Predicting Physical Object Properties from Video
2022 International Joint Conference on Neural Networks (IJCNN)(2022)
摘要
We present a novel approach to estimating physical properties of objects from video. Our approach consists of a physics engine and a correction estimator. Starting from the initial observed state, object behavior is simulated forward in time. Based on the simulated and observed behavior, the correction estimator then determines refined physical parameters for each object. The method can be iterated for increased precision. Our approach is generic, as it allows for the use of an arbitrary-not necessarily differentiable-physics engine and correction estimator. For the latter, we evaluate both gradient-free hyperparameter optimization and a deep convolutional neural network. We demonstrate faster and more robust convergence of the learned method in several simulated 2D scenarios focusing on bin situations.
更多查看译文
关键词
System identification,physics simulation,physical parameters,iterative refinement
AI 理解论文
溯源树
样例
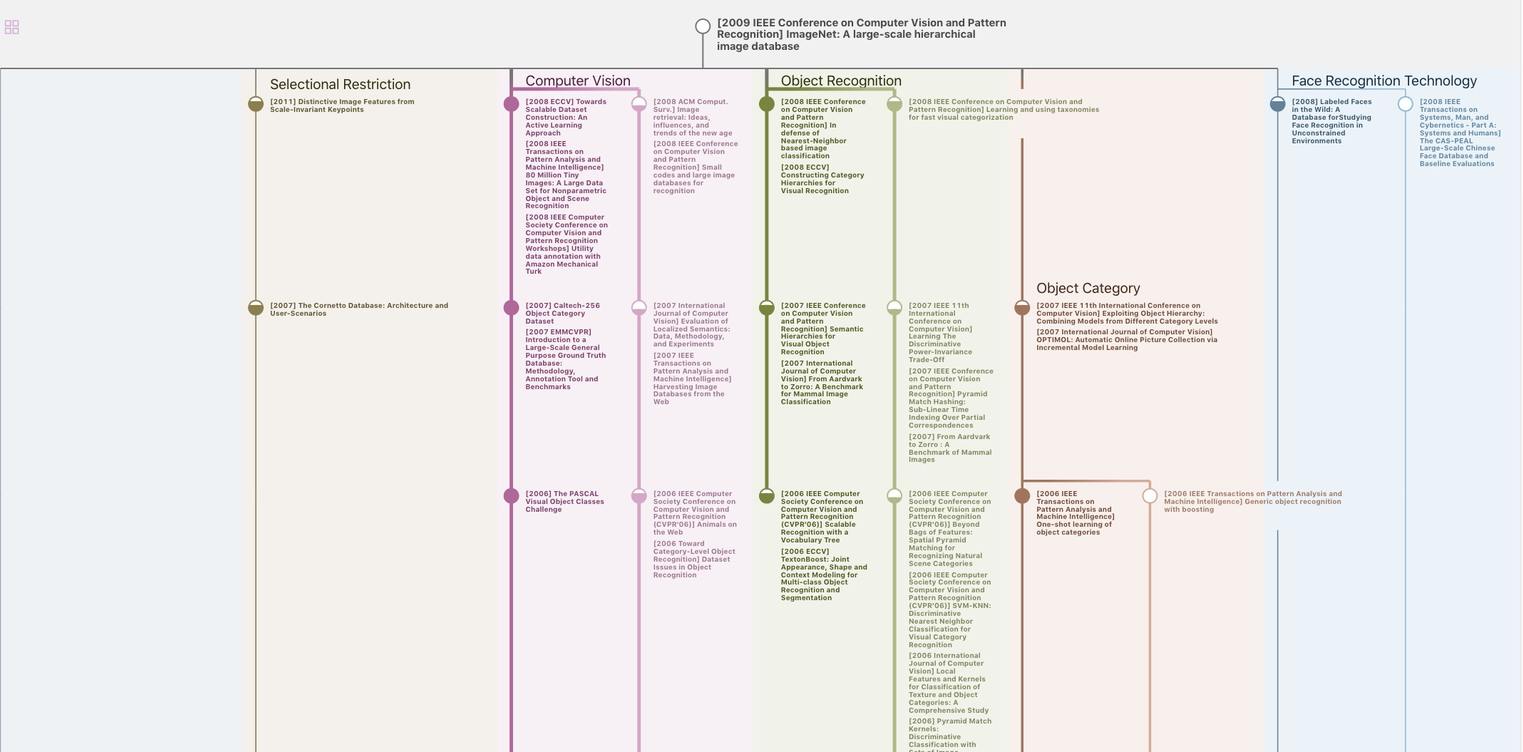
生成溯源树,研究论文发展脉络
Chat Paper
正在生成论文摘要