Reinforcement-learning-based parameter adaptation method for particle swarm optimization
arxiv(2023)
摘要
Particle swarm optimization (PSO) is a well-known optimization algorithm that shows good performances in solving different optimization problems. However, the PSO usually suffers from slow convergence. In this article, a reinforcement-learning-based parameter adaptation method (RLAM) is developed to enhance the PSO convergence by designing a network to control the coefficients of the PSO. Moreover, based on the RLAM, a new reinforcement-learning-based PSO (RLPSO) algorithm is designed. To investigate the performance of the RLAM and RLPSO, experiments on 28 CEC 2013 benchmark functions were carried out to compare with other adaptation methods and PSO variants. The reported computational results showed that the proposed RLAM is efficient and effective and that the proposed RLPSO is superior to several state-of-the-art PSO variants.
更多查看译文
关键词
Particle swarm optimization,Reinforcement learning,CEC 2013 benchmark
AI 理解论文
溯源树
样例
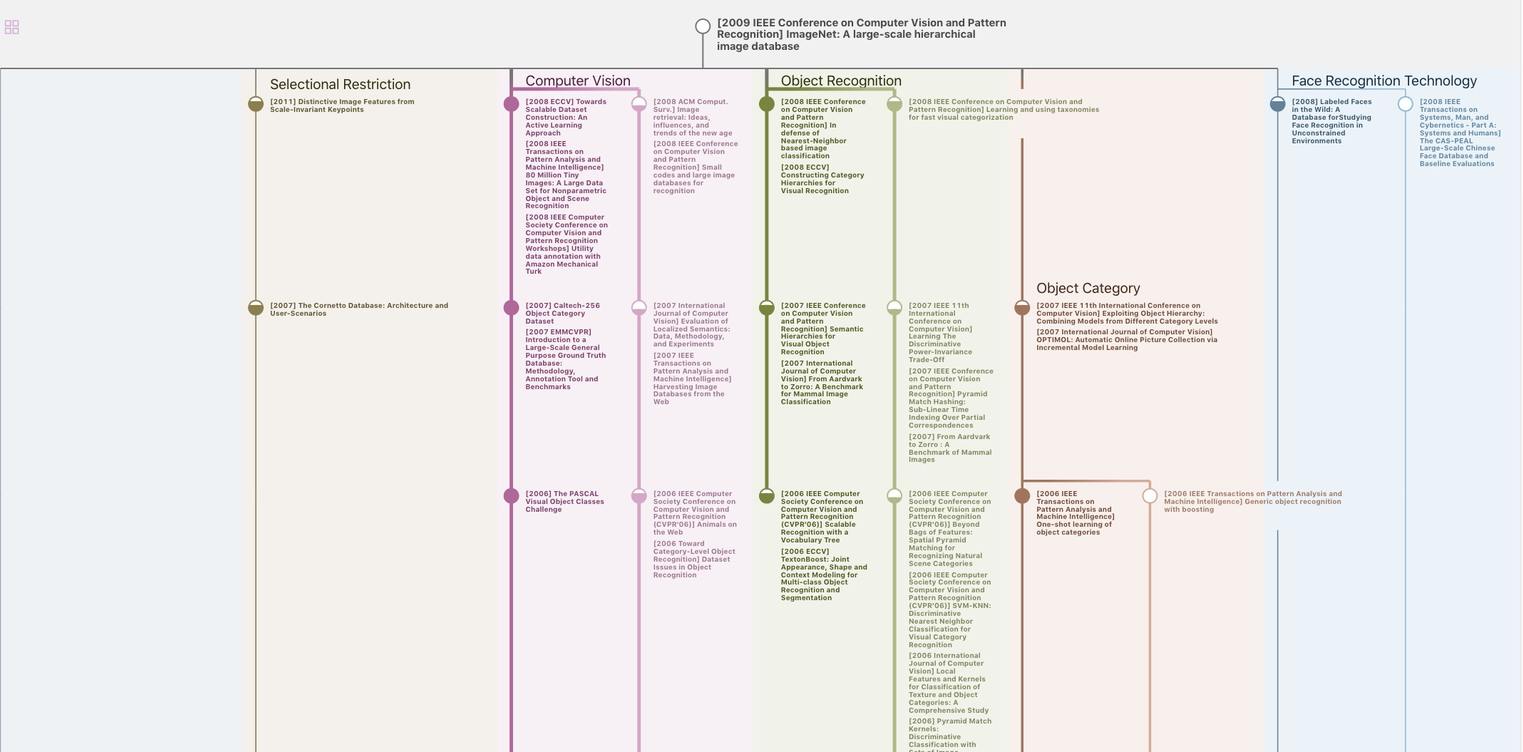
生成溯源树,研究论文发展脉络
Chat Paper
正在生成论文摘要