MuSiC2: cell type deconvolution for multi-condition bulk RNA-seq data
Briefings in Bioinformatics(2022)
Abstract
Cell type composition of intact bulk tissues can vary across samples. Deciphering cell type composition and its changes during disease progression is an important step towards understanding disease pathogenesis. To infer cell type composition, existing cell type deconvolution methods for bulk RNA-seq data often require matched single-cell RNA-seq (scRNA-seq) data, generated from samples with similar clinical conditions, as reference. However, due to the difficulty of obtaining scRNA-seq data in diseased samples, only limited scRNA-seq data in matched disease conditions are available. Using scRNA-seq reference to deconvolve bulk RNA-seq data from samples with different disease conditions may lead to biased estimation of cell type proportions. To overcome this limitation, we propose an iterative estimation procedure, MuSiC2, which is an extension of MuSiC [[1][1]], to perform deconvolution analysis of bulk RNA-seq data generated from samples with multiple clinical conditions where at least one condition is different from that of the scRNA-seq reference. Extensive benchmark evaluations indicated that MuSiC2 improved the accuracy of cell type proportion estimates of bulk RNA-seq samples under different conditions as compared to the traditional MuSiC [[1][1]] deconvolution. MuSiC2 was applied to two bulk RNA-seq datasets for deconvolution analysis, including one from human pancreatic islets and the other from human retina. We show that MuSiC2 improves current deconvolution methods and provides more accurate cell type proportion estimates when the bulk and single-cell reference differ in clinical conditions. We believe the condition-specific cell type composition estimates from MuSiC2 will facilitate downstream analysis and help identify cellular targets of human diseases.
### Competing Interest Statement
The authors have declared no competing interest.
[1]: #ref-1
MoreTranslated text
AI Read Science
Must-Reading Tree
Example
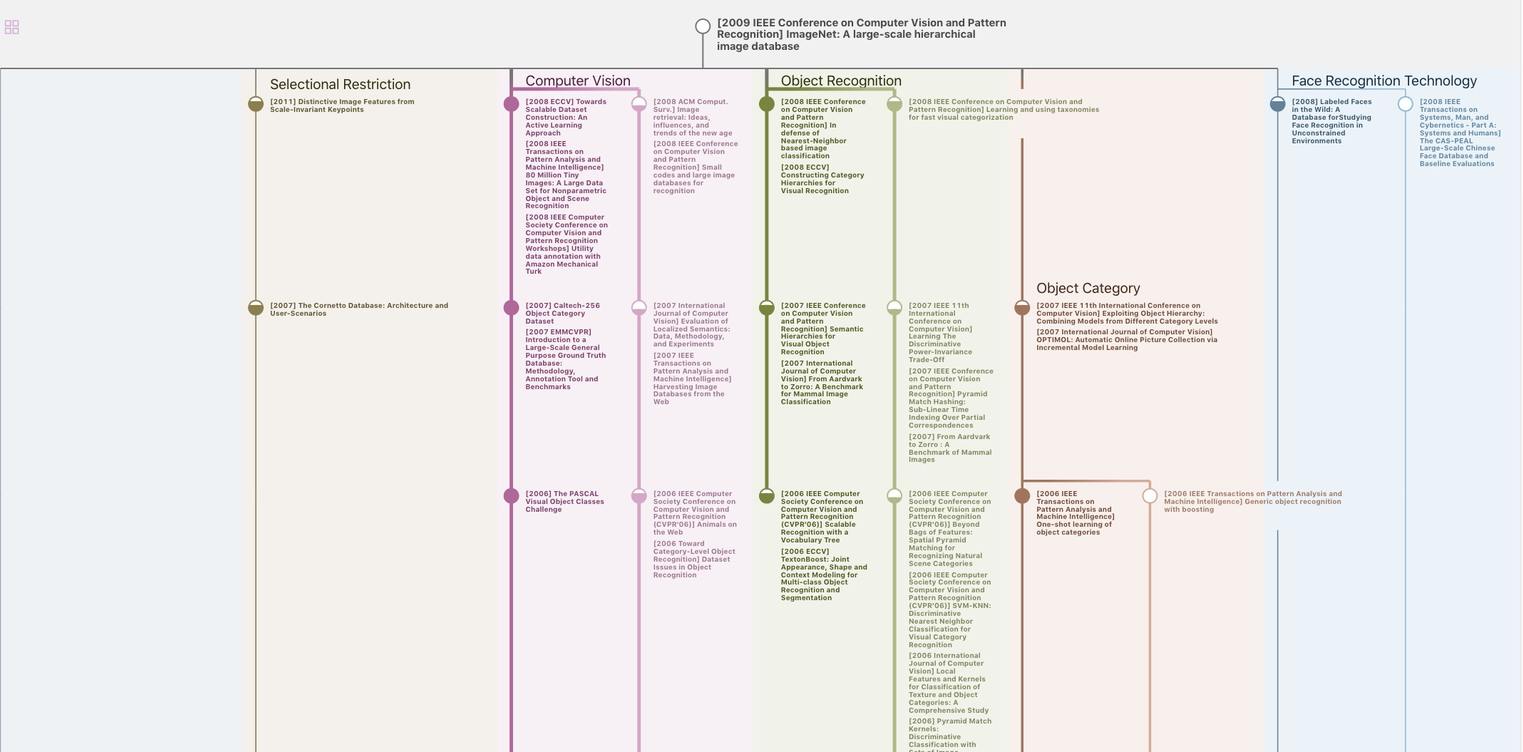
Generate MRT to find the research sequence of this paper
Chat Paper
Summary is being generated by the instructions you defined