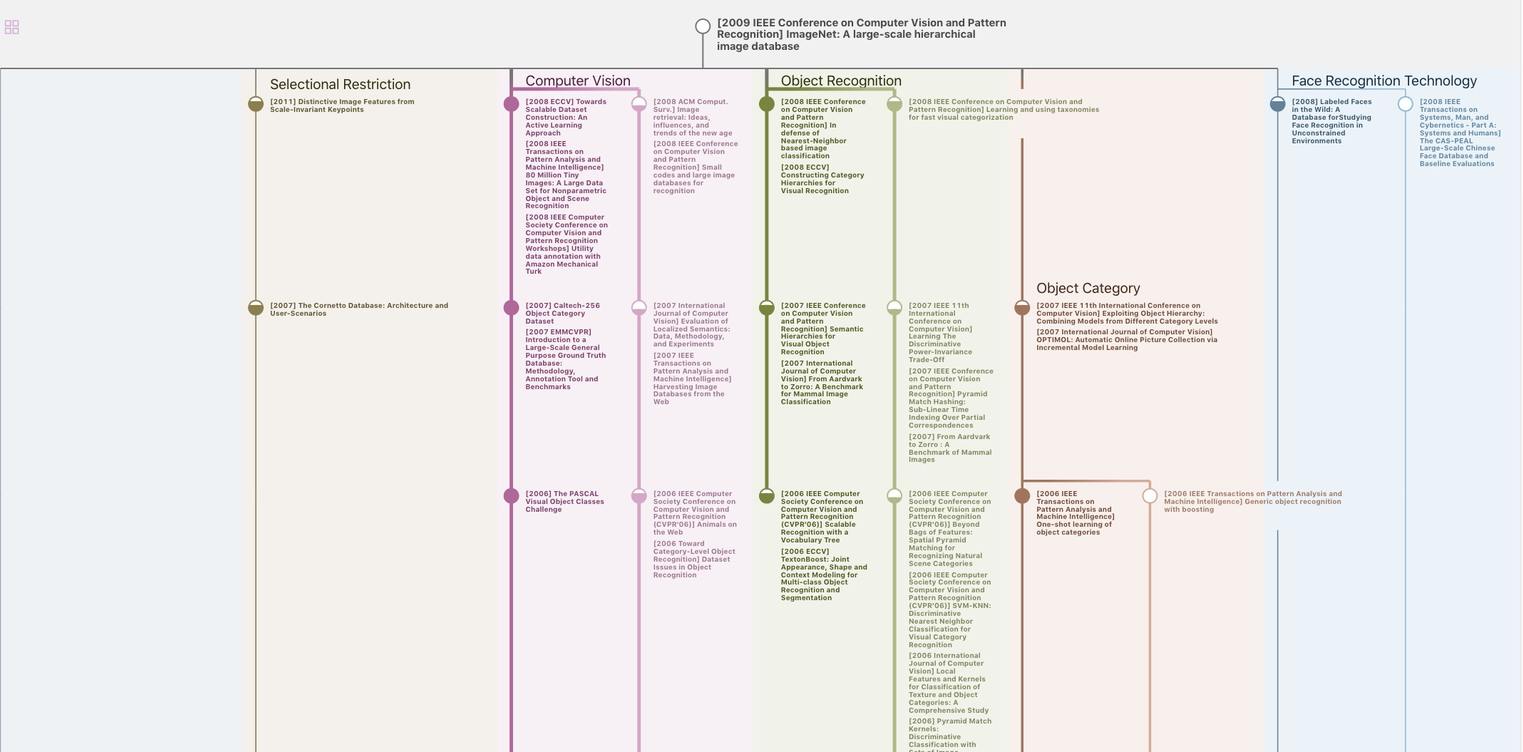
生成溯源树,研究论文发展脉络
Chat Paper
正在生成论文摘要