An Adaptive Sampling Sequential Quadratic Programming Method for Equality Constrained Stochastic Optimization
arXiv (Cornell University)(2023)
Abstract
This paper presents a methodology for using varying sample sizes in sequential quadratic programming (SQP) methods for solving equality constrained stochastic optimization problems. The first part of the paper deals with the delicate issue of dynamic sample selection in the evaluation of the gradient in conjunction with inexact solutions to the SQP subproblems. Under reasonable assumptions on the quality of the employed gradient approximations and the accuracy of the solutions to the SQP subproblems, we establish global convergence results for the proposed method. Motivated by these results, the second part of the paper describes a practical adaptive inexact stochastic sequential quadratic programming (PAIS-SQP) method. We propose criteria for controlling the sample size and the accuracy in the solutions of the SQP subproblems based on estimates of the variance in the stochastic gradient approximations obtained as the optimization progresses. Finally, we demonstrate the performance of the practical method on a subset of the CUTE problems and constrained classification tasks.
MoreTranslated text
Key words
equality constrained stochastic optimization
AI Read Science
Must-Reading Tree
Example
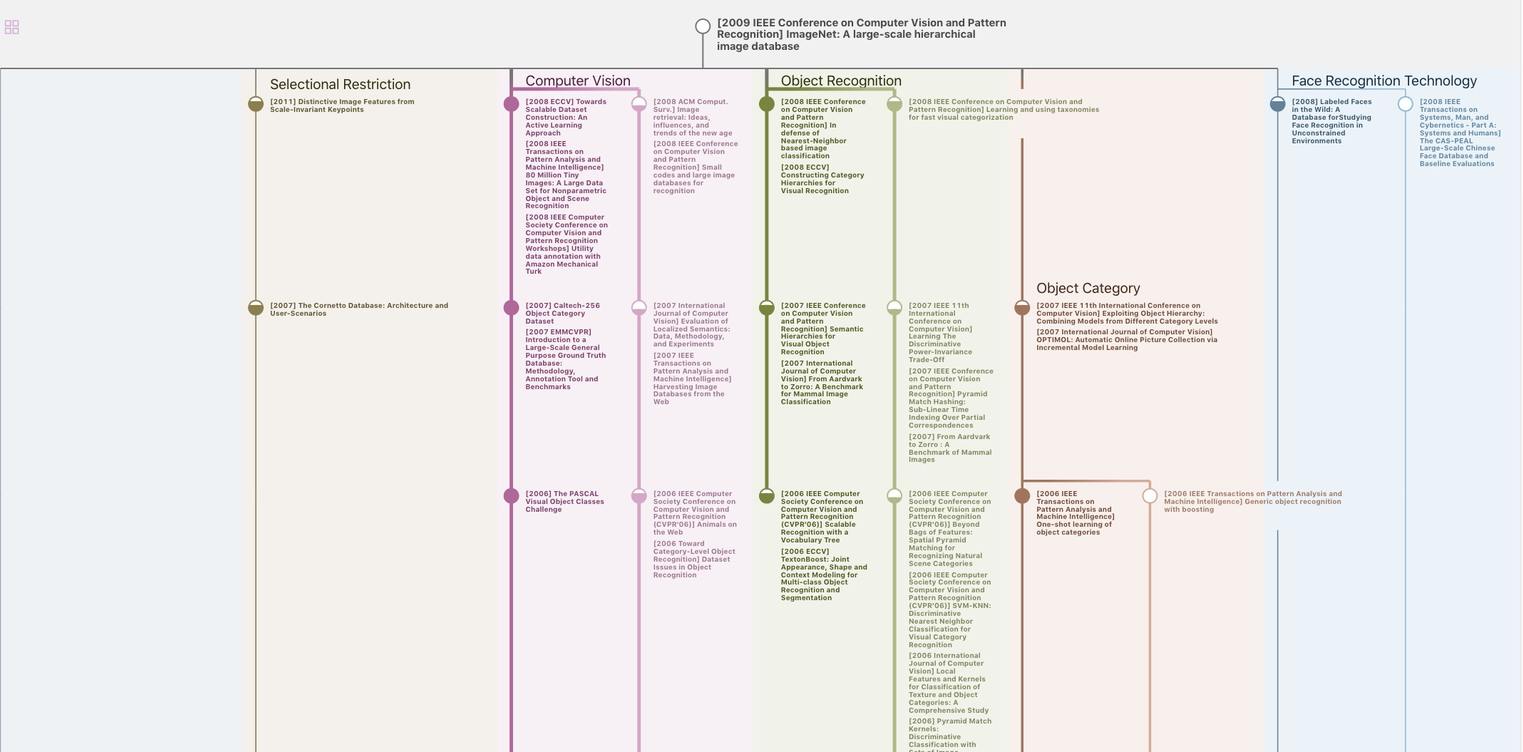
Generate MRT to find the research sequence of this paper
Chat Paper
Summary is being generated by the instructions you defined